Problem 3: Expressive Power of Convolutional Neural Networks (CNNs) Compared to Fully Connected Networks Statement: Demonstrate that convolutional neural networks (CNNs) with a fixed number of layers and sufficient width can represent certain classes of functions exponentially more efficiently (in terms of the number of parameters) than fully connected neural networks of the same depth. Key Points for the Proof: • • Identify specific function classes that benefit from the local connectivity and weight sharing properties of CNNs. Construct explicit examples where CNNs achieve the representation with fewer parameters. • Compare the parameter counts required for equivalent expressiveness in fully connected networks. • Utilize combinatorial arguments to show the exponential efficiency.
Problem 3: Expressive Power of Convolutional Neural Networks (CNNs) Compared to Fully Connected Networks Statement: Demonstrate that convolutional neural networks (CNNs) with a fixed number of layers and sufficient width can represent certain classes of functions exponentially more efficiently (in terms of the number of parameters) than fully connected neural networks of the same depth. Key Points for the Proof: • • Identify specific function classes that benefit from the local connectivity and weight sharing properties of CNNs. Construct explicit examples where CNNs achieve the representation with fewer parameters. • Compare the parameter counts required for equivalent expressiveness in fully connected networks. • Utilize combinatorial arguments to show the exponential efficiency.
College Algebra (MindTap Course List)
12th Edition
ISBN:9781305652231
Author:R. David Gustafson, Jeff Hughes
Publisher:R. David Gustafson, Jeff Hughes
Chapter6: Linear Systems
Section6.8: Linear Programming
Problem 40E
Related questions
Question

Transcribed Image Text:Problem 3: Expressive Power of Convolutional Neural Networks (CNNs)
Compared to Fully Connected Networks
Statement: Demonstrate that convolutional neural networks (CNNs) with a fixed number of layers
and sufficient width can represent certain classes of functions exponentially more efficiently (in terms
of the number of parameters) than fully connected neural networks of the same depth.
Key Points for the Proof:
•
•
Identify specific function classes that benefit from the local connectivity and weight sharing
properties of CNNs.
Construct explicit examples where CNNs achieve the representation with fewer parameters.
• Compare the parameter counts required for equivalent expressiveness in fully connected
networks.
•
Utilize combinatorial arguments to show the exponential efficiency.
Expert Solution

This question has been solved!
Explore an expertly crafted, step-by-step solution for a thorough understanding of key concepts.
Step by step
Solved in 2 steps with 3 images

Recommended textbooks for you
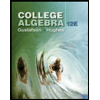
College Algebra (MindTap Course List)
Algebra
ISBN:
9781305652231
Author:
R. David Gustafson, Jeff Hughes
Publisher:
Cengage Learning
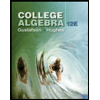
College Algebra (MindTap Course List)
Algebra
ISBN:
9781305652231
Author:
R. David Gustafson, Jeff Hughes
Publisher:
Cengage Learning