The Real World? Suppose a United States (CDC) created test for the Covid-19 virus has a false positive of only 2%, Pr(P | ~C). People who do not have the virus test positive. The accuracy of the test is very good. Pr(P | C) = 98%. If a person has the virus, they will test positive 98% on the time. Supposing a doctor determines that a particular patient, based on clinical signs, has an 80% chance of having the virus. C = 80%. After a first text comes back positive, a Bayesian calculation shows the patient has a 99% probability of having the virus. Pr(C | P) = [Pr(C) x Pr Pr(P | C)] / {[Pr(C) x Pr(P | C)] + [Pr(~C) x Pr(P| ~C)]} Pr(C | P) = (0.80 x 0.90) / [(0.80 x 0.90) + (0.20 x 0.02)] Pr(C | P) = 0.72/ (0.72 + 0.004) Pr(C | P) = 0.72 / 0.724 %3D Pr(C | P) = 0.99, 99% It took several precious weeks to develop this accurate test. Meanwhile a European test was available, but it had a false positive rate of 40%, Pr(P | ~C) = 0.40. Plus, Pr(P | C) = 0.70. If a person had the virus, the test would confirm 70% on the time. What would Pr(C | P) have been using the European test for a test of the same patient? Keep C = 80%. Show the calculation. Some medical supporters of the Trump administration argued that it was wise (many medical professionals disagreed) to wait for the more accurate CDC test. Correct? Did the administration have a reasonable basis for the delay in testing? There is not a black and white answer here. What have we learned in reasoning about risk? Apply as many course concepts as you can in giving your view.
![The Real World?
Suppose a United States
(CDC) created test for the
Covid-19 virus has a false
positive of only 2%,
Pr(P | ~C). People who do not
have the virus test positive.
The accuracy of the test is
very good. Pr(P | C) = 98%. If
a person has the virus, they
will test positive 98% on the
time. Supposing a doctor
determines that a particular
patient, based on clinical
signs, has an 80% chance of
having the virus. C = 80%.
After a first text comes back
positive, a Bayesian
calculation shows the patient
has a 99% probability of
having the virus.
Pr(C | P) = [Pr(C) x Pr
Pr(P | C)] / {[Pr(C) x Pr(P | C)]
+ [Pr(~C) x Pr(P| ~C)]}
Pr(C | P) = (0.80 x 0.90) /
[(0.80 x 0.90) + (0.20 x 0.02)]
Pr(C | P) = 0.72/ (0.72 +
0.004)
Pr(C | P) = 0.72 / 0.724
%3D](/v2/_next/image?url=https%3A%2F%2Fcontent.bartleby.com%2Fqna-images%2Fquestion%2Fe6dca9a0-fc4b-43b5-bd72-f47741689ef2%2Fb4990ef8-9ebe-4596-8387-d6cca6bc8528%2Fbm6wz1p.jpeg&w=3840&q=75)


Note:
Hey there! Thank you for the question. In the calculation that you have shown for the US test, the formula used is absolutely correct, but we found a small error in the calculation. We have re-done the calculation below, with the changes made by us given in bold:
Pr (C | P)
= {Pr (C) × Pr (P | C)} / {[Pr (C) × Pr (P | C)] + [Pr (CC) × Pr (P | CC)]}
= (0.80 × 0.98) / [(0.80 × 0.98) + (0.20 × 0.02)]
= 0.784 / (0.784 + 0.004)
= 0.784 / 0.788
≈ 0.9949
= 99.49%.
Thus, the value of Pr (C | P) using the US test is 99.49%.
The above probability value implies that, if a randomly selected person tests positive (and it is unknown whether the person truly has the virus or not), then there is a 99.49% chance that the person actually has the virus.
Now, 99.49% can approximately be written as 99.5%. This means that 99.5 persons, out of 100 persons testing positive, will actually have the virus. To make the conclusion easier to understand practically, multiply it my 10. Then it can be said that if 1,000 randomly selected persons test positive, then about 995 of them will actually have the disease.
This is quite a high probability of detecting the virus among people who test positive, although it can definitely be improved upon.
Now, when a person tests positive for a disease, or as a carrier of a disease, they are subjected to treatments. If the treatment for a disease has severe side-effects, then one must be extremely cautious before applying the treatment to a person. In this situation, the probability Pr (C | P) must be increased further (yes, even more than 99.49%), so that one can be almost sure that the persons being sent under the treatment absolutely require it, so that no one has to endure the severe side-effects of the treatment unless it is absolutely necessary in order to save their lives.
However, if the treatment does not have too much serious side-effects, but in comparison, it would a serious issue if a person goes untreated, then the above value of Pr (C | P) is acceptable. This is because even if a person tests positive by chance when they do not have the disease, it would not harm them even if they undergo the treatment. In such a situation, it is not absolutely necessary to improve upon the 99.49% probability before applying the treatment.
We request you to use your own thoughts and opinions while answering whether the decision to wait better for tests has been correct or not.
Introduction:
From the given info, Pr (C) = 0.80.
Therefore, Pr (CC) = 1 – Pr (C) = 1 – 0.80 = 0.20.
Since Pr (P | C) + Pr (PC | C) = 1, therefore Pr (PC | C) = 1 – Pr (P | C) = 1 – 0.70 = 0.30.
Since Pr (P | CC) + Pr (PC | CC) = 1, therefore Pr (PC | CC) = 1 – Pr (P | CC) = 1 – 0.40 = 0.60.
Step by step
Solved in 3 steps

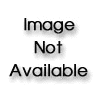
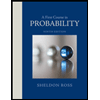
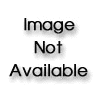
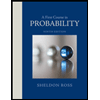