The county assessor is studying housing demand and is interested in developing a regression model to estimate the market value (i.e., selling price) of residential property within his jurisdiction. The assessor suspects that the most important variable affecting selling price (measured in thousands of dollars) is the size of house (measured in hundreds of square feet). He randomly selects 15 houses and measures both the selling price and size, as shown in the following table. Complete the table and then use it to determine the estimated regression line. Observation Size Selling Price (x 100 sq. ft.) (x $1,000) ii xixi yiyi xixiyiyi xi2xi2 yi2yi2 1 12 265.2 3,182.40 144.00 70,331.04 2 20.2 279.6 5,647.92 408.04 78,176.16 3 27 311.2 8,402.40 729.00 96,845.44 4 30 328.0 9,840.00 900.00 107,584.00 5 30 352.0 10,560.00 900.00 123,904.00 6 21.4 281.2 6,017.68 457.96 79,073.44 7 21.6 288.4 6,229.44 466.56 83,174.56 8 25.2 292.8 7,378.56 635.04 85,731.84 9 37.2 360.0 13,392.00 1,383.84 129,600.00 10 14.4 263.2 3,790.08 207.36 69,274.24 11 15 272.4 4,086.00 225.00 74,201.76 12 22.4 291.2 6,522.88 501.76 84,797.44 13 23.9 299.6 7,160.44 571.21 89,760.16 14 26.6 307.6 8,182.16 707.56 94,617.76 15 30.7 320.4 9,836.28 942.49 102,656.16 Total 357.60 4,512.80 ? 9,179.82 ? Regression Parameters Estimations Slope (ββ) ? Intercept (αα) ? In words, for each hundred square feet, the expected selling price of a house (?) by $ (?) . What is the standard error of the estimate (sese)? 10.249 8.513 8.812 What is the estimate of the standard deviation of the estimated slope (sbsb)? 0.344 0.401 0.333 Can the assessor reject the hypothesis (at the 0.05 level of significance) that there is no relationship (i.e., β=0β=0) between the price and size variables? (Hint: t0.025,13=2.160t0.025,13=2.160) No Yes Complete the following worksheet and then use it to calculate the coefficient of determination. ii xixi yiyi yˆy^ (yˆi−y¯)2y^i−y¯2 (yi−yˆ)2yi−y^2 (yi−y¯)2yi−y¯2 1 12 265.2 253.1 2,280.4 146.4 1,271.2 2 20.2 279.6 286.2 214.7 43.6 451.7 3 27 311.2 313.7 165.0 6.3 107.1 4 30 328.0 325.8 622.3 4.8 736.9 5 30 352.0 325.8 622.3 686.4 2,616.0 6 21.4 281.2 291.1 95.1 98.0 386.3 7 21.6 288.4 291.9 80.2 12.3 155.1 8 25.2 292.8 306.4 30.8 185.0 64.9 9 37.2 360.0 354.9 2,921.0 26.0 3,498.3 10 14.4 263.2 262.8 1,448.1 0.2 1,417.8 11 15 272.4 265.2 1,271.2 51.8 809.6 12 22.4 291.2 295.1 33.1 15.2 93.2 13 23.9 299.6 301.2 0.1 2.6 1.6 14 26.6 307.6 312.1 126.5 20.3 45.5 15 30.7 320.4 328.6 769.9 67.2 382.1 Total ? ? ? The coefficient of determination (r2r2) is: ? The F-ratio is (?) , which means that the assessor (?) reject, at the 5% level of significance, the null hypothesis that there is no relationship between the selling price and the area of the house. (Hint: The critical value of F0.05,1,13F0.05,1,13 is 4.67.) Which of the following is an approximate 95% prediction interval for the selling price of a house having an area (size) of 15 (hundred) square feet? 244.7 to 285.7 177.7 to 211.7 174.2 to 215.2
Correlation
Correlation defines a relationship between two independent variables. It tells the degree to which variables move in relation to each other. When two sets of data are related to each other, there is a correlation between them.
Linear Correlation
A correlation is used to determine the relationships between numerical and categorical variables. In other words, it is an indicator of how things are connected to one another. The correlation analysis is the study of how variables are related.
Regression Analysis
Regression analysis is a statistical method in which it estimates the relationship between a dependent variable and one or more independent variable. In simple terms dependent variable is called as outcome variable and independent variable is called as predictors. Regression analysis is one of the methods to find the trends in data. The independent variable used in Regression analysis is named Predictor variable. It offers data of an associated dependent variable regarding a particular outcome.
Observation
|
Size
|
Selling Price
|
|
||
---|---|---|---|---|---|
(x 100 sq. ft.)
|
(x $1,000)
|
||||
ii | xixi | yiyi | xixiyiyi | xi2xi2 | yi2yi2 |
1 | 12 | 265.2 | 3,182.40 | 144.00 | 70,331.04 |
2 | 20.2 | 279.6 | 5,647.92 | 408.04 | 78,176.16 |
3 | 27 | 311.2 | 8,402.40 | 729.00 | 96,845.44 |
4 | 30 | 328.0 | 9,840.00 | 900.00 | 107,584.00 |
5 | 30 | 352.0 | 10,560.00 | 900.00 | 123,904.00 |
6 | 21.4 | 281.2 | 6,017.68 | 457.96 | 79,073.44 |
7 | 21.6 | 288.4 | 6,229.44 | 466.56 | 83,174.56 |
8 | 25.2 | 292.8 | 7,378.56 | 635.04 | 85,731.84 |
9 | 37.2 | 360.0 | 13,392.00 | 1,383.84 | 129,600.00 |
10 | 14.4 | 263.2 | 3,790.08 | 207.36 | 69,274.24 |
11 | 15 | 272.4 | 4,086.00 | 225.00 | 74,201.76 |
12 | 22.4 | 291.2 | 6,522.88 | 501.76 | 84,797.44 |
13 | 23.9 | 299.6 | 7,160.44 | 571.21 | 89,760.16 |
14 | 26.6 | 307.6 | 8,182.16 | 707.56 | 94,617.76 |
15 | 30.7 | 320.4 | 9,836.28 | 942.49 | 102,656.16 |
Total | 357.60 | 4,512.80 | ? | 9,179.82 | ? |
Regression Parameters
|
Estimations
|
---|---|
Slope (ββ) | ? |
Intercept (αα) | ? |
ii
|
xixi
|
yiyi
|
yˆy^
|
(yˆi−y¯)2y^i−y¯2
|
(yi−yˆ)2yi−y^2
|
(yi−y¯)2yi−y¯2
|
---|---|---|---|---|---|---|
1 | 12 | 265.2 | 253.1 | 2,280.4 | 146.4 | 1,271.2 |
2 | 20.2 | 279.6 | 286.2 | 214.7 | 43.6 | 451.7 |
3 | 27 | 311.2 | 313.7 | 165.0 | 6.3 | 107.1 |
4 | 30 | 328.0 | 325.8 | 622.3 | 4.8 | 736.9 |
5 | 30 | 352.0 | 325.8 | 622.3 | 686.4 | 2,616.0 |
6 | 21.4 | 281.2 | 291.1 | 95.1 | 98.0 | 386.3 |
7 | 21.6 | 288.4 | 291.9 | 80.2 | 12.3 | 155.1 |
8 | 25.2 | 292.8 | 306.4 | 30.8 | 185.0 | 64.9 |
9 | 37.2 | 360.0 | 354.9 | 2,921.0 | 26.0 | 3,498.3 |
10 | 14.4 | 263.2 | 262.8 | 1,448.1 | 0.2 | 1,417.8 |
11 | 15 | 272.4 | 265.2 | 1,271.2 | 51.8 | 809.6 |
12 | 22.4 | 291.2 | 295.1 | 33.1 | 15.2 | 93.2 |
13 | 23.9 | 299.6 | 301.2 | 0.1 | 2.6 | 1.6 |
14 | 26.6 | 307.6 | 312.1 | 126.5 | 20.3 | 45.5 |
15 | 30.7 | 320.4 | 328.6 | 769.9 | 67.2 | 382.1 |
Total | ? | ? | ? |

Trending now
This is a popular solution!
Step by step
Solved in 3 steps

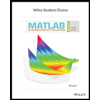
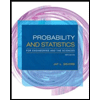
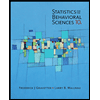
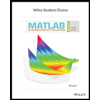
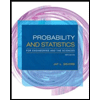
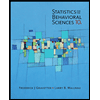
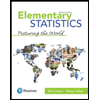
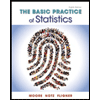
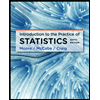