The Simple Linear Regression model is Y = b0 + b1*X1 + u and the Multiple Linear Regression model with k variables is: Y = b0 + b1*X1 + b2*X2 + ... + bk*Xk + u Y is the dependent variable, the X1, X2, ..., Xk are the explanatory variables, b0 is the intercept, b1, b2, ..., bk are the slope coefficients, and u is the error term, Yhat represents the OLS fitted values, uhat represent the OLS residuals, b0_hat represents the OLS estimated intercept, and b1_hat, b2_hat,..., bk_hat, represent the OLS estimated slope coefficients. QUESTION 19 Suppose your estimated MLR model is: Y_hat = 11 - 0.4*X + 0.01*X2 According to this estimated model, what is the value of X that minimizes Y_hat? It is equal to -20 It is equal to -40 It is equal to 20 It is equal to 40 QUESTION 20 Suppose your estimated MLR model with two explanatory variables, log(X1) and X2, is: Y_hat= 10 – 10*log(X1) + 0.04*X2 Which of the following statements about the interpretation of the coefficient of log(X1) is correct? If X1 increases by 2%, Y is predicted to decrease by approximately 0.20 units, holding X2 constant If X1 increases by 2%, Y is predicted to decrease by approximately 20 units, holding X2 constant If X1 increases by 2 units, Y is predicted to decrease by approximately 20 units, holding X2 constant If X1 increases by 2 units, Y is predicted to decrease by approximately 20%, holding X2 constant QUESTION 21 Suppose your estimated MLR model is: Y_hat = 11 + 0.6*X - 0.01*X2 If X increases by 1 unit from X=60, what will the approximate change in Y_hat be? the change will be approximately equal to 0. an increase of about 34.8 units an increase of about 0.59 units a decrease of about 0.6 units
The Simple Linear Regression model is
Y = b0 + b1*X1 + u
and the Multiple Linear Regression model with k variables is:
Y = b0 + b1*X1 + b2*X2 + ... + bk*Xk + u
Y is the dependent variable, the X1, X2, ..., Xk are the explanatory variables, b0 is the intercept, b1, b2, ..., bk are the slope coefficients, and u is the error term,
Yhat represents the OLS fitted values, uhat represent the OLS residuals, b0_hat represents the OLS estimated intercept, and b1_hat, b2_hat,..., bk_hat, represent the OLS estimated slope coefficients.
QUESTION 19
Suppose your estimated MLR model is:
Y_hat = 11 - 0.4*X + 0.01*X2
According to this estimated model, what is the value of X that minimizes Y_hat?
- It is equal to -20
- It is equal to -40
- It is equal to 20
- It is equal to 40
QUESTION 20
Suppose your estimated MLR model with two explanatory variables, log(X1) and X2, is:
Y_hat= 10 – 10*log(X1) + 0.04*X2
Which of the following statements about the interpretation of the coefficient of log(X1) is correct?
- If X1 increases by 2%, Y is predicted to decrease by approximately 0.20 units, holding X2 constant
- If X1 increases by 2%, Y is predicted to decrease by approximately 20 units, holding X2 constant
- If X1 increases by 2 units, Y is predicted to decrease by approximately 20 units, holding X2 constant
- If X1 increases by 2 units, Y is predicted to decrease by approximately 20%, holding X2 constant
QUESTION 21
Suppose your estimated MLR model is:
Y_hat = 11 + 0.6*X - 0.01*X2
If X increases by 1 unit from X=60, what will the approximate change in Y_hat be?
- the change will be approximately equal to 0.
- an increase of about 34.8 units
- an increase of about 0.59 units
- a decrease of about 0.6 units

Trending now
This is a popular solution!
Step by step
Solved in 3 steps with 3 images

could you help with these too please, the simple linear regression and multiple linear regression are all the same.
QUESTION 5
In the SLR model, suppose the explanatory variable (X1) represents how much a school spends per student (in £1000), and the dependent variable (Y) represents the average test score (in percent) in a standardised test in that school. Suppose you collect a sample of different schools and estimate this model by OLS. Then the estimated intercept b0_hat, represents:
- by how much the average test score (in percent) is predicted to change, if spending per student in a school increases by £1000
- The predicted spending per student for a school, if the average test score in that school is equal to 0%
- the predicted average test score (in percent), for a school that spends £0 per student
- the predicted average test score (in percent) in a school, irrespective of the spending per student in that school
QUESTION 6
Suppose you have the following data:
Y |
X |
-1 |
4 |
-2 |
2 |
1 |
-1 |
4 |
-3 |
3 |
1 |
-3 |
4 |
What is the OLS fitted line?
- Yhat = 1.178 -1.034*X1
- Yhat = -0.845 + 0.333*X1
- Yhat = 1.441 - 0.822*X1
- Yhat = 1.305 - 0.833*X1
QUESTION 14
Suppose that in the model Y=b0+b1*X1+u, we add a variable that is
- It will go up
- It will go down
- We cannot say with the provided information
- It will remain unchanged
QUESTION 15
Suppose you have an MLR model that includes an intercept, with 150 observations and 11 variables. If assumptions MLR1-MLR6 hold, a t-statistic for any of the coefficients in this model follows the t-distribution with degrees of freedom equal to:
- 138
- 11
- 150
- 139
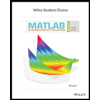
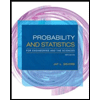
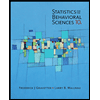
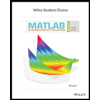
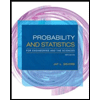
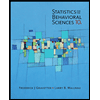
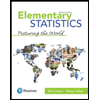
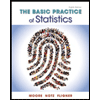
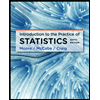