The linear regression alanysis shows that the pressure, measured in millibars is the explanatory variable, and the wind speed, measured in miles per hour is the reponse variable. The scatter plot shown below represents wind speed contrasted with the pressure in a hurricane, each dot in the scatter plot represents a hurricane. This figure reveals that there is a negative linear association between the two variables with a sample correlation coefficient of r = -0.9498. y = -1.095x + 1152.3 is the regression model that is used to describe the relationship between the two variables, the slope is a = -1.095, the y-intercepts is b = 1152.3, and the coefficient of determination is ?2 = 0.9022. The slope reveals that as the atmospheric pressure increases by one milibar, the predicted speed of wind will decrease by -1.095. Analysing the coefficient of determination displayed in the figure below, ?2 = 0.9022 means that approximately 90% of the variability on wind speed is explained by the least squared regression model. Supposing that there is an athmospheric pressure reading of 950 mb, the least squared regression model will estimate that the maximum sustained wind speed would be of 112.05 miles per hour (mph). In 2000, hurricane DEBBY recorded a maximum wind speed of 65 mph and in 2004 hurricane JEANNE recorded a maximum wind speed of 110 mph, but each of their corresponding athmosspheric pressure readings were unknown. Using the regression model obtained before, which is a linear approach that models the relationship between a dependent variable (y) and one independent variable (x), a prediction can be made towards these pressure readings. The general equation for linear regresion with one independent variable is y= ax+ b, where (a) represents the slope and (b) represents the y-intercepts. The equation is y=−1.095x+1152.3, from the given information it can be predicted that the atmospheric pressure of the hurricane DEBBY would be of 1081.125 mb, and the atmospheric pressure of the hurricane JEANNE would be of 1031.85 mb Given the information, what is your conclusion of this proyect. Does it proves the direct realtion between wind speeds and pressure? why?
Correlation
Correlation defines a relationship between two independent variables. It tells the degree to which variables move in relation to each other. When two sets of data are related to each other, there is a correlation between them.
Linear Correlation
A correlation is used to determine the relationships between numerical and categorical variables. In other words, it is an indicator of how things are connected to one another. The correlation analysis is the study of how variables are related.
Regression Analysis
Regression analysis is a statistical method in which it estimates the relationship between a dependent variable and one or more independent variable. In simple terms dependent variable is called as outcome variable and independent variable is called as predictors. Regression analysis is one of the methods to find the trends in data. The independent variable used in Regression analysis is named Predictor variable. It offers data of an associated dependent variable regarding a particular outcome.
The linear regression alanysis shows that the pressure, measured in millibars is the explanatory variable, and the wind speed, measured in miles per hour is the reponse variable. The
The slope reveals that as the atmospheric pressure increases by one milibar, the predicted speed of wind will decrease by -1.095. Analysing the coefficient of determination displayed in the figure below, ?2 = 0.9022 means that approximately 90% of the variability on wind speed is explained by the least squared regression model. Supposing that there is an athmospheric pressure reading of 950 mb, the least squared regression model will estimate that the maximum sustained wind speed would be of 112.05 miles per hour (mph). In 2000, hurricane DEBBY recorded a maximum wind speed of 65 mph and in 2004 hurricane JEANNE recorded a maximum wind speed of 110 mph, but each of their corresponding athmosspheric pressure readings were unknown. Using the regression model obtained before, which is a linear approach that models the relationship between a dependent variable (y) and one independent variable (x), a prediction can be made towards these pressure readings. The general equation for linear regresion with one independent variable is y= ax+ b, where (a) represents the slope and (b) represents the y-intercepts. The equation is y=−1.095x+1152.3, from the given information it can be predicted that the atmospheric pressure of the hurricane DEBBY would be of 1081.125 mb, and the atmospheric pressure of the hurricane JEANNE would be of 1031.85 mb
- Given the information, what is your conclusion of this proyect.
- Does it proves the direct realtion between wind speeds and pressure? why?


Trending now
This is a popular solution!
Step by step
Solved in 2 steps

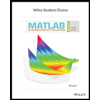
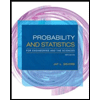
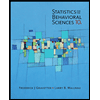
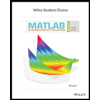
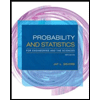
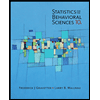
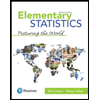
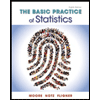
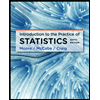