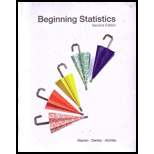
(a)
To calculate:
The sum of squared errors, SSE.

Answer to Problem 9CR
Solution:
The required SSE is 16.7246.
Explanation of Solution
Given Information:
The following data is collected on the number of years of post high-school education and the annual incomes of eight people ten years after graduation from high school.
Thread Count | 150 | 200 | 225 | 250 | 275 | 300 | 350 | 400 |
Price (in Dollars) | 18 | 21 | 25 | 28 | 30 | 31 | 35 | 45 |
The least Squares regression line is the line for which the average variation from the data is the smallest, also called the line of best fit, given by
Where
And
Formula used:
The equation of least-squares regression line is given by,
Where
And
Where n is the number of data pairs in the sample,
And
The sum of squared errors (SSE) for a regression line is calculated as,
Where,
And
Calculation:
Thread Count | Price(in Dollars) | |||
150 | 18 | 2700 | 22500 | 324 |
200 | 21 | 4200 | 40000 | 441 |
225 | 25 | 5625 | 50625 | 625 |
250 | 28 | 7000 | 62500 | 784 |
275 | 30 | 8250 | 75625 | 900 |
300 | 31 | 9300 | 90000 | 961 |
350 | 35 | 12250 | 122500 | 1225 |
400 | 45 | 18000 | 160000 | 2025 |
Let
And
The slope of the least-squares regression line is calculated as,
Where,
Substitute 150 for
Proceed in the same manner to calculate
The slope of the least-squares regression line is calculated as,
Substitute 2150 for
The y-intercept of regression line is calculated as,
Substitute 2150 for
The equation of least-squares regression line is given by,
Substitute 28.6514 for
Number of years |
Annual income |
Predicted value |
||
150 | 18 | 16.965 | 1.035 | 1.071225 |
200 | 21 | 22.085 | -1.085 | 1.177225 |
225 | 25 | 24.645 | 0.355 | 0.126025 |
250 | 28 | 27.205 | 0.795 | 0.632025 |
275 | 30 | 29.765 | 0.235 | 0.055225 |
300 | 31 | 32.325 | -1.325 | 1.755625 |
350 | 35 | 37.445 | -2.445 | 5.978025 |
400 | 45 | 42.565 | 2.435 | 5.929225 |
The predicted values are calculated as,
The predicted value
Substitute 150 for
Proceed in the same manner to calculate
The residual is calculated as,
Substitute 18 for
Square both sides of the equation.
Proceed in the same manner to calculate
Conclusion:
Thus, the SSE is 16.7246
(b)
To calculate:
The standard error of estimate,

Answer to Problem 9CR
Solution:
The required standard error of estimate is
Explanation of Solution
Given Information:
The following data is collected on the number of years of post high-school education and the annual incomes of eight people ten years after graduation from high school.
Thread Count | 150 | 200 | 225 | 250 | 275 | 300 | 350 | 400 |
Price (in Dollars) | 18 | 21 | 25 | 28 | 30 | 31 | 35 | 45 |
Formula used:
The standard error of estimate, which is used to measure by how much the sample data points deviate from regression line is given by,
Where,
n is the number of data pairs in the sample,
And SSE is the sum of squared errors.
Calculation:
The standard error of estimate is calculated as,
Substitute 5868.153 for SSE and 8 for n in the above formula.
Conclusion:
Thus, the standard error of estimate is
(c)
The 95% prediction interval for the price of 350-thread count sheets.

Answer to Problem 9CR
Solution:
The required prediction interval is.
Explanation of Solution
Given Information:
The following data is collected on the number of years of post high-school education and the annual incomes of eight people ten years after graduation from high school.
Thread Count | 150 | 200 | 225 | 250 | 275 | 300 | 350 | 400 |
Price (in Dollars) | 18 | 21 | 25 | 28 | 30 | 31 | 35 | 45 |
Formula used:
The margin of error of a prediction interval for an individual y-value is calculated as,
With degree of freedom
Where,
n is the number of data pairs in the sample,
SSE is the sum of squared errors,
And
Then the prediction interval for an individual y-value is,
Calculation:
It is given that the level of prediction is 0.95 then the level of significance is calculated as,
Then,
The mean of the number of years of post high school education is calculated as,
Substitute 2150 for
The margin of error of a prediction interval for an individual y-value is calculated as,
Substitute 2.447 for
The
The regression line is,
Substitute 350 for
The prediction interval is,
Conclusion:
The required prediction interval is.
(d)
The 95% confidence interval for the y-intercept of the regression line.

Answer to Problem 9CR
Solution:
The required confidence interval is
Explanation of Solution
Given Information:
The following data is collected on the number of years of post high-school education and the annual incomes of eight people ten years after graduation from high school.
Thread Count | 150 | 200 | 225 | 250 | 275 | 300 | 350 | 400 |
Price (in Dollars) | 18 | 21 | 25 | 28 | 30 | 31 | 35 | 45 |
Formula Used:
The
Coefficient of determination measures the proportion of variation in the response variable caused by explanatory variable which is simply the square of r, the correlation coefficient.
The standard error of estimate,
In ANOVA,
Grand Mean is the weighted mean of the
Sum of Squares among Treatments (SST) is the measures the variation between the sample means and the grand mean, given by,
Sum of Squares for Error (SSE) is the measures the variation in the sample data resulting from the variability within each sample,
Total Variation, it is the sum of the squared deviations from the grand mean for all of the data values in each sample, given by
Mean Square for Treatments (MST) found by dividing the sum of squares among treatments by its degrees of freedom, given by
Mean Square for Error (MSE) found by dividing the sum of squares for error by its degrees of freedom, given by
Test Statistic for an ANOVA Test is used when independent, simple random samples are taken from populations with variances that are unknown and assumed to be equal, where all of the
Calculation:
To generate the regression table in excel follow the given steps:
1. Under data tab, choose data analytics and then select regression.
2. Select the input Y range and enter the range of the given
3.Choose 95% confidence interval and click OK.
The following table will appear.
Regression Statistics | |
Multiple R | 0.983094904 |
R Square | 0.96647559 |
Adjusted R Square | 0.960888189 |
Standard Error | 1.66955532 |
Observations | 8 |
ANOVA | |||||
df | SS | MS | F | Significance F | |
Regression | 1 | 482.1505102 | 482.1505102 | 172.9740696 | 1.19253E-05 |
Residual | 6 | 16.7244898 | 2.787414966 | ||
Total | 7 | 498.875 |
Coefficients | Standard Error | t Stat | P-value | Lower 95% | Upper 95% | |
Intercept | 1.591836735 | 2.17509095 | 0.73184835 | 0.491845191 | -3.730419077 | 6.914092547 |
Slope | 0.10244898 | 0.007789635 | 13.15196067 | 1.19253E-05 | 0.083388428 | 0.121509531 |
RESIDUAL OUTPUT | |||
Observation | Predicted y | Residuals | Standard Residuals |
1 | 16.95918367 | 1.040816327 | 0.673359012 |
2 | 22.08163265 | -1.081632653 | -0.699765248 |
3 | 24.64285714 | 0.357142857 | 0.231054563 |
4 | 27.20408163 | 0.795918367 | 0.514921598 |
5 | 29.76530612 | 0.234693878 | 0.151835856 |
6 | 32.32653061 | -1.326530612 | -0.858202663 |
7 | 37.44897959 | -2.448979592 | -1.584374147 |
8 | 42.57142857 | 2.428571429 | 1.571171029 |
The confidence interval of the y-intercept can be constructed by adding and subtracting the margin of error to the point estimate by using Microsoft excel.
Referring regression statistics,
Standard error is the standard error of estimate,
The lower 95% and the upper 95% gives the confidence interval of the y-intercept.
The intercept given in the row of the table above is the
So the regression line is,
The lower and the upper endpoints for a 95% confidence interval for the y-intercept of the regression line,
Conclusion:
Thus, the 95% confidence interval for the y-intercept of the regression line is.
(e)
Construct a 95% confidence interval for the slope of the regression line.

Answer to Problem 9CR
Solution:
The required confidence interval is
Explanation of Solution
Given Information:
The following data is collected on the number of years of post high-school education and the annual incomes of eight people ten years after graduation from high school.
Thread Count | 150 | 200 | 225 | 250 | 275 | 300 | 350 | 400 |
Price (in Dollars) | 18 | 21 | 25 | 28 | 30 | 31 | 35 | 45 |
Formula Used:
The
Coefficient of determination measures the proportion of variation in the response variable caused by explanatory variable which is simply the square of r, the correlation coefficient.
The standard error of estimate,
In ANOVA,
Grand Mean is the weighted mean of the
Sum of Squares among Treatments (SST) is the measures the variation between the sample means and the grand mean, given by,
Sum of Squares for Error (SSE) is the measures the variation in the sample data resulting from the variability within each sample,
Total Variation, it is the sum of the squared deviations from the grand mean for all of the data values in each sample, given by
Mean Square for Treatments (MST) found by dividing the sum of squares among treatments by its degrees of freedom, given by
Mean Square for Error (MSE) found by dividing the sum of squares for error by its degrees of freedom, given by
Test Statistic for an ANOVA Test is used when independent, simple random samples are taken from populations with variances that are unknown and assumed to be equal, where all of the
Calculation:
To generate the regression table in excel follow the given steps:
1. Under data tab, choose data analytics and then select regression.
2. Select the input Y range and enter the range of the given
3.Choose 95% confidence interval and click OK.
The following table will appear.
Regression Statistics | |||||||||||||||||
Multiple R | 0.983094904 | ||||||||||||||||
R Square | 0.96647559 | ||||||||||||||||
Adjusted R Square | 0.960888189 | ||||||||||||||||
Standard Error | 1.66955532 | ||||||||||||||||
Observations | 8 | ||||||||||||||||
ANOVA | |||||||||||||||||
df | SS | MS | F | Significance F | |||||||||||||
Regression | 1 | 482.1505102 | 482.1505102 | 172.9740696 | 1.19253E-05 | ||||||||||||
Residual | 6 | 16.7244898 | 2.787414966 | ||||||||||||||
Total | 7 | 498.875 | |||||||||||||||
Coefficients | Standard Error | t Stat | P-value | Lower 95% | Upper 95% | ||||||||||||
Intercept | 1.591836735 | 2.17509095 | 0.731848356 | 0.491845191 | -3.730419077 | 6.914092547 | |||||||||||
Slope | 0.10244898 | 0.007789635 | 13.15196067 | 1.19253E-05 | 0.083388428 | 0.121509531 | |||||||||||
RESIDUAL OUTPUT | |||||||||||||||||
Observation | Predicted y | Residuals | Standard Residuals | ||||||||||||||
1 | 16.95918367 | 1.040816327 | 0.673359012 | ||||||||||||||
2 | 22.08163265 | -1.081632653 | -0.699765248 | ||||||||||||||
3 | 24.64285714 | 0.357142857 | 0.231054563 | ||||||||||||||
4 | 27.20408163 | 0.795918367 | 0.514921598 | ||||||||||||||
5 | 29.76530612 | 0.234693878 | 0.151835856 | ||||||||||||||
6 | 32.32653061 | -1.326530612 | -0.858202663 | ||||||||||||||
7 | 37.44897959 | -2.448979592 | -1.584374147 | ||||||||||||||
8 | 42.57142857 | 2.428571429 | 1.571171029 | ||||||||||||||
The lower 95% and the upper 95% gives the confidence interval of the slope.
The intercept given in the row of the table above is the
So the regression line is,
The lower and the upper endpoints for a 95% confidence interval for the slope of the regression line,
Conclusion:
Thus, the 95% confidence interval for the slope of the regression line is
Want to see more full solutions like this?
Chapter 12 Solutions
Beginning Statistics, 2nd Edition
- Business discussarrow_forwardBusiness discussarrow_forwardI just need to know why this is wrong below: What is the test statistic W? W=5 (incorrect) and What is the p-value of this test? (p-value < 0.001-- incorrect) Use the Wilcoxon signed rank test to test the hypothesis that the median number of pages in the statistics books in the library from which the sample was taken is 400. A sample of 12 statistics books have the following numbers of pages pages 127 217 486 132 397 297 396 327 292 256 358 272 What is the sum of the negative ranks (W-)? 75 What is the sum of the positive ranks (W+)? 5What type of test is this? two tailedWhat is the test statistic W? 5 These are the critical values for a 1-tailed Wilcoxon Signed Rank test for n=12 Alpha Level 0.001 0.005 0.01 0.025 0.05 0.1 0.2 Critical Value 75 70 68 64 60 56 50 What is the p-value for this test? p-value < 0.001arrow_forward
- ons 12. A sociologist hypothesizes that the crime rate is higher in areas with higher poverty rate and lower median income. She col- lects data on the crime rate (crimes per 100,000 residents), the poverty rate (in %), and the median income (in $1,000s) from 41 New England cities. A portion of the regression results is shown in the following table. Standard Coefficients error t stat p-value Intercept -301.62 549.71 -0.55 0.5864 Poverty 53.16 14.22 3.74 0.0006 Income 4.95 8.26 0.60 0.5526 a. b. Are the signs as expected on the slope coefficients? Predict the crime rate in an area with a poverty rate of 20% and a median income of $50,000. 3. Using data from 50 workarrow_forward2. The owner of several used-car dealerships believes that the selling price of a used car can best be predicted using the car's age. He uses data on the recent selling price (in $) and age of 20 used sedans to estimate Price = Po + B₁Age + ε. A portion of the regression results is shown in the accompanying table. Standard Coefficients Intercept 21187.94 Error 733.42 t Stat p-value 28.89 1.56E-16 Age -1208.25 128.95 -9.37 2.41E-08 a. What is the estimate for B₁? Interpret this value. b. What is the sample regression equation? C. Predict the selling price of a 5-year-old sedan.arrow_forwardian income of $50,000. erty rate of 13. Using data from 50 workers, a researcher estimates Wage = Bo+B,Education + B₂Experience + B3Age+e, where Wage is the hourly wage rate and Education, Experience, and Age are the years of higher education, the years of experience, and the age of the worker, respectively. A portion of the regression results is shown in the following table. ni ogolloo bash 1 Standard Coefficients error t stat p-value Intercept 7.87 4.09 1.93 0.0603 Education 1.44 0.34 4.24 0.0001 Experience 0.45 0.14 3.16 0.0028 Age -0.01 0.08 -0.14 0.8920 a. Interpret the estimated coefficients for Education and Experience. b. Predict the hourly wage rate for a 30-year-old worker with four years of higher education and three years of experience.arrow_forward
- 1. If a firm spends more on advertising, is it likely to increase sales? Data on annual sales (in $100,000s) and advertising expenditures (in $10,000s) were collected for 20 firms in order to estimate the model Sales = Po + B₁Advertising + ε. A portion of the regression results is shown in the accompanying table. Intercept Advertising Standard Coefficients Error t Stat p-value -7.42 1.46 -5.09 7.66E-05 0.42 0.05 8.70 7.26E-08 a. Interpret the estimated slope coefficient. b. What is the sample regression equation? C. Predict the sales for a firm that spends $500,000 annually on advertising.arrow_forwardCan you help me solve problem 38 with steps im stuck.arrow_forwardHow do the samples hold up to the efficiency test? What percentages of the samples pass or fail the test? What would be the likelihood of having the following specific number of efficiency test failures in the next 300 processors tested? 1 failures, 5 failures, 10 failures and 20 failures.arrow_forward
- The battery temperatures are a major concern for us. Can you analyze and describe the sample data? What are the average and median temperatures? How much variability is there in the temperatures? Is there anything that stands out? Our engineers’ assumption is that the temperature data is normally distributed. If that is the case, what would be the likelihood that the Safety Zone temperature will exceed 5.15 degrees? What is the probability that the Safety Zone temperature will be less than 4.65 degrees? What is the actual percentage of samples that exceed 5.25 degrees or are less than 4.75 degrees? Is the manufacturing process producing units with stable Safety Zone temperatures? Can you check if there are any apparent changes in the temperature pattern? Are there any outliers? A closer look at the Z-scores should help you in this regard.arrow_forwardNeed help pleasearrow_forwardPlease conduct a step by step of these statistical tests on separate sheets of Microsoft Excel. If the calculations in Microsoft Excel are incorrect, the null and alternative hypotheses, as well as the conclusions drawn from them, will be meaningless and will not receive any points. 4. One-Way ANOVA: Analyze the customer satisfaction scores across four different product categories to determine if there is a significant difference in means. (Hints: The null can be about maintaining status-quo or no difference among groups) H0 = H1=arrow_forward
- MATLAB: An Introduction with ApplicationsStatisticsISBN:9781119256830Author:Amos GilatPublisher:John Wiley & Sons IncProbability and Statistics for Engineering and th...StatisticsISBN:9781305251809Author:Jay L. DevorePublisher:Cengage LearningStatistics for The Behavioral Sciences (MindTap C...StatisticsISBN:9781305504912Author:Frederick J Gravetter, Larry B. WallnauPublisher:Cengage Learning
- Elementary Statistics: Picturing the World (7th E...StatisticsISBN:9780134683416Author:Ron Larson, Betsy FarberPublisher:PEARSONThe Basic Practice of StatisticsStatisticsISBN:9781319042578Author:David S. Moore, William I. Notz, Michael A. FlignerPublisher:W. H. FreemanIntroduction to the Practice of StatisticsStatisticsISBN:9781319013387Author:David S. Moore, George P. McCabe, Bruce A. CraigPublisher:W. H. Freeman
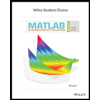
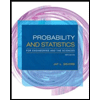
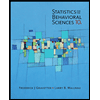
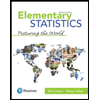
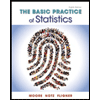
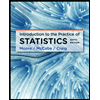