S₁ Ds S₂ S3 D₁ Ꭲ, | Encoder Output (shifted right) Output 0.0 00 Input Embedding Muti-Head Add & Attention Norm Feed Forward Add & Norm Encoder #N| Muti-Head Add & Attention Norm Muti-Head Attention Add & Norm Forward Feed Add & Norm Decoder #N Linear 00 T₁ S₁ T₁ S₁ S₂ S3 Linear Кт VT Qs Vs Ks Cross Adaptive Layer Sigmoid Muti-Head Cross Attention Add & Norm Muti-Head Attention Add & Norm ypred T₁ S₁ S₁ LMMD LMSE Feed Forward Add & Norm Feed Forward Add & Norm Ldistillation Y label T1 S₁ Cross Adaptive Layer | Ltotal = arg min (waLdistillation+ WMLMMD + W,Lregression) Fig. 6. Architecture of the proposed MSCATN. 7
Please provide me with the output image of both of them . below are the diagrams code
make sure to update the code and mentionned clearly each section also the digram should be clearly describe like in the attached image. please do not provide the same answer like in other question . I repost this question because it does not satisfy the requirment I need in terms of clarifty the output of both code are not very well details
I have two diagram :
first diagram code
graph LR subgraph Teacher Model (Pretrained) Input_Teacher[Input C (Complete Data)] --> Teacher_Encoder[Transformer Encoder T] Teacher_Encoder --> Teacher_Prediction[Teacher Prediction y_T] Teacher_Encoder --> Teacher_Features[Internal Features F_T] end subgraph Student_A_Model[Student Model A (Handles Missing Values)] Input_Student_A[Input M (Data with Missing Values)] --> Student_A_Encoder[Transformer Encoder E_A] Student_A_Encoder --> Student_A_Prediction[Student A Prediction y_A] Student_A_Encoder --> Student_A_Features[Student A Features F_A] end subgraph Knowledge_Distillation_A [Knowledge Distillation (Student A)] Teacher_Prediction -- Logits Distillation Loss (L_logits_A) --> Total_Loss_A Teacher_Features -- Feature Alignment Loss (L_feature_A) --> Total_Loss_A Ground_Truth_A[Ground Truth y_gt] -- Prediction Loss (L_pred_A) --> Total_Loss_A Total_Loss_A -- Backpropagation --> Student_A_Encoder end Teacher_Prediction -- Logits --> Logits_Distillation_A Teacher_Features -- Features --> Feature_Alignment_A Feature_Alignment_A -- Feature Alignment Loss (L_feature_A) --> Knowledge_Distillation_A Logits_Distillation_A -- Logits Distillation Loss (L_logits_A) --> Knowledge_Distillation_A Ground_Truth_A -- Labels --> Prediction_Loss_A Prediction_Loss_A -- Prediction Loss (L_pred_A) --> Knowledge_Distillation_A style Knowledge_Distillation_A fill:#ccf,stroke:#333,stroke-width:2px style Total_Loss_A fill:#fff,stroke:#333,stroke-width:2px
second diagram code :
graph LR subgraph Teacher_Model_B [Teacher Model (Pretrained)] Input_Teacher_B[Input C (Complete Data)] --> Teacher_Encoder_B[Transformer Encoder T] Teacher_Encoder_B --> Teacher_Prediction_B[Teacher Prediction y_T] Teacher_Encoder_B --> Teacher_Features_B[Internal Features F_T] end subgraph Student_B_Model [Student Model B (Handles Missing Labels)] Input_Student_B[Input C (Complete Data)] --> Student_B_Encoder[Transformer Encoder E_B] Student_B_Encoder --> Student_B_Prediction[Student B Prediction y_B] end subgraph Knowledge_Distillation_B [Knowledge Distillation (Student B)] Teacher_Prediction_B -- Logits Distillation Loss (L_logits_B) --> Total_Loss_B Teacher_Features_B -- Feature Alignment Loss (L_feature_B) --> Total_Loss_B Partial_Labels_B[Partial Labels y_p] -- Prediction Loss (L_pred_B) --> Total_Loss_B Total_Loss_B -- Backpropagation --> Student_B_Encoder end Teacher_Prediction_B -- Logits --> Logits_Distillation_B Teacher_Features_B -- Features --> Feature_Alignment_B Feature_Alignment_B -- Feature Alignment Loss (L_feature_B) --> Knowledge_Distillation_B Logits_Distillation_B -- Logits Distillation Loss (L_logits_B) --> Knowledge_Distillation_B Partial_Labels_B -- Available Labels --> Prediction_Loss_B Prediction_Loss_B -- Prediction Loss (L_pred_B) --> Knowledge_Distillation_B style Knowledge_Distillation_B fill:#aed,stroke:#333,stroke-width:2px style Total_Loss_B fill:#fff,stroke:#333,stroke-width:2px
Please provide me with the output in image of both of them


Step by step
Solved in 2 steps

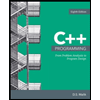
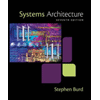
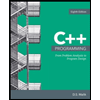
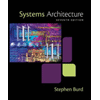
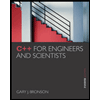
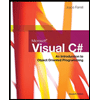