Question 6: Causal Inference Analysis of Determinants Affecting Mathematics Performance Objective: Determine the causal effects of various factors on students' mathematics scores. Specifically, investigate whether increasing study hours leads to higher math scores while accounting for potential confounders. Background: Understanding the causal relationships between study habits and academic performance can inform educational strategies and interventions. However, observational data often contain confounding variables that may bias the estimated effects. This analysis aims to employ causal inference techniques to estimate the true effect of study hours on math scores. Dataset Assumptions: Assume that mathnew.csv includes the following additional variables relevant to causal analysis: PreviousMathScore: The math score from the previous semester. • • • • • MotivationLevel: A self-reported measure of the student's motivation on a scale from 1 to 10. Access To Resources: Indicator of access to educational resources (Yes, No). TeacherQuality: Rating of the teacher's quality on a scale from 1 to 5. SchoolFunding: Amount of funding per student in the school. Tasks: 1. Conceptual Framework: a. Develop a causal diagram (Directed Acyclic Graph - DAG) illustrating the hypothesized relationships between Study Hours, MathScore, and potential confounders (Age, Gender, ParentalEducation, SocioEconomic Status, PreviousMathScore, MotivationLevel, AccessToResources, TeacherQuality, SchoolFunding). b. Identify the set of variables that need to be controlled for to estimate the causal effect of StudyHours on MathScore. 2. Data Preparation: • • a. Load the mathnew.csv dataset into R. b. Handle missing data using multiple imputation techniques (e.g., using the mice package). c. Convert categorical variables (Gender, Parental Education, SocioEconomic Status, AccessToResources) into appropriate numerical formats using dummy encoding or factor variables. d. Check for and address any multicollinearity issues among the predictors.
Question 6: Causal Inference Analysis of Determinants Affecting Mathematics Performance Objective: Determine the causal effects of various factors on students' mathematics scores. Specifically, investigate whether increasing study hours leads to higher math scores while accounting for potential confounders. Background: Understanding the causal relationships between study habits and academic performance can inform educational strategies and interventions. However, observational data often contain confounding variables that may bias the estimated effects. This analysis aims to employ causal inference techniques to estimate the true effect of study hours on math scores. Dataset Assumptions: Assume that mathnew.csv includes the following additional variables relevant to causal analysis: PreviousMathScore: The math score from the previous semester. • • • • • MotivationLevel: A self-reported measure of the student's motivation on a scale from 1 to 10. Access To Resources: Indicator of access to educational resources (Yes, No). TeacherQuality: Rating of the teacher's quality on a scale from 1 to 5. SchoolFunding: Amount of funding per student in the school. Tasks: 1. Conceptual Framework: a. Develop a causal diagram (Directed Acyclic Graph - DAG) illustrating the hypothesized relationships between Study Hours, MathScore, and potential confounders (Age, Gender, ParentalEducation, SocioEconomic Status, PreviousMathScore, MotivationLevel, AccessToResources, TeacherQuality, SchoolFunding). b. Identify the set of variables that need to be controlled for to estimate the causal effect of StudyHours on MathScore. 2. Data Preparation: • • a. Load the mathnew.csv dataset into R. b. Handle missing data using multiple imputation techniques (e.g., using the mice package). c. Convert categorical variables (Gender, Parental Education, SocioEconomic Status, AccessToResources) into appropriate numerical formats using dummy encoding or factor variables. d. Check for and address any multicollinearity issues among the predictors.
Big Ideas Math A Bridge To Success Algebra 1: Student Edition 2015
1st Edition
ISBN:9781680331141
Author:HOUGHTON MIFFLIN HARCOURT
Publisher:HOUGHTON MIFFLIN HARCOURT
Chapter4: Writing Linear Equations
Section: Chapter Questions
Problem 14CR
Related questions
Question
100%
These question need to be solved using R with the given data, please do not provide AI solution , also i need detailed solution , do everything in detail which is required, answer it as soon as possible.

Transcribed Image Text:Question 6: Causal Inference Analysis of Determinants Affecting
Mathematics Performance
Objective:
Determine the causal effects of various factors on students' mathematics scores. Specifically,
investigate whether increasing study hours leads to higher math scores while accounting for
potential confounders.
Background:
Understanding the causal relationships between study habits and academic performance can inform
educational strategies and interventions. However, observational data often contain confounding
variables that may bias the estimated effects. This analysis aims to employ causal inference
techniques to estimate the true effect of study hours on math scores.
Dataset Assumptions:
Assume that mathnew.csv includes the following additional variables relevant to causal analysis:
PreviousMathScore: The math score from the previous semester.
•
•
•
•
•
MotivationLevel: A self-reported measure of the student's motivation on a scale from 1 to 10.
Access To Resources: Indicator of access to educational resources (Yes, No).
TeacherQuality: Rating of the teacher's quality on a scale from 1 to 5.
SchoolFunding: Amount of funding per student in the school.
Tasks:
1. Conceptual Framework:
a. Develop a causal diagram (Directed Acyclic Graph - DAG) illustrating the hypothesized
relationships between Study Hours, MathScore, and potential confounders (Age, Gender,
ParentalEducation, SocioEconomic Status, PreviousMathScore, MotivationLevel,
AccessToResources, TeacherQuality, SchoolFunding).
b. Identify the set of variables that need to be controlled for to estimate the causal effect of
StudyHours on MathScore.
2. Data Preparation:
•
•
a. Load the mathnew.csv dataset into R.
b. Handle missing data using multiple imputation techniques (e.g., using the mice
package).
c. Convert categorical variables (Gender, Parental Education, SocioEconomic Status,
AccessToResources) into appropriate numerical formats using dummy encoding or factor
variables.
d. Check for and address any multicollinearity issues among the predictors.
Expert Solution

This question has been solved!
Explore an expertly crafted, step-by-step solution for a thorough understanding of key concepts.
Step by step
Solved in 2 steps

Recommended textbooks for you
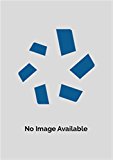
Big Ideas Math A Bridge To Success Algebra 1: Stu…
Algebra
ISBN:
9781680331141
Author:
HOUGHTON MIFFLIN HARCOURT
Publisher:
Houghton Mifflin Harcourt
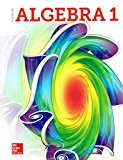
Glencoe Algebra 1, Student Edition, 9780079039897…
Algebra
ISBN:
9780079039897
Author:
Carter
Publisher:
McGraw Hill
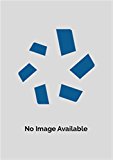
Big Ideas Math A Bridge To Success Algebra 1: Stu…
Algebra
ISBN:
9781680331141
Author:
HOUGHTON MIFFLIN HARCOURT
Publisher:
Houghton Mifflin Harcourt
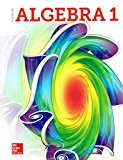
Glencoe Algebra 1, Student Edition, 9780079039897…
Algebra
ISBN:
9780079039897
Author:
Carter
Publisher:
McGraw Hill