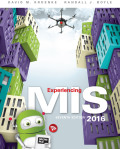
Experiencing MIS
7th Edition
ISBN: 9780134380421
Author: KROENKE
Publisher: PEARSON
expand_more
expand_more
format_list_bulleted
Expert Solution & Answer
Chapter 1, Problem 3ARQ
Explanation of Solution
Summary of IS related jobs:
- Spence and Hlatshwayo studied about the employment in the US from 1990 to 2008.
- They found that a tradable job as one that was not dependent on a particular location. And such jobs can be outsourced overseas.
- At that time computer related services had the strongest growth of any job type in that category.
- After the dot-com bust in 2000, the number of jobs dipped substantially...
Explanation of Solution
Growth rate of IS related jobs:
- According to Labour Satistics salary growth done by U.S Bureau, from 2012 to 2014 growth rates of all
information system related jobs are above 7 percent average for all occupation...
Explanation of Solution
How innovations in technology increase the value of IS-related jobs:
- The most significant determinant of employment and salary is the nature of work performed.
- In short, as the price of computer technology plummets, the value of jobs that benefit from it increases dramatically...
Expert Solution & Answer

Want to see the full answer?
Check out a sample textbook solution
Students have asked these similar questions
a. Product: Which business majors are presently in greatest demand by employers? Use
entry-level salaries as the primary indicator for demand.
b. Product: Which colleges or universities in your region pose the greatest competitive
threat to students with your major?
c. Price: What is the average salary for entry-level employees in your major and
geographic region? Is salary your top concern? Why or why not?
d. Place: Which areas of the country are currently experiencing the greatest employment
growth?
e. Promotion: What is your marketing plan? Describe how you plan to get your name and
qualifications in front of prospective employers. How can the Internet help you get noticed?
To what extent does an organization's success or failure depend on its workers' level of job satisfaction?
List and explain the factors that are the most important for your job satisfaction.
Chapter 1 Solutions
Experiencing MIS
Ch. 1.3 - Prob. 1SWCh. 1.3 - Prob. 2SWCh. 1.3 - Prob. 3SWCh. 1.3 - Prob. 4SWCh. 1.3 - Prob. 5SWCh. 1 - Prob. 1EGDQCh. 1 - Prob. 2EGDQCh. 1 - Prob. 3EGDQCh. 1 - Prob. 4EGDQCh. 1 - Prob. 5EGDQ
Ch. 1 - Prob. 6EGDQCh. 1 - Prob. 7EGDQCh. 1 - Prob. 1GDQCh. 1 - Prob. 2GDQCh. 1 - Prob. 3GDQCh. 1 - Prob. 4GDQCh. 1 - Prob. 1ARQCh. 1 - Prob. 2ARQCh. 1 - Prob. 3ARQCh. 1 - WHAT IS MIS? Identify the three important phrases...Ch. 1 - Prob. 5ARQCh. 1 - Prob. 3UYKCh. 1 - Prob. 4CECh. 1 - Prob. 5CECh. 1 - Prob. 6CECh. 1 - Prob. 7CECh. 1 - Prob. 8CECh. 1 - Prob. 9CSCh. 1 - Prob. 10CSCh. 1 - Prob. 11CSCh. 1 - Prob. 12CSCh. 1 - Prob. 13CSCh. 1 - Prob. 14CSCh. 1 - Prob. 15CS
Knowledge Booster
Similar questions
- A report summarized a survey of 2,315 working adults. The report indicates that 463 of the working adults surveyed said they were very concerned that their job will be automated, outsourced, or otherwise made obsolete in the next 5 years. The sample was selected in a way designed to produce a representative sample of working adults. Construct and interpret a 95% confidence interval for the proportion of working adults who are very concerned that their job will be automated, outsourced, or otherwise made obsolete in the next 5 years. Step 1 A confidence interval for a population proportion p can be constructed if the following criteria have been met. To determine if the criteria are met for constructing a confidence interval for the population proportion of workers who are concerned their job will become obsolete in the next 5 years, we must first calculate the sample proportion. There were 2,315 working adults surveyed, giving n = 2,315. It is given that 463 of those surveyed are…arrow_forwardIn 1-2 double spaced pages, please respond to the following: What is job analysis? In what areas (or tasks) in HR can you make use of the information it provides?arrow_forwardWhat effect does employee happiness have on a company's success or failure?arrow_forward
- To what extent does employee contentment in their jobs determine a company's fortunes?arrow_forwardAssignment 3 (On Unit 2): ...LWI practice for you also for the MID exam --/ \ Question 1 What is the tool that is more appropriate for a company manager to look and compare the sales in different parts of the country? Histogram 1 Pivot tables Trend lines 3 Curve Fitting 4 Question 2 4 من 10 مكتمل إرسال الحفظ لوقت لاحقarrow_forwardCan employment happiness be a predictor of whether a company will succeed or fail?arrow_forward
- What has affected the overall accessibility to V.R. therapy? Why are people not able to access V.R. therapy?arrow_forwardCreate a tablethat lists 10 or more possible career areas-including estimated annual salaries and brief job descriptions. Rate how much you think you would like each potential career area on a scale from 1 ("don't like'') to 10 (like the most'').Sort the careers from high to low rating and print the results.Sort the table according to annual salaries,from high to low, and then print the resulting table. Sort the table from the most liked to least liked, and then print the results.arrow_forwardTelecommuting is an increasingly popular work option: people who do their work in their homes rather than at a workplace, at least some of the time. Some groups oppose the growth of telecommuting as a job option, arguing that workers who are at home may work in less safe environments and may be required to work too many hours. Research both sides of this question: both the positives of telecommuting and the negatives. Give an ethical analysis of permitting or requiring employees to telecommute.arrow_forward
- How may the degree of pleasure experienced by workers at their place of employment affect the success or failure of a business?arrow_forwardExplain how and why business mergers and other ties have affected employee morale.arrow_forwardWhat differences are there between pensions and retiree healthcare?arrow_forward
arrow_back_ios
SEE MORE QUESTIONS
arrow_forward_ios
Recommended textbooks for you
- Fundamentals of Information SystemsComputer ScienceISBN:9781305082168Author:Ralph Stair, George ReynoldsPublisher:Cengage LearningPrinciples of Information Systems (MindTap Course...Computer ScienceISBN:9781285867168Author:Ralph Stair, George ReynoldsPublisher:Cengage LearningPrinciples of Information Systems (MindTap Course...Computer ScienceISBN:9781305971776Author:Ralph Stair, George ReynoldsPublisher:Cengage Learning
- Enhanced Discovering Computers 2017 (Shelly Cashm...Computer ScienceISBN:9781305657458Author:Misty E. Vermaat, Susan L. Sebok, Steven M. Freund, Mark Frydenberg, Jennifer T. CampbellPublisher:Cengage Learning
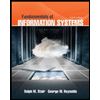
Fundamentals of Information Systems
Computer Science
ISBN:9781305082168
Author:Ralph Stair, George Reynolds
Publisher:Cengage Learning
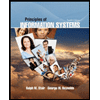
Principles of Information Systems (MindTap Course...
Computer Science
ISBN:9781285867168
Author:Ralph Stair, George Reynolds
Publisher:Cengage Learning
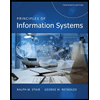
Principles of Information Systems (MindTap Course...
Computer Science
ISBN:9781305971776
Author:Ralph Stair, George Reynolds
Publisher:Cengage Learning
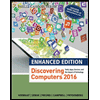
Enhanced Discovering Computers 2017 (Shelly Cashm...
Computer Science
ISBN:9781305657458
Author:Misty E. Vermaat, Susan L. Sebok, Steven M. Freund, Mark Frydenberg, Jennifer T. Campbell
Publisher:Cengage Learning