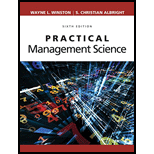
Practical Management Science
6th Edition
ISBN: 9781337406659
Author: WINSTON, Wayne L.
Publisher: Cengage,
expand_more
expand_more
format_list_bulleted
Concept explainers
Textbook Question
Chapter 14.2, Problem 1P
The file P14_01.xlsx contains data on 100 consumers who drink beer. Some of them prefer light beer, and others prefer regular beer. A major beer producer believes that the following variables might be useful in discriminating between these two groups: gender, marital status, annual income level, and age.
- a. Use logistic regression to classify the consumers on the basis of these explanatory variables. How successful is it? Which variables appear to be most important in the classification?
- b. Consider a new customer: Male, Married, Income $42,000, Age 47. Use the logistic regression equation to estimate the probability that this customer prefers Regular. How would you classify this person?
Expert Solution & Answer

Trending nowThis is a popular solution!

Students have asked these similar questions
IM.54 A growing online retailer sells thousands of items yet has has one specialty product category with just 30 items. They want to classify these thirty items according to the annual dollar volume (revenue) generated by each item. The table below lists each item number, Annual Sales (in units), and Price per unit:
Item #
Annual Sales
Price
1
221
$25.85
2
446
$14.15
3
8,940
$168.19
4
2,965
$15.99
5
1,322
$29.37
6
2,575
$12.77
7
767
$28.43
8
5,803
$163.01
9
2,673
$20.51
10
642
$14.71
11
4,168
$54.53
12
1,881
$22.55
13
2,417
$29.63
14
5,207
$36.41
15
1,381
$20.55
16
9,635
$173.69
17
17,406
$27.07
18
1,573
$25.99
19
6,471
$64.99
20
6,047
$29.83
21
433
$20.89
22
2,279
$53.59
23
15,532
$106.91
24
1,585
$4.09
25
5,376
$65.23
26
2,965
$37.93
27
2,048
$28.51
28
1,174
$22.99
29
381
$5.57
30
2,930
$3.43
Which item in the table above has the highest annual dollar volume? In the answer field below, write the ITEM # that…
IM.84 An outdoor equipment manufacturer sells a rugged water bottle to complement its product line. They sell this item to a variety of sporting goods stores and other retailers. The manufacturer offers quantity discounts per the following discount schedule:
Option Plan
Quantity
Price
A
1 - 2,199
$5.00
B
2,200 - 3,699
$4.55
C
3,700+
$3.90
A large big-box retailer expects to sell 9,200 units this year. This retailer estimates that it incurs an internal administrative cost of $235 each time it places an order with the manufacturer. Holding cost for the retailer is $60 per case per year. (There are 52 units or water bottles per case.)Based on this information, and not taking into account any quantity discount offers, what is the calculated EOQ (in units)? (Display your answer to the nearest whole number.)
Based on this information, sort each quantity discount plan from left to right by dragging the MOST preferred option plan to the left, and the LEAST preferred option…
Please provide detailed solutions to the following problems/exercises (4 problems/exercises x 9 points each):
1) View the video ON Unveils ‘Lightspray’ Technology (4.55 mins, Ctrl+Click in the link), and The Secret of Lightspray (8.27 mins, Ctrl+Click in the link), answer the following questions: https://www.youtube.com/watch?v=wjmeaC-wlZs
a) What is new about the design of ON’s shoes?
b) How will ON’s new manufacturing technique affect location planning for footwear firms?
c) How does ON focus on it sustainability strategy?
Note: As a rough guideline, please try to keep the written submission to one or two paragraphs for each of the questions.
2) Unimed Hospital currently processes patient admissions through three admitting clerks who are set up to work in series, with respective reliabilities of 0.96, 0.95, and 0.90 (see figure below).
a) Find the reliability of the current admission process.
Due to rising patient complaints, the hospital administrator, Chimeg…
Knowledge Booster
Learn more about
Need a deep-dive on the concept behind this application? Look no further. Learn more about this topic, operations-management and related others by exploring similar questions and additional content below.Similar questions
- 3) A startup firm, Pocket International, has come up with a tiny programmable computer, NerdCom Mini Air, that sells for $49.99. The firm estimates that the programmable computers have an expected life that is exponential, with a mean of 24 months. The firm wants to estimate the probability that the NerdCom Mini Air will have a life that ends: a) after the initial 24 months of service. b) before the 24 months of service is completed. c) not before 48 months of service. Note: You could work out the problem by hand or use excel; in chapter 4S, section 4S.2 of the Stevenson text, reliability (finding the probability of functioning for a specified length of time) is covered with examples; chapters 4 & 4S Stevenson lecture power point slides 33 to 38 (chapters 4 & 4S lecture: 32.30 mins to 38.56 mins) cover reliability over time with examples.arrow_forwardDiscuss how training and development programs can be best presented to ultimately change the behavior of employees, often without their knowledge or awareness.arrow_forwardDoctor Earrow_forward
- Considering contemporary challenges in business, analyze a real-world case where a company successfully navigated a major shift in its marketing strategy to adapt to changing market dynamics. Discussion Question and Prompt: Identify the key factors that contributed to the success of their marketing strategy in the face of contemporary issues. How can businesses draw insights from this case to inform their own marketing strategies amid current business challenges?arrow_forward1) View the video Service Processing at BuyCostumes (10.41 minutes, Ctrl+Click on the link); what are your key takeaways (tie to one or more of the topics discussed in Chapter 3) after watching this video. (viddler.com/embed/a6b7054c) Note: As a rough guideline, please try to keep the written submission to one or two paragraphs. 2) Orkhon Foods makes hand-held pies (among other products). The firm’s weekly sales of hand-held pies over the past seven weeks are given in the table. The firm’s operations manager, Amarjargal, wants to forecast sales for week 8. Weeks Sales of hand-held pies(000s) 1 19 2 18 3 17 4 20 5 18 6 22 7 20 Forecast the week 8 sales using the following approaches: a) Naïve approach b) 5-month moving average c) 3-month weighted moving average using the following weights: 0.50 for week 7, 0.30 for week 6, and 0.20 for week 5. d) Exponential smoothing using a smoothing constant of 0.30, assume a…arrow_forwardAnswer all parts to question 4 and show all workingarrow_forward
- 1) View the video Service Processing at BuyCostumes (10.41 minutes, Ctrl+Click on the link); what are your key takeaways (tie to one or more of the topics discussed in Chapter 3) after watching this video. (viddler.com/embed/a6b7054c) Note: As a rough guideline, please try to keep the written submission to one or two paragraphs. 2) Orkhon Foods makes hand-held pies (among other products). The firm’s weekly sales of hand-held pies over the past seven weeks are given in the table. The firm’s operations manager, Amarjargal, wants to forecast sales for week 8. Weeks Sales of hand-held pies(000s) 1 19 2 18 3 17 4 20 5 18 6 22 7 20 Forecast the week 8 sales using the following approaches: a) Naïve approach b) 5-month moving average c) 3-month weighted moving average using the following weights: 0.50 for week 7, 0.30 for week 6, and 0.20 for week 5. d) Exponential smoothing using a smoothing constant of 0.30, assume a week 2…arrow_forwardWhat area of emotional intelligence refers to the ability to manage your emotions, particularly in stressful situations, and maintain a positive outlook despite setbacks? relationship management self awareness social awareness self managementarrow_forwardWhat area of emotional intelligence refers to the ability to manage your emotions, particularly in stressful situations, and maintain a positive outlook despite setbacks? relationship management self awareness social awareness self managementarrow_forward
- This area of emotional intelligence describes your ability to not only understand your strengths and weaknesses but to recognize your emotions and their effect on you and your team’s performance self management self awareness relationship management social awarenessarrow_forwardEmotional intelligence is defined as the ability to understand and manage your emotions, as well as recognize and influence the emotions of those around you. True Falsearrow_forwardAt the Ford automobile Highland plant, assume the one-millionth vehicle was produced in 1916 at a cost of $8084 (in 2013 US$), by how much did the Ford company reduce his cost with each doubling of cumulative output from 1916 to 1927?arrow_forward
arrow_back_ios
SEE MORE QUESTIONS
arrow_forward_ios
Recommended textbooks for you
- Practical Management ScienceOperations ManagementISBN:9781337406659Author:WINSTON, Wayne L.Publisher:Cengage,MarketingMarketingISBN:9780357033791Author:Pride, William MPublisher:South Western Educational PublishingContemporary MarketingMarketingISBN:9780357033777Author:Louis E. Boone, David L. KurtzPublisher:Cengage Learning
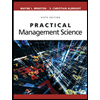
Practical Management Science
Operations Management
ISBN:9781337406659
Author:WINSTON, Wayne L.
Publisher:Cengage,
Marketing
Marketing
ISBN:9780357033791
Author:Pride, William M
Publisher:South Western Educational Publishing
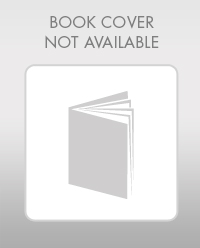
Contemporary Marketing
Marketing
ISBN:9780357033777
Author:Louis E. Boone, David L. Kurtz
Publisher:Cengage Learning
Single Exponential Smoothing & Weighted Moving Average Time Series Forecasting; Author: Matt Macarty;https://www.youtube.com/watch?v=IjETktmL4Kg;License: Standard YouTube License, CC-BY
Introduction to Forecasting - with Examples; Author: Dr. Bharatendra Rai;https://www.youtube.com/watch?v=98K7AG32qv8;License: Standard Youtube License