You decide to use the computational formula to calculate the Pearson correlation between the number of nonsocial days in a month and the number of illnesses per year. To do so, you call the number of nonsocial days in a month X and the number of illnesses per year Y. Then, you add up your data values (∑X and ∑Y), add up the squares of your data values (∑X2 and ∑Y2), and add up the products of your data values (∑XY). The following table summarizes your results: ∑X 590 ∑Y 380 ∑XY 4,887 ∑X2 10,456 ∑Y2 4,258 Find the following values: The sum of squares for the number of nonsocial days in a month is SSX= [ Select ] ["380", "590", "3494", "10456"] The sum of squares for the number of illnesses per year is SSY= [ Select ] ["380", "590", "1370", "4258"] The sum of products for the number of nonsocial days in a month and the number of illnesses per year is SP = [ Select ] ["403", "4258", "-403", "590"] The Pearson correlation coefficient is r = [ Select ] ["0.07", "0.18", "-0.18", "0.82"] Suppose you also want to predict the number of illnesses per year from the number of nonsocial days in a month among elderly men who live alone. The coefficient of determination is r2 = [ Select ] ["0.03", "0.07", "0.18", "0.82"] , indicating that [ Select ] ["3", "0.03", "18", "97"] % of the variability in the number of illnesses per year can be explained by the relationship between the number of illnesses per year and the number of nonsocial days in a month. When doing your analysis, suppose that, in addition to having data for the number of nonsocial days in a month for these elderly men who live alone, you have data for the number of face-to-face interactions in a month. You'd expect the correlation between the number of face-to-face interactions in a month and the number of nonsocial days in a month to be [ Select ] ["negative", "positive"] and the correlation between the number of face-to-face interactions in a month and the number of illnesses per year to be [ Select ] ["negative", "positive"
Correlation
Correlation defines a relationship between two independent variables. It tells the degree to which variables move in relation to each other. When two sets of data are related to each other, there is a correlation between them.
Linear Correlation
A correlation is used to determine the relationships between numerical and categorical variables. In other words, it is an indicator of how things are connected to one another. The correlation analysis is the study of how variables are related.
Regression Analysis
Regression analysis is a statistical method in which it estimates the relationship between a dependent variable and one or more independent variable. In simple terms dependent variable is called as outcome variable and independent variable is called as predictors. Regression analysis is one of the methods to find the trends in data. The independent variable used in Regression analysis is named Predictor variable. It offers data of an associated dependent variable regarding a particular outcome.
Suppose you are interested in the role of social support in immune
You decide to use the computational formula to calculate the Pearson
∑X | 590 |
∑Y | 380 |
∑XY | 4,887 |
∑X2 | 10,456 |
∑Y2 | 4,258 |
Find the following values:
The sum of squares for the number of nonsocial days in a month is SSX= [ Select ] ["380", "590", "3494", "10456"]
The sum of squares for the number of illnesses per year is SSY= [ Select ] ["380", "590", "1370", "4258"]
The sum of products for the number of nonsocial days in a month and the number of illnesses per year is SP = [ Select ] ["403", "4258", "-403", "590"]
The Pearson
Suppose you also want to predict the number of illnesses per year from the number of nonsocial days in a month among elderly men who live alone. The coefficient of determination is r2 = [ Select ] ["0.03", "0.07", "0.18", "0.82"] , indicating that [ Select ] ["3", "0.03", "18", "97"] % of the variability in the number of illnesses per year can be explained by the relationship between the number of illnesses per year and the number of nonsocial days in a month.
When doing your analysis, suppose that, in addition to having data for the number of nonsocial days in a month for these elderly men who live alone, you have data for the number of face-to-face interactions in a month. You'd expect the correlation between the number of face-to-face interactions in a month and the number of nonsocial days in a month to be [ Select ] ["negative", "positive"] and the correlation between the number of face-to-face interactions in a month and the number of illnesses per year to be [ Select ] ["negative", "positive"] .

Trending now
This is a popular solution!
Step by step
Solved in 3 steps with 5 images

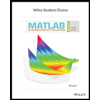
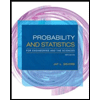
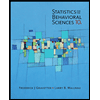
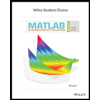
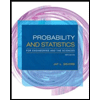
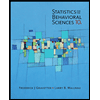
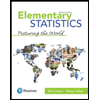
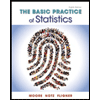
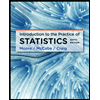