Consider the following regression model Yt = β0 + β1 Ut + β2 Vt + β3 Wt + β4Xt + ∈t , where U, V, W, X and Y are economic variables observed from t = 1, . . . , 75, β0 , . . . , β4 are the model parameters and ∈t is the random disturbance term satisfying the classical assumptions. Ordinary Least Squares (OLS) is used to estimate the parameters, producing the following estimated model: Yt = 1.115 + 0.790*Ut − 0.327*Vt + 0.763*Wt + 0.456*Xt (0.405) (0.178) (0.088) (0.274) (0.017) where standard errors are given in parentheses, the R-squared = 0.941, the Durbin-Watson statistic is DW = 1.907 and the residual sum of squares is RSS = 0.0757. In answering this question, use the 5% level of significance for any hypothesis tests that you are asked to perform, state clearly the null and al- ternative hypotheses that you are testing, the test statistics that you are using and interpret the decisions that you make. (a) Describe the concepts of unbiasedness and efficiency. State the conditions required of regression in order that the OLS estimators of the model parameters possess these properties. (b) Perform the following tests on the parameters of above regression: (i) test whether the parameters β1 , β2 , β3 and β4 are individually statistically significant; (ii) test the overall significance of the regression model; (iii) test whether β4 is statistically equal to 0.5 against whether it is less than 0.5. (c) Suppose you wish to test whether the economic variables U and W have the same impact on Y or if they have different impacts on Y . Express this in terms of an appropriate null and alternative hypothesis and show that if the impacts were the same then the regression model would become: Yt = β0 + β1 Zt + β2 Vt + β4Xt + ∈t , where Zt = (Ut + Wt ). Perform the test, using the information in the following OLS estimated regression: Yt = 1.225 + 0.782*Zt − 0.403*Vt + 0.412*Xt (0.361) (0.147) (0.151) (0.081) where the RSS = 0.0781 and the DW = 2.043.
Consider the following regression model
Yt = β0 + β1 Ut + β2 Vt + β3 Wt + β4Xt + ∈t ,
where U, V, W, X and Y are economic variables observed from t = 1, . . . , 75, β0 , . . . , β4 are the model parameters and ∈t is the random disturbance term satisfying the classical assumptions. Ordinary Least Squares (OLS) is used to estimate the parameters, producing the following estimated model:
Yt = 1.115 + 0.790*Ut − 0.327*Vt + 0.763*Wt + 0.456*Xt
(0.405) (0.178) (0.088) (0.274) (0.017)
where standard errors are given in parentheses, the R-squared = 0.941, the Durbin-Watson statistic is DW = 1.907 and the residual sum of squares is RSS = 0.0757. In answering this question, use the 5% level of significance for any hypothesis tests that you are asked to perform, state clearly the null and al- ternative hypotheses that you are testing, the test statistics that you are using and interpret the decisions that you make.
(a) Describe the concepts of unbiasedness and efficiency. State the conditions required of regression in order that the OLS estimators of the model parameters possess these properties.
(b) Perform the following tests on the parameters of above regression: (i) test whether the parameters β1 , β2 , β3 and β4 are individually statistically significant; (ii) test the overall significance of the regression model; (iii) test whether β4 is statistically equal to 0.5 against whether it is less than 0.5.
(c) Suppose you wish to test whether the economic variables U and W have the same impact on Y or if they have different impacts on Y . Express this in terms of an appropriate null and alternative hypothesis and show that if the impacts were the same then the regression model would become:
Yt = β0 + β1 Zt + β2 Vt + β4Xt + ∈t ,
where Zt = (Ut + Wt ). Perform the test, using the information in the following OLS estimated regression:
Yt = 1.225 + 0.782*Zt − 0.403*Vt + 0.412*Xt
(0.361) (0.147) (0.151) (0.081)
where the RSS = 0.0781 and the DW = 2.043.
(d) What are the consequences of autocorrelated errors on OLS estimators and discuss a way in which this can be resolved? For the model that you have chosen as a result of the test in part (c), perform a test for autocorrelation of the error term.

Trending now
This is a popular solution!
Step by step
Solved in 4 steps

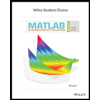
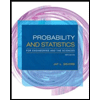
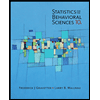
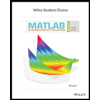
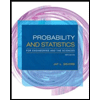
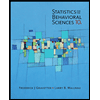
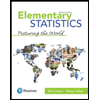
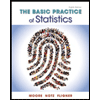
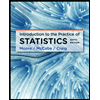