I've had to ask this question multiple times, to which a few had either been copied from the previous answer or from the internet so please, if you can produce a direct and concise answer it would be much appreciated. I have recently produced a linear regression model in R, to which everything seemed fine with the code and model, but my standard residual plots appear to be non-linear. Why is it that my qq plot and initial plot seem linear but the residual contradicts? Furthermore what does this mean in context to the topic? The topic is on the dependency of strength on body weight. Also, why is it that my r-squared value is high and my Shapiro test fails to reject the null hypothesis but my residual plot is non-linear The data I used was of a linear trend as well, as shown by the initial scatter plot. Context in the answer as well as why this has happened is vital in my understanding so sincerely please do your best I can’t attach more than 2 images so I will attach my dataset and results. Here’s the R script: Residuals: Min -10.531 -4.989 1Q Median 30 Max 1.869 5.267 7.950 Coefficients: Estimate Std. Error t value Pr (>|t|) (Intercept) 42.62755 6.86517 6.209 0.000157 *** 'Body Weight (kg)' 0.62692 0.07461 8.402 1.49e-05 *** Signif. codes: 0 ***** 0.001 **** 0.01 *** 0.05 '.' 0.1 ' ' 1 Residual standard error: 7.077 on 9 degrees of freedom Multiple R-squared: 0.8869, Adjusted R-squared: 0.8744 F-statistic: 70.6 on 1 and 9 DF, p-value: 1.492e-05 > abline (Regression.model, col=4, 1wd=3) > anova (Regression.model) Analysis of Variance Table Response: Bench press standards per class of body weight (kg) - Average Df Sum Sq Mean Sq F value Pr (>F) 'Body Weight (kg) 1 3536.0 3536.0 70.601 1.492e-05 *** Residuals 9 450.8 50.1 Signif. codes: 0 ***** 0.001 **** 0.01 *** 0.05 ' ' 0.1 ' ' 1 > stdres‹- rstandard (Regression.model) > print (stdres) 1 2 3 4 5 6 7 -1.4864868 -1. 0645996 -0.5043759 0.2843252 1.1786018 0. 6513863 1.1083291 11 0.9248828 0.5985440 -0.3245869 -2.0230776 > plot ('Body Weight (kg)', stdres, main="Std residuals versus explanatory variable") > fits‹- fitted (Regression.model) > plot (fits, stdres, main="Std residuals versus fits") > qqnorm (stdres, main="e-l Plot") > galine (stdres) > shapiro. test (stdres) Shapiro-Wilk normality test data: stares W = 0.91857, p-value = 0.3069 Sorry for the mess of it, as I’ve said I can’t attach more than 2 images.
I've had to ask this question multiple times, to which a few had either been copied from the previous answer or from the internet so please, if you can produce a direct and concise answer it would be much appreciated. I have recently produced a linear regression model in R, to which everything seemed fine with the code and model, but my standard residual plots appear to be non-linear. Why is it that my qq plot and initial plot seem linear but the residual contradicts? Furthermore what does this mean in context to the topic? The topic is on the dependency of strength on body weight. Also, why is it that my r-squared value is high and my Shapiro test fails to reject the null hypothesis but my residual plot is non-linear The data I used was of a linear trend as well, as shown by the initial scatter plot. Context in the answer as well as why this has happened is vital in my understanding so sincerely please do your best I can’t attach more than 2 images so I will attach my dataset and results. Here’s the R script: Residuals: Min -10.531 -4.989 1Q Median 30 Max 1.869 5.267 7.950 Coefficients: Estimate Std. Error t value Pr (>|t|) (Intercept) 42.62755 6.86517 6.209 0.000157 *** 'Body Weight (kg)' 0.62692 0.07461 8.402 1.49e-05 *** Signif. codes: 0 ***** 0.001 **** 0.01 *** 0.05 '.' 0.1 ' ' 1 Residual standard error: 7.077 on 9 degrees of freedom Multiple R-squared: 0.8869, Adjusted R-squared: 0.8744 F-statistic: 70.6 on 1 and 9 DF, p-value: 1.492e-05 > abline (Regression.model, col=4, 1wd=3) > anova (Regression.model) Analysis of Variance Table Response: Bench press standards per class of body weight (kg) - Average Df Sum Sq Mean Sq F value Pr (>F) 'Body Weight (kg) 1 3536.0 3536.0 70.601 1.492e-05 *** Residuals 9 450.8 50.1 Signif. codes: 0 ***** 0.001 **** 0.01 *** 0.05 ' ' 0.1 ' ' 1 > stdres‹- rstandard (Regression.model) > print (stdres) 1 2 3 4 5 6 7 -1.4864868 -1. 0645996 -0.5043759 0.2843252 1.1786018 0. 6513863 1.1083291 11 0.9248828 0.5985440 -0.3245869 -2.0230776 > plot ('Body Weight (kg)', stdres, main="Std residuals versus explanatory variable") > fits‹- fitted (Regression.model) > plot (fits, stdres, main="Std residuals versus fits") > qqnorm (stdres, main="e-l Plot") > galine (stdres) > shapiro. test (stdres) Shapiro-Wilk normality test data: stares W = 0.91857, p-value = 0.3069 Sorry for the mess of it, as I’ve said I can’t attach more than 2 images.
MATLAB: An Introduction with Applications
6th Edition
ISBN:9781119256830
Author:Amos Gilat
Publisher:Amos Gilat
Chapter1: Starting With Matlab
Section: Chapter Questions
Problem 1P
Related questions
Question
I've had to ask this question multiple times, to which a few had either been copied from the previous answer or from the internet so please, if you can produce a direct and concise answer it would be much appreciated.
I have recently produced a linear regression model in R, to which everything seemed fine with the code and model, but my standard residual plots appear to be non-linear. Why is it that my qq plot and initial plot seem linear but the residual contradicts? Furthermore what does this mean in context to the topic? The topic is on the dependency of strength on body weight.
Also, why is it that my r-squared value is high and my Shapiro test fails to reject the null hypothesis but my residual plot is non-linear
The data I used was of a linear trend as well, as shown by the initial scatter plot .
Context in the answer as well as why this has happened is vital in my understanding so sincerely please do your best
I can’t attach more than 2 images so I will attach my dataset and results.
Here’s the R script:
Residuals:
Min
-10.531 -4.989
1Q Median
30
Max
1.869 5.267 7.950
Coefficients:
Estimate Std. Error t value Pr (>|t|)
(Intercept)
42.62755
6.86517 6.209 0.000157
***
'Body Weight (kg)' 0.62692 0.07461 8.402 1.49e-05 ***
Signif. codes: 0 ***** 0.001 **** 0.01 *** 0.05 '.' 0.1 ' ' 1
Residual standard error: 7.077 on 9 degrees of freedom
Multiple R-squared: 0.8869, Adjusted R-squared: 0.8744
F-statistic: 70.6 on 1 and
9 DF, p-value: 1.492e-05
> abline (Regression.model, col=4, 1wd=3)
> anova (Regression.model)
Analysis of Variance Table
Response: Bench press standards per class of body weight (kg) - Average
Df Sum Sq Mean Sq F value
Pr (>F)
'Body Weight (kg) 1 3536.0 3536.0 70.601 1.492e-05 ***
Residuals
9
450.8
50.1
Signif. codes: 0 ***** 0.001 **** 0.01 *** 0.05 ' ' 0.1 ' ' 1
> stdres‹- rstandard (Regression.model)
> print (stdres)
1
2
3
4
5
6
7
-1.4864868 -1. 0645996 -0.5043759 0.2843252
1.1786018
0. 6513863
1.1083291
11
0.9248828 0.5985440 -0.3245869 -2.0230776
> plot ('Body Weight (kg)', stdres, main="Std residuals versus explanatory variable")
> fits‹- fitted (Regression.model)
> plot (fits, stdres, main="Std residuals versus fits")
> qqnorm (stdres, main="e-l Plot")
> galine (stdres)
> shapiro. test (stdres)
Shapiro-Wilk normality test
data: stares
W = 0.91857, p-value = 0.3069
Sorry for the mess of it, as I’ve said I can’t attach more than 2 images.

Transcribed Image Text:1
2
3
4
5
6
7
8
9
10
11
Body Weight (kg)
52
56
60
67
75
82
90
100
110
125
145
Bench press standards per class of body weight (kg) - Average
66.0
71.0
77.0
86.5
94.0
101.5
107.0
111.5
115.5
119.0
123.0

Transcribed Image Text:100 110 120
Bench press standards per class of body weight (kg) - Average
Sample Quantiles
06
80
70
0.0 0.5 1.0
-1.0
-2.0
O
O
60
Scatterplot for average
O
80
-1.5 -1.0
0
100
-0.5
Body Weight (kg)
O
Q-Q Plot
O
120
0.0 0.5
Theoretical Quantiles
O
O
1.0
140
O
1.5
O
stdres
0.0 0.5 1.0
stdres
-1.0
-2.0
1.0
0.5
0.0
-0.5
-1.5 -1.0
-2.0
Std residuals versus explanatory variable
O
T
09
o
80
O
O
80
90
O
O
Body Weight (kg)
O
Std residuals versus fits
O
100
100
O
fits
O
120
110
O
120
140
130
O
Expert Solution

This question has been solved!
Explore an expertly crafted, step-by-step solution for a thorough understanding of key concepts.
Step by step
Solved in 4 steps with 1 images

Recommended textbooks for you
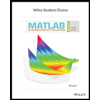
MATLAB: An Introduction with Applications
Statistics
ISBN:
9781119256830
Author:
Amos Gilat
Publisher:
John Wiley & Sons Inc
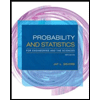
Probability and Statistics for Engineering and th…
Statistics
ISBN:
9781305251809
Author:
Jay L. Devore
Publisher:
Cengage Learning
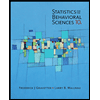
Statistics for The Behavioral Sciences (MindTap C…
Statistics
ISBN:
9781305504912
Author:
Frederick J Gravetter, Larry B. Wallnau
Publisher:
Cengage Learning
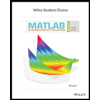
MATLAB: An Introduction with Applications
Statistics
ISBN:
9781119256830
Author:
Amos Gilat
Publisher:
John Wiley & Sons Inc
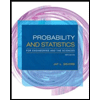
Probability and Statistics for Engineering and th…
Statistics
ISBN:
9781305251809
Author:
Jay L. Devore
Publisher:
Cengage Learning
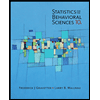
Statistics for The Behavioral Sciences (MindTap C…
Statistics
ISBN:
9781305504912
Author:
Frederick J Gravetter, Larry B. Wallnau
Publisher:
Cengage Learning
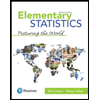
Elementary Statistics: Picturing the World (7th E…
Statistics
ISBN:
9780134683416
Author:
Ron Larson, Betsy Farber
Publisher:
PEARSON
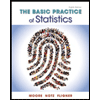
The Basic Practice of Statistics
Statistics
ISBN:
9781319042578
Author:
David S. Moore, William I. Notz, Michael A. Fligner
Publisher:
W. H. Freeman
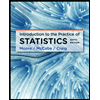
Introduction to the Practice of Statistics
Statistics
ISBN:
9781319013387
Author:
David S. Moore, George P. McCabe, Bruce A. Craig
Publisher:
W. H. Freeman