PYTHON ASSIGNMENT ON 2D IMAGE MANIPULATION (i) Run the following: >>> import numpy as np >>> import scipy as sp >>> import matplotlib.pyplot as plt >>> n = 10 >>> l = 256 >>> im = np.zeros((l, l)) >>> np.random.seed(1) >>> points = l*np.random.random((2, n**2)) >>> im[(points[0]).astype(np.int), (points[1]).astype(np.int)]= 1 >>> im = ndimage.gaussian_filter(im, sigma=l/(4.*n)) >>> mask = (im > im.mean()).astype(np.float) >>> mask += 0.1 * im >>> img = mask + 0.2*np.random.randn(*mask.shape) >>> hist, bin_edges = np.histogram(img, bins=60) >>> bin_centers = 0.5*(bin_edges[:-1] + bin_edges[1:]) >>> binary_img = img > 0.5 >>> plt.imshow(binary_img) >>> plt.show()
PYTHON ASSIGNMENT ON 2D IMAGE MANIPULATION
(i) Run the following:
>>> import numpy as np
>>> import scipy as sp
>>> import matplotlib.pyplot as plt
>>> n = 10
>>> l = 256
>>> im = np.zeros((l, l))
>>> np.random.seed(1)
>>> points = l*np.random.random((2, n**2))
>>> im[(points[0]).astype(np.int), (points[1]).astype(np.int)]= 1
>>> im = ndimage.gaussian_filter(im, sigma=l/(4.*n))
>>> mask = (im > im.mean()).astype(np.float)
>>> mask += 0.1 * im
>>> img = mask + 0.2*np.random.randn(*mask.shape)
>>> hist, bin_edges = np.histogram(img, bins=60)
>>> bin_centers = 0.5*(bin_edges[:-1] + bin_edges[1:])
>>> binary_img = img > 0.5
>>> plt.imshow(binary_img)
>>> plt.show()
(ii) Firstly, try and clean up the random noise with 2D filters, and create a subplot for each filter you try, with a descriptive title.
i.e. Try a Gaussian filter, a Median filter, and design at least ONE filter of your own.
What does the best job?
(iii)Now experiment with morphological math (i.e., combinations of opening, closing, erosion, and dilation operations).
What sequence of these operations works best for cleaning up noise?
Choose three different permutations of parameters and explain IN WORDS what you think the result will be, and why.
Create a subplot for each.
(iv)In the original image, count BY HAND how many separate clusters you think there should be (think of this as "overlapping bacteria", and a game of "count the bacteria").
Naturally, the shapes of the bacteria to be cylindrical or spherical, and overlapping ones might not simply count as one.
Next, use histogram segmentation and connectivity kernels to generate a count of different families.
Determine how close is the count to the estimate?
v) To improve the match between your eye and your computer, progressively erode the structures, such that narrow links between overlapping bacteria can become "eroded away".
>>>ndimage.binary_erosion(binary_img, iterations=1) has an iteration input (i.e., it runs the
Run it from 1 to 10 iterations, and for every run, compute your estimate of cluster numbers, and produce a subplot with the eroded image and a title that shows how many families are in the image.
Determine which works best with the previous estimate?

Step by step
Solved in 4 steps with 1 images

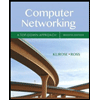
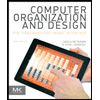
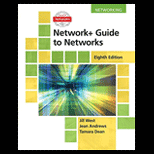
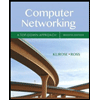
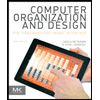
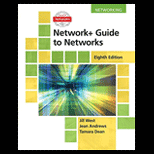
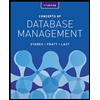
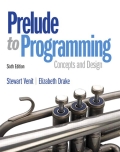
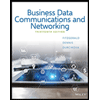