Consider the following medical data set on coronary heart disease in Table 1. The task is to predict the disease of patients based on their clinical features. Table 1: Heart disease data set. [gender: 0 represents Female and 1 represents Male; heart-disease: 0 represents No and 1 represents Yes] heart-disease (class) age 58 58 55 46 54 71 43 34 gender 0 1 1 1 1 0 0 0 C. d. Ratio RBP - (resting blood pressure in mm Hg) 100 114 160 120 122 112 132 118 a) Which attribute type ("level of measurement") best describes the RBP attribute? a. Nominal b. Ordinal Interval C. d. cholesterol (serum cholesterol in mg/dl) 248 318 289 249 286 149 341 210 b) Which attribute type ("level of measurement") best describes the age attribute? a. Nominal b. Ordinal Interval Ratio c) List a possible source (i.e. the cause) of noise, missing data, or errors in this dataset 1 0 0 0 0 1 0 1 d) Select a data cleaning method to address the problem you identified in the previous question. Justify your choice. e) Name a classifier that could be applied to this problem. Briefly outline the steps of the training algorithm for that classifier.
Consider the following medical data set on coronary heart disease in Table 1. The task is to predict the disease of patients based on their clinical features. Table 1: Heart disease data set. [gender: 0 represents Female and 1 represents Male; heart-disease: 0 represents No and 1 represents Yes] heart-disease (class) age 58 58 55 46 54 71 43 34 gender 0 1 1 1 1 0 0 0 C. d. Ratio RBP - (resting blood pressure in mm Hg) 100 114 160 120 122 112 132 118 a) Which attribute type ("level of measurement") best describes the RBP attribute? a. Nominal b. Ordinal Interval C. d. cholesterol (serum cholesterol in mg/dl) 248 318 289 249 286 149 341 210 b) Which attribute type ("level of measurement") best describes the age attribute? a. Nominal b. Ordinal Interval Ratio c) List a possible source (i.e. the cause) of noise, missing data, or errors in this dataset 1 0 0 0 0 1 0 1 d) Select a data cleaning method to address the problem you identified in the previous question. Justify your choice. e) Name a classifier that could be applied to this problem. Briefly outline the steps of the training algorithm for that classifier.
Database System Concepts
7th Edition
ISBN:9780078022159
Author:Abraham Silberschatz Professor, Henry F. Korth, S. Sudarshan
Publisher:Abraham Silberschatz Professor, Henry F. Korth, S. Sudarshan
Chapter1: Introduction
Section: Chapter Questions
Problem 1PE
Related questions
Question
100%
![Consider the following medical data set on coronary heart disease in Table 1. The task is to predict the disease
of patients based on their clinical features.
Table 1: Heart disease data set.
[gender: 0 represents Female and 1 represents Male; heart-disease: 0 represents No and 1 represents Yes]
heart-disease (class)
age
58
58
55
46
54
71
43
34
a.
b.
gender
0
1
1
1
1
0
0
0
a) Which attribute type ("level of measurement") best describes the RBP attribute?
Nominal
Ordinal
Interval
C.
d. Ratio
a.
b.
RBP - (resting blood
pressure in mm Hg)
100
114
160
120
122
112
132
118
cholesterol - (serum
cholesterol in mg/dl)
248
318
289
249
286
149
341
210
b) Which attribute type ("level of measurement") best describes the age attribute?
Nominal
Ordinal
Interval
C.
d. Ratio
c) List a possible source (i.e. the cause) of noise, missing data, or errors in this dataset
1
0
0
0
0
1
0
1
d) Select a data cleaning method to address the problem you identified in the previous question. Justify your
choice.
e) Name a classifier that could be applied to this problem. Briefly outline the steps of the training algorithm
for that classifier.](/v2/_next/image?url=https%3A%2F%2Fcontent.bartleby.com%2Fqna-images%2Fquestion%2F4346761a-0140-452b-a6bd-a6a1421e4117%2F8167cd81-a6de-4687-9ce6-83d0fd29bdf1%2Fyj1u5cc_processed.jpeg&w=3840&q=75)
Transcribed Image Text:Consider the following medical data set on coronary heart disease in Table 1. The task is to predict the disease
of patients based on their clinical features.
Table 1: Heart disease data set.
[gender: 0 represents Female and 1 represents Male; heart-disease: 0 represents No and 1 represents Yes]
heart-disease (class)
age
58
58
55
46
54
71
43
34
a.
b.
gender
0
1
1
1
1
0
0
0
a) Which attribute type ("level of measurement") best describes the RBP attribute?
Nominal
Ordinal
Interval
C.
d. Ratio
a.
b.
RBP - (resting blood
pressure in mm Hg)
100
114
160
120
122
112
132
118
cholesterol - (serum
cholesterol in mg/dl)
248
318
289
249
286
149
341
210
b) Which attribute type ("level of measurement") best describes the age attribute?
Nominal
Ordinal
Interval
C.
d. Ratio
c) List a possible source (i.e. the cause) of noise, missing data, or errors in this dataset
1
0
0
0
0
1
0
1
d) Select a data cleaning method to address the problem you identified in the previous question. Justify your
choice.
e) Name a classifier that could be applied to this problem. Briefly outline the steps of the training algorithm
for that classifier.
Expert Solution

This question has been solved!
Explore an expertly crafted, step-by-step solution for a thorough understanding of key concepts.
Step by step
Solved in 2 steps

Follow-up Questions
Read through expert solutions to related follow-up questions below.
Follow-up Question
Thank you for the answers. I appreciate.
Can you handle this too? Please...
![Consider the following version of the heart disease data set in Table 2. Here the goal is to predict whether
patients have heart disease or not based on chest pain types and locations. Consider a new instance, with
attribute values [chest-pain-type = 2, chest-pain-location = 1]. Using the naïve Bayes classifier, calculate the
predicted probability of having heart disease. Show your work, including all intermediate probabilities that
you needed to calculate. I recommend only calculating the conditional probabilities that are directly needed for
classifying this instance, rather than calculating the full table of conditional probabilities. Also, there is no
need to use Laplace smoothing.
Recall that Bayes' rule is p(x) = ²(c)p(c)
p(x)
chest-pain-type
{1: typical angina; 2: atypical
angina; 3: non-anginal pain}
2
1
1
3
3
2
3
=
p(c)p(c)
Σp(c')p(c')'
and naïve Bayes assumes p(x) = [₁ p(x₁|c)
Table 2: Another heart disease data set
chest-pain-location
{1: substernal; 0: otherwise}
1
0
1
0
1
1
0
heart-disease (class)
{0: No; 1: Yes}
0
0
1
0
1
1
1](https://content.bartleby.com/qna-images/question/4346761a-0140-452b-a6bd-a6a1421e4117/8ec32935-34ab-4939-a1c3-d73d19e07c85/cn3b4wn_thumbnail.jpeg)
Transcribed Image Text:Consider the following version of the heart disease data set in Table 2. Here the goal is to predict whether
patients have heart disease or not based on chest pain types and locations. Consider a new instance, with
attribute values [chest-pain-type = 2, chest-pain-location = 1]. Using the naïve Bayes classifier, calculate the
predicted probability of having heart disease. Show your work, including all intermediate probabilities that
you needed to calculate. I recommend only calculating the conditional probabilities that are directly needed for
classifying this instance, rather than calculating the full table of conditional probabilities. Also, there is no
need to use Laplace smoothing.
Recall that Bayes' rule is p(x) = ²(c)p(c)
p(x)
chest-pain-type
{1: typical angina; 2: atypical
angina; 3: non-anginal pain}
2
1
1
3
3
2
3
=
p(c)p(c)
Σp(c')p(c')'
and naïve Bayes assumes p(x) = [₁ p(x₁|c)
Table 2: Another heart disease data set
chest-pain-location
{1: substernal; 0: otherwise}
1
0
1
0
1
1
0
heart-disease (class)
{0: No; 1: Yes}
0
0
1
0
1
1
1
Solution
Knowledge Booster
Learn more about
Need a deep-dive on the concept behind this application? Look no further. Learn more about this topic, computer-science and related others by exploring similar questions and additional content below.Recommended textbooks for you
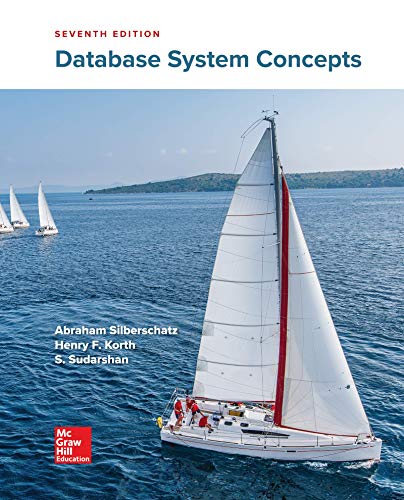
Database System Concepts
Computer Science
ISBN:
9780078022159
Author:
Abraham Silberschatz Professor, Henry F. Korth, S. Sudarshan
Publisher:
McGraw-Hill Education
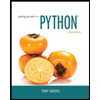
Starting Out with Python (4th Edition)
Computer Science
ISBN:
9780134444321
Author:
Tony Gaddis
Publisher:
PEARSON
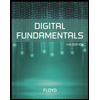
Digital Fundamentals (11th Edition)
Computer Science
ISBN:
9780132737968
Author:
Thomas L. Floyd
Publisher:
PEARSON
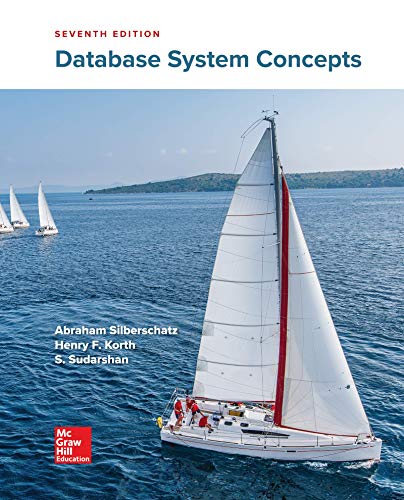
Database System Concepts
Computer Science
ISBN:
9780078022159
Author:
Abraham Silberschatz Professor, Henry F. Korth, S. Sudarshan
Publisher:
McGraw-Hill Education
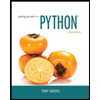
Starting Out with Python (4th Edition)
Computer Science
ISBN:
9780134444321
Author:
Tony Gaddis
Publisher:
PEARSON
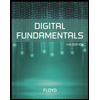
Digital Fundamentals (11th Edition)
Computer Science
ISBN:
9780132737968
Author:
Thomas L. Floyd
Publisher:
PEARSON
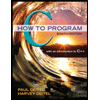
C How to Program (8th Edition)
Computer Science
ISBN:
9780133976892
Author:
Paul J. Deitel, Harvey Deitel
Publisher:
PEARSON
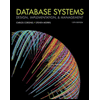
Database Systems: Design, Implementation, & Manag…
Computer Science
ISBN:
9781337627900
Author:
Carlos Coronel, Steven Morris
Publisher:
Cengage Learning
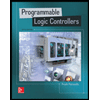
Programmable Logic Controllers
Computer Science
ISBN:
9780073373843
Author:
Frank D. Petruzella
Publisher:
McGraw-Hill Education