Rose Par posted Apr 20, 2025 11:00 AM Subscribe In a future business administration role, especially in human resources or finance, one type of data commonly collected is employee salary information. This includes salary amounts, job titles, departments, and years of service, typically gathered through internal HR systems or payroll software. It's essential to make reliable estimates from a sample of this data to identify pay trends, allocate budgets, and ensure fair compensation practices without surveying every employee (Whitfield, 2023, par. 1). Since salary data is often skewed due to a few high earners, the Central Limit Theorem (CLT) becomes essential. CLT states that as sample sizes grow larger, the distribution of sample means will approach a normal distribution, regardless of the population's original shape (Whitfield, 2023, par. 3 & 7). This means even if the salary distribution is not normal, taking a large enough sample, typically 30 or more employees, allows us to make valid inferences about the average salary (Whitfield, 2023, par. 8). A helpful way to understand this is through the example of rolling dice. Although the outcome of a single roll is not normally distributed, the averages of repeated rolls quickly form a bell-shaped curve (Whitfield, 2023, par. 10). Similarly, averaging salaries across many employees smooths the skewness, allowing for more accurate and meaningful analysis (Whitfield, 2023, par. 14). PEER REPLY 1: Review a classmate's Main Post. 1. What type of data is being evaluated, qualitative or quantitative? 2. What are the restrictions on using the Central Limit Theorem with that type of data? 3. What would be a good method for collecting the data required? If you agree with the current method, explain why. If you disagree with the current method, explain a method that you think would be better.
Rose Par posted Apr 20, 2025 11:00 AM Subscribe In a future business administration role, especially in human resources or finance, one type of data commonly collected is employee salary information. This includes salary amounts, job titles, departments, and years of service, typically gathered through internal HR systems or payroll software. It's essential to make reliable estimates from a sample of this data to identify pay trends, allocate budgets, and ensure fair compensation practices without surveying every employee (Whitfield, 2023, par. 1). Since salary data is often skewed due to a few high earners, the Central Limit Theorem (CLT) becomes essential. CLT states that as sample sizes grow larger, the distribution of sample means will approach a normal distribution, regardless of the population's original shape (Whitfield, 2023, par. 3 & 7). This means even if the salary distribution is not normal, taking a large enough sample, typically 30 or more employees, allows us to make valid inferences about the average salary (Whitfield, 2023, par. 8). A helpful way to understand this is through the example of rolling dice. Although the outcome of a single roll is not normally distributed, the averages of repeated rolls quickly form a bell-shaped curve (Whitfield, 2023, par. 10). Similarly, averaging salaries across many employees smooths the skewness, allowing for more accurate and meaningful analysis (Whitfield, 2023, par. 14). PEER REPLY 1: Review a classmate's Main Post. 1. What type of data is being evaluated, qualitative or quantitative? 2. What are the restrictions on using the Central Limit Theorem with that type of data? 3. What would be a good method for collecting the data required? If you agree with the current method, explain why. If you disagree with the current method, explain a method that you think would be better.
Algebra & Trigonometry with Analytic Geometry
13th Edition
ISBN:9781133382119
Author:Swokowski
Publisher:Swokowski
Chapter7: Analytic Trigonometry
Section7.6: The Inverse Trigonometric Functions
Problem 92E
Related questions
Question
Business discuss

Transcribed Image Text:Rose Par posted Apr 20, 2025 11:00 AM
Subscribe
In a future business administration role, especially in human resources or finance,
one type of data commonly collected is employee salary information. This includes
salary amounts, job titles, departments, and years of service, typically gathered
through internal HR systems or payroll software. It's essential to make reliable
estimates from a sample of this data to identify pay trends, allocate budgets, and
ensure fair compensation practices without surveying every employee (Whitfield,
2023, par. 1). Since salary data is often skewed due to a few high earners, the
Central Limit Theorem (CLT) becomes essential. CLT states that as sample sizes
grow larger, the distribution of sample means will approach a normal distribution,
regardless of the population's original shape (Whitfield, 2023, par. 3 & 7). This
means even if the salary distribution is not normal, taking a large enough sample,
typically 30 or more employees, allows us to make valid inferences about the
average salary (Whitfield, 2023, par. 8). A helpful way to understand this is
through the example of rolling dice. Although the outcome of a single roll is not
normally distributed, the averages of repeated rolls quickly form a bell-shaped
curve (Whitfield, 2023, par. 10). Similarly, averaging salaries across many
employees smooths the skewness, allowing for more accurate and meaningful
analysis (Whitfield, 2023, par. 14).

Transcribed Image Text:PEER REPLY 1:
Review a classmate's Main Post.
1. What type of data is being evaluated, qualitative or quantitative?
2. What are the restrictions on using the Central Limit Theorem with that type
of data?
3. What would be a good method for collecting the data required? If you
agree with the current method, explain why. If you disagree with the
current method, explain a method that you think would be better.
Expert Solution

This question has been solved!
Explore an expertly crafted, step-by-step solution for a thorough understanding of key concepts.
Step by step
Solved in 2 steps

Recommended textbooks for you
Algebra & Trigonometry with Analytic Geometry
Algebra
ISBN:
9781133382119
Author:
Swokowski
Publisher:
Cengage
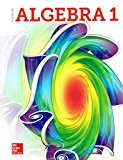
Glencoe Algebra 1, Student Edition, 9780079039897…
Algebra
ISBN:
9780079039897
Author:
Carter
Publisher:
McGraw Hill
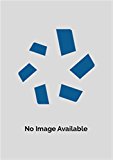
Big Ideas Math A Bridge To Success Algebra 1: Stu…
Algebra
ISBN:
9781680331141
Author:
HOUGHTON MIFFLIN HARCOURT
Publisher:
Houghton Mifflin Harcourt
Algebra & Trigonometry with Analytic Geometry
Algebra
ISBN:
9781133382119
Author:
Swokowski
Publisher:
Cengage
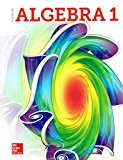
Glencoe Algebra 1, Student Edition, 9780079039897…
Algebra
ISBN:
9780079039897
Author:
Carter
Publisher:
McGraw Hill
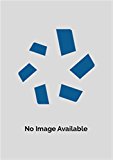
Big Ideas Math A Bridge To Success Algebra 1: Stu…
Algebra
ISBN:
9781680331141
Author:
HOUGHTON MIFFLIN HARCOURT
Publisher:
Houghton Mifflin Harcourt
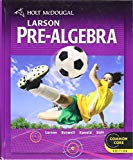
Holt Mcdougal Larson Pre-algebra: Student Edition…
Algebra
ISBN:
9780547587776
Author:
HOLT MCDOUGAL
Publisher:
HOLT MCDOUGAL
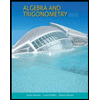
Algebra and Trigonometry (MindTap Course List)
Algebra
ISBN:
9781305071742
Author:
James Stewart, Lothar Redlin, Saleem Watson
Publisher:
Cengage Learning
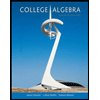
College Algebra
Algebra
ISBN:
9781305115545
Author:
James Stewart, Lothar Redlin, Saleem Watson
Publisher:
Cengage Learning