Year 1 2 3 4 January 742 741 896 951 1,030 February 697 700 793 861 1,032 March 776 774 885 938 1,126 April 898 932 1,055 1,109 1,285 May 1,030 1,099 1,204 1,274 1,468 June 1,107 1,223 1,326 1,422 1,637 July 1,165 1,290 1,303 1,486 1,611 August 1,216 1,349 1,436 1,555 1,608 September 1,208 1,341 1,473 1,604 1,528 October 1,131 1,296 1,453 1,600 1,420 November 971 1,066 1,170 1,403 1,119| December 783 901 1,023 1,209 1,013
Correlation
Correlation defines a relationship between two independent variables. It tells the degree to which variables move in relation to each other. When two sets of data are related to each other, there is a correlation between them.
Linear Correlation
A correlation is used to determine the relationships between numerical and categorical variables. In other words, it is an indicator of how things are connected to one another. The correlation analysis is the study of how variables are related.
Regression Analysis
Regression analysis is a statistical method in which it estimates the relationship between a dependent variable and one or more independent variable. In simple terms dependent variable is called as outcome variable and independent variable is called as predictors. Regression analysis is one of the methods to find the trends in data. The independent variable used in Regression analysis is named Predictor variable. It offers data of an associated dependent variable regarding a particular outcome.
Cannister, Inc., specializes in the manufacture of plastic containers.
The data on the monthly sales of 10-ounce shampoo bottles for the past 5 years are as follows:
a. Using the multiplicative seasonal method, calculate the monthly seasonal indices.
b. Develop a simple linear regression equation to forecast annual sales. For this regression, the dependent variable, Y, is the demand in each year and the independent variable, X, is the index for the year (i.e.,X = 1 for year 1, X = 2 for year 2, and so on until X = 5 for year 5).
c. Forecast the annual sales for year 6 by using the regression model you developed in part (b).
d. Prepare the seasonal forecast for each month by using the monthly seasonal indices calculated in part (a).


Trending now
This is a popular solution!
Step by step
Solved in 2 steps with 2 images

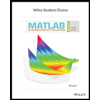
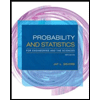
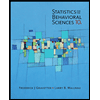
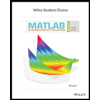
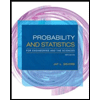
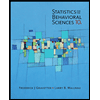
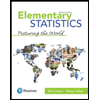
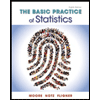
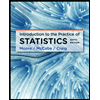