(Math) Let D be the distribution over the data points (x, y), and let H be thehypothesis class, in which one would like to find a function f that has a small expected loss L(f) by minimizing the empirical loss Lˆ(f). A few definitions/terminologies:• The best function among all (measurable) functions is called Bayes hypothesis:f∗ = arg inffL(f).• The best function in the hypothesis class is denoted asfopt = arg inff∈HL(f)• The function that minimizes the empirical loss in the hypothesis class is denoted asˆfopt = arg inff∈HLˆ(f)• The function output by the algorithm is denoted as ˆf. (It can be different from ˆfopt since the optimization may not find the best solution.)• The difference between the loss of f∗ and fopt is called approximation error:xapp = L(fopt) − L(f∗)which measures the error introduced in building the model/hypothesis class.• The difference between the loss of fopt and ˆfopt is called estimation error:xest = L(ˆfopt) − L(fopt)which measures the error introduced by using finite data to approximate the distribution D.• The difference between the loss of ˆfopt and ˆf is called optimization error:xopt = L(ˆf) − L(ˆfopt)which measures the error introduced in optimization.• The difference between the loss of f∗ and ˆf is called excess risk:xexc = L(ˆf) − L(f∗)which measures the distance from the output of the algorithm to the best solution possible.(1) Show that xexc = xapp + xest + xopt. Comments: This means that to get better performance, one can think of: 1) building a hypothesis class closer to the ground truth; 2) collecting more data; 3) improving the optimization. (2) Typically, when one has enough data, the empirical loss concentrates around the expected loss: there exists xcon > 0, such that for any f ∈ H, |Lˆ(f) − L(f)| ≤ xcon. Show thatin this case, xest ≤ 2 xcon.Comments: This means that to get small estimation error, the number of data points should be large enough so that concentration happens. The number of data points needed to get concentration xcon is called sample complexity, which is an important topic in learning theory and statistics.
Continuous Probability Distributions
Probability distributions are of two types, which are continuous probability distributions and discrete probability distributions. A continuous probability distribution contains an infinite number of values. For example, if time is infinite: you could count from 0 to a trillion seconds, billion seconds, so on indefinitely. A discrete probability distribution consists of only a countable set of possible values.
Normal Distribution
Suppose we had to design a bathroom weighing scale, how would we decide what should be the range of the weighing machine? Would we take the highest recorded human weight in history and use that as the upper limit for our weighing scale? This may not be a great idea as the sensitivity of the scale would get reduced if the range is too large. At the same time, if we keep the upper limit too low, it may not be usable for a large percentage of the population!
(Math)
Let D be the distribution over the data points (x, y), and let H be the
hypothesis class, in which one would like to find a function f that has a small expected loss L(f) by minimizing the empirical loss Lˆ(f). A few definitions/terminologies:
• The best function among all (measurable)
f∗ = arg inffL(f).
• The best function in the hypothesis class is denoted as
fopt = arg inff∈HL(f)
• The function that minimizes the empirical loss in the hypothesis class is denoted as
ˆfopt = arg inff∈HLˆ(f)
• The function output by the algorithm is denoted as ˆf. (It can be different from ˆfopt since the optimization may not find the best solution.)
• The difference between the loss of f∗ and fopt is called approximation error:
xapp = L(fopt) − L(f∗)
which measures the error introduced in building the model/hypothesis class.
• The difference between the loss of fopt and ˆfopt is called estimation error:
xest = L(ˆfopt) − L(fopt)
which measures the error introduced by using finite data to approximate the distribution D.
• The difference between the loss of ˆfopt and ˆf is called optimization error:
xopt = L(ˆf) − L(ˆfopt)
which measures the error introduced in optimization.
• The difference between the loss of f∗ and ˆf is called excess risk:
xexc = L(ˆf) − L(f∗)
which measures the distance from the output of the algorithm to the best solution possible.
(1) Show that xexc = xapp + xest + xopt.
Comments: This means that to get better performance, one can think of: 1) building a hypothesis class closer to the ground truth; 2) collecting more data; 3) improving the optimization.
(2) Typically, when one has enough data, the empirical loss concentrates around the expected loss: there exists xcon > 0, such that for any f ∈ H, |Lˆ(f) − L(f)| ≤ xcon. Show that
in this case, xest ≤ 2 xcon.
Comments: This means that to get small estimation error, the number of data points should be large enough so that concentration happens. The number of data points needed to get concentration xcon is called sample complexity, which is an important topic in learning theory and statistics.

Trending now
This is a popular solution!
Step by step
Solved in 4 steps with 1 images

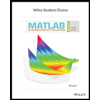
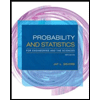
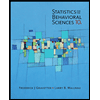
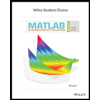
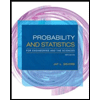
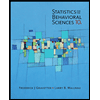
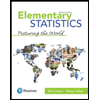
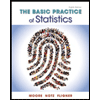
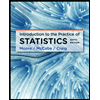