For the same houses from Question 1, a multiple regression model is now used to predict the price y (in $1000) of the n = 28 Seatle home prices based on two more explanatory variables in addition to square feet. The explanatory variables are then I1 = square feet; Price/Square Feet ; Bathrooms (Number of bathrooms). I2 = Bi, B2 and 33 are the corresponding parameters in the model. For all the testing problem hereby, set significance level a = 0.05. Response Price ($000) Summary of Fit RSquare RSquare Adj Root Mean Square Error Mean of Response Observations (or Sum Wgts) 0.9534 - Response Price (So00) 0.947575 29.38132 356.8214 Summary of Fit 28 RSquare RSquare Adj Root Mean Square Error Mean of Response Observations (or Sum Wgts) 0.131114 - Analysis of Variance 0.097695 121.8935 Sum of Squares Mean Square 3 423883.82 356.8214 Source DF F Ratio 28 Model 141295 163.6752 863 Prob > F Analysis of Variance Error 24 20718.29 C. Total - Parameter Estimates 27 444602.11 <.0001 Sum of Source DF F Ratio Squares Mean Square 58293.36 Model 58293.4 3.9234 Estimate Std Error tRatio Prob>lt| -8.13 <.0001 17.16 <.0001 12,51 <.0001 Term Error 26 386308.75 14858.0 Prob >F Intercept Square Feet 0.1895693 0.011048 Price/Sq Ft 1961.0355 156.728 Bathrooms -371.4508 45.67288 C. Total 27 444602.11 0.0583 Parameter Estimates -3.798639 11.36416 -0.33 0.7411 Term Estimate Std Error t Ratio Prob>t| 1.40 0.1731 1.98 0.0583 v Effect Tests Intercept Price/Sq Ft 1089.7999 550.1965 149.87283 106.9894 Sum of F Ratio Prob >F Effect Tests Source Nparm DF Squares Square Feet Price/Sq Ft Bathrooms 254169.70 294.4294 <.0001" <.0001" Sum of 135151.41 156.5590 Source Nparm DF Squares F Ratio Prob >F 96.45 0.1117 0.7411 Price/Sq Ft 58293.360 3.9234 0.0583 (d) Below are JMP summaries of different regression models for predicting selling price y based on various combinations of the variables: Square Feet(r), Price/Square Feet(r2) and Bathrooms(r3) From this output, state which model (i.e., selecting a combination of variables among r1, T2, 13) seems best for predicting y and give two reasons for your choice. (Cite values in the output to support your answer.) Model Variables: Model Square Feet Price/Sq Ft Bathrooms Square Feet, Price/Sq Ft Square Feet,Bathrooms Price/Sq Ft,Bathrooms Square Feet, Price/Sq Ft,Bathrooms AICC 0.5605 86.6916 334.278 337.274 BIC 202.3524 423.4988 463.5412 2.1117 158.5590 Reason #1: 296.4294 4.0000 Number RSquare RMSE Cp 0.1311 121.894 353.362 356.358 0.0534 127.230 355.761 358.758 0.9532 28.8546 274.314 277.903 0.6494 78.9607 330.688 334.277 0.3817 104.860 346.573 350.163 0.9534 29.3813 277.172 281.105 Reason #2:
For the same houses from Question 1, a multiple regression model is now used to predict the price y (in $1000) of the n = 28 Seatle home prices based on two more explanatory variables in addition to square feet. The explanatory variables are then I1 = square feet; Price/Square Feet ; Bathrooms (Number of bathrooms). I2 = Bi, B2 and 33 are the corresponding parameters in the model. For all the testing problem hereby, set significance level a = 0.05. Response Price ($000) Summary of Fit RSquare RSquare Adj Root Mean Square Error Mean of Response Observations (or Sum Wgts) 0.9534 - Response Price (So00) 0.947575 29.38132 356.8214 Summary of Fit 28 RSquare RSquare Adj Root Mean Square Error Mean of Response Observations (or Sum Wgts) 0.131114 - Analysis of Variance 0.097695 121.8935 Sum of Squares Mean Square 3 423883.82 356.8214 Source DF F Ratio 28 Model 141295 163.6752 863 Prob > F Analysis of Variance Error 24 20718.29 C. Total - Parameter Estimates 27 444602.11 <.0001 Sum of Source DF F Ratio Squares Mean Square 58293.36 Model 58293.4 3.9234 Estimate Std Error tRatio Prob>lt| -8.13 <.0001 17.16 <.0001 12,51 <.0001 Term Error 26 386308.75 14858.0 Prob >F Intercept Square Feet 0.1895693 0.011048 Price/Sq Ft 1961.0355 156.728 Bathrooms -371.4508 45.67288 C. Total 27 444602.11 0.0583 Parameter Estimates -3.798639 11.36416 -0.33 0.7411 Term Estimate Std Error t Ratio Prob>t| 1.40 0.1731 1.98 0.0583 v Effect Tests Intercept Price/Sq Ft 1089.7999 550.1965 149.87283 106.9894 Sum of F Ratio Prob >F Effect Tests Source Nparm DF Squares Square Feet Price/Sq Ft Bathrooms 254169.70 294.4294 <.0001" <.0001" Sum of 135151.41 156.5590 Source Nparm DF Squares F Ratio Prob >F 96.45 0.1117 0.7411 Price/Sq Ft 58293.360 3.9234 0.0583 (d) Below are JMP summaries of different regression models for predicting selling price y based on various combinations of the variables: Square Feet(r), Price/Square Feet(r2) and Bathrooms(r3) From this output, state which model (i.e., selecting a combination of variables among r1, T2, 13) seems best for predicting y and give two reasons for your choice. (Cite values in the output to support your answer.) Model Variables: Model Square Feet Price/Sq Ft Bathrooms Square Feet, Price/Sq Ft Square Feet,Bathrooms Price/Sq Ft,Bathrooms Square Feet, Price/Sq Ft,Bathrooms AICC 0.5605 86.6916 334.278 337.274 BIC 202.3524 423.4988 463.5412 2.1117 158.5590 Reason #1: 296.4294 4.0000 Number RSquare RMSE Cp 0.1311 121.894 353.362 356.358 0.0534 127.230 355.761 358.758 0.9532 28.8546 274.314 277.903 0.6494 78.9607 330.688 334.277 0.3817 104.860 346.573 350.163 0.9534 29.3813 277.172 281.105 Reason #2:
MATLAB: An Introduction with Applications
6th Edition
ISBN:9781119256830
Author:Amos Gilat
Publisher:Amos Gilat
Chapter1: Starting With Matlab
Section: Chapter Questions
Problem 1P
Related questions
Question
See the attached images for both the introduction and the question.

Transcribed Image Text:For the same houses from Question 1, a multiple regression model is now used to predict the price
y (in $1000) of the n = 28 Seatle home prices based on two more explanatory variables in addition
to square feet. The explanatory variables are then
I1 = square feet;
Price/Square Feet ;
Bathrooms (Number of bathrooms).
I2 =
Bi, B2 and 33 are the corresponding parameters in the model. For all the testing problem hereby,
set significance level a = 0.05.
Response Price ($000)
Summary of Fit
RSquare
RSquare Adj
Root Mean Square Error
Mean of Response
Observations (or Sum Wgts)
0.9534
- Response Price (So00)
0.947575
29.38132
356.8214
Summary of Fit
28
RSquare
RSquare Adj
Root Mean Square Error
Mean of Response
Observations (or Sum Wgts)
0.131114
- Analysis of Variance
0.097695
121.8935
Sum of
Squares Mean Square
3 423883.82
356.8214
Source
DF
F Ratio
28
Model
141295 163.6752
863 Prob > F
Analysis of Variance
Error
24
20718.29
C. Total
- Parameter Estimates
27 444602.11
<.0001
Sum of
Source
DF
F Ratio
Squares Mean Square
58293.36
Model
58293.4
3.9234
Estimate Std Error tRatio Prob>lt|
-8.13 <.0001
17.16 <.0001
12,51 <.0001
Term
Error
26 386308.75
14858.0 Prob >F
Intercept
Square Feet 0.1895693 0.011048
Price/Sq Ft 1961.0355 156.728
Bathrooms
-371.4508 45.67288
C. Total
27 444602.11
0.0583
Parameter Estimates
-3.798639 11.36416
-0.33 0.7411
Term
Estimate Std Error t Ratio Prob>t|
1.40 0.1731
1.98 0.0583
v Effect Tests
Intercept
Price/Sq Ft 1089.7999 550.1965
149.87283 106.9894
Sum of
F Ratio Prob >F
Effect Tests
Source
Nparm
DF
Squares
Square Feet
Price/Sq Ft
Bathrooms
254169.70 294.4294
<.0001"
<.0001"
Sum of
135151.41 156.5590
Source
Nparm
DF
Squares
F Ratio Prob >F
96.45
0.1117
0.7411
Price/Sq Ft
58293.360
3.9234
0.0583

Transcribed Image Text:(d) Below are JMP summaries of different regression models for predicting selling price y based on
various combinations of the variables: Square Feet(r), Price/Square Feet(r2) and Bathrooms(r3)
From this output, state which model (i.e., selecting a combination of variables among r1, T2, 13)
seems best for predicting y and give two reasons for your choice.
(Cite values in the output to support your answer.)
Model Variables:
Model
Square Feet
Price/Sq Ft
Bathrooms
Square Feet, Price/Sq Ft
Square Feet,Bathrooms
Price/Sq Ft,Bathrooms
Square Feet, Price/Sq Ft,Bathrooms
AICC
0.5605 86.6916 334.278 337.274
BIC
202.3524
423.4988
463.5412
2.1117
158.5590 Reason #1:
296.4294
4.0000
Number RSquare
RMSE
Cp
0.1311 121.894 353.362 356.358
0.0534 127.230 355.761 358.758
0.9532 28.8546 274.314 277.903
0.6494 78.9607 330.688 334.277
0.3817 104.860 346.573 350.163
0.9534 29.3813 277.172 281.105
Reason #2:
Expert Solution

This question has been solved!
Explore an expertly crafted, step-by-step solution for a thorough understanding of key concepts.
Step by step
Solved in 2 steps with 2 images

Knowledge Booster
Learn more about
Need a deep-dive on the concept behind this application? Look no further. Learn more about this topic, statistics and related others by exploring similar questions and additional content below.Recommended textbooks for you
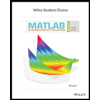
MATLAB: An Introduction with Applications
Statistics
ISBN:
9781119256830
Author:
Amos Gilat
Publisher:
John Wiley & Sons Inc
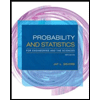
Probability and Statistics for Engineering and th…
Statistics
ISBN:
9781305251809
Author:
Jay L. Devore
Publisher:
Cengage Learning
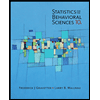
Statistics for The Behavioral Sciences (MindTap C…
Statistics
ISBN:
9781305504912
Author:
Frederick J Gravetter, Larry B. Wallnau
Publisher:
Cengage Learning
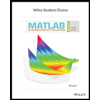
MATLAB: An Introduction with Applications
Statistics
ISBN:
9781119256830
Author:
Amos Gilat
Publisher:
John Wiley & Sons Inc
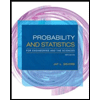
Probability and Statistics for Engineering and th…
Statistics
ISBN:
9781305251809
Author:
Jay L. Devore
Publisher:
Cengage Learning
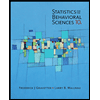
Statistics for The Behavioral Sciences (MindTap C…
Statistics
ISBN:
9781305504912
Author:
Frederick J Gravetter, Larry B. Wallnau
Publisher:
Cengage Learning
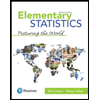
Elementary Statistics: Picturing the World (7th E…
Statistics
ISBN:
9780134683416
Author:
Ron Larson, Betsy Farber
Publisher:
PEARSON
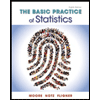
The Basic Practice of Statistics
Statistics
ISBN:
9781319042578
Author:
David S. Moore, William I. Notz, Michael A. Fligner
Publisher:
W. H. Freeman
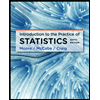
Introduction to the Practice of Statistics
Statistics
ISBN:
9781319013387
Author:
David S. Moore, George P. McCabe, Bruce A. Craig
Publisher:
W. H. Freeman