This is based on Modelling Real - World Data with Statistics. I would really appreciate your help to solve this question using statistical models and show some steps please 1. Testing the Global significance of the regression model – the F test 2. Division of SST between SSR and SSE 3. Comment on the R2 and adj-R2 values
Correlation
Correlation defines a relationship between two independent variables. It tells the degree to which variables move in relation to each other. When two sets of data are related to each other, there is a correlation between them.
Linear Correlation
A correlation is used to determine the relationships between numerical and categorical variables. In other words, it is an indicator of how things are connected to one another. The correlation analysis is the study of how variables are related.
Regression Analysis
Regression analysis is a statistical method in which it estimates the relationship between a dependent variable and one or more independent variable. In simple terms dependent variable is called as outcome variable and independent variable is called as predictors. Regression analysis is one of the methods to find the trends in data. The independent variable used in Regression analysis is named Predictor variable. It offers data of an associated dependent variable regarding a particular outcome.
This is based on Modelling Real - World Data with Statistics. I would really appreciate your help to solve this question using statistical models and show some steps please
1. Testing the Global significance of the regression model – the F test
2. Division of SST between SSR and SSE
3. Comment on the R2 and adj-R2 values


a.
The F-test tests for the overall significance of the regression model. The purpose of this test is to determine, whether the fitted model performs better than an intercept-only model, that is, whether the fitted model is significantly better than a model with no predictors.
The null and alternative hypotheses for the F-test displayed in the overall ANOVA table for the full model are:
H0: The intercept-only model, that is, the model with no predictors fits the data as well as the obtained model.
H1: The obtained model fits the data better than the intercept-only model.
The ANOVA table, or the “Analysis of Variance” table should be used to test the above hypotheses.
The F statistic value, given under the column of “F value” is 30.90, and the p-value corresponding to this F statistic value, given under the column of “Pr > F” is 0.0003.
The level of significance, α is not given.
The decision rule regarding a hypothesis testing problem using the p-value is:
Reject H0 if p-value ≤ α. Otherwise fail to reject H0.
Since p-value (= 0.0003) is less than most of the commonly used levels of significance, such as, α = 0.10, 0.05, 0.01, 0.001, etc. the decision is to reject the null hypothesis.
Thus, it can be concluded that the obtained full regression model fits the data better than the intercept-only model.
b.
The total sum of squares (SST) can be divided orthogonally into the regression sum of squares (SSR) and the error sum of squares (SSE). This means that the total sum of squares can be written as the sum of the regression sum of squares and the error sum of squares, as the cross product between the regression and error deviations from the respective means reduces to zero.
Thus, SST = SSR + SSE.
This can be verified from the output given in the “Analysis of Variance” table.
The sums of squares are given under the column “Sum of Squares”.
The total sum of squares is given corresponding to the row of “Corrected Total”, the regression sum of squares is given corresponding to the row of “Model”, and the error sum of squares is given corresponding to the row of “Error”.
The given values are: SST = 262.4, SSR = 235.6989; SSE = 26.7011.
Now, SSR + SSE = 235.6989 + 26.7011 = 262.4 = SST.
Thus, it is illustrated that SST can be divided orthogonally into SSR and SSE.
Step by step
Solved in 3 steps

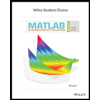
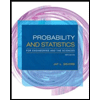
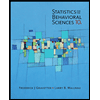
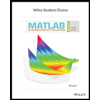
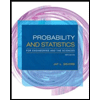
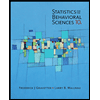
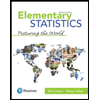
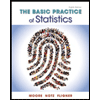
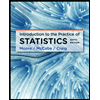