In python, for a sample data with 4 columns and 60 rows how do you find the parameters for the regression with the feature map (see attached) where we consider the loss function to be the square of residuals. Once this is done, how do you compute the empirical risk? I've attached some of the data below, it would be sufficient to see how you get results for the question using the above dataset. 1 14 25 620 -1 69 29 625 0 83 27 850 0 28 25 1315 1 41 25 2120 -1 153 31 1315 0 55 25 2600 0 55 31 490 1 69 25 3110 1 83 25 3535
In python, for a sample data with 4 columns and 60 rows how do you find the parameters for the regression with the feature map (see attached) where we consider the loss function to be the square of residuals. Once this is done, how do you compute the empirical risk? I've attached some of the data below, it would be sufficient to see how you get results for the question using the above dataset. 1 14 25 620 -1 69 29 625 0 83 27 850 0 28 25 1315 1 41 25 2120 -1 153 31 1315 0 55 25 2600 0 55 31 490 1 69 25 3110 1 83 25 3535
Computer Networking: A Top-Down Approach (7th Edition)
7th Edition
ISBN:9780133594140
Author:James Kurose, Keith Ross
Publisher:James Kurose, Keith Ross
Chapter1: Computer Networks And The Internet
Section: Chapter Questions
Problem R1RQ: What is the difference between a host and an end system? List several different types of end...
Related questions
Question
In python, for a sample data with 4 columns and 60 rows how do you find the parameters for the regression with the feature map (see attached) where we consider the loss function to be the square of residuals. Once this is done, how do you compute the empirical risk? I've attached some of the data below, it would be sufficient to see how you get results for the question using the above dataset.
1 | 14 | 25 | 620 |
-1 | 69 | 29 | 625 |
0 | 83 | 27 | 850 |
0 | 28 | 25 | 1315 |
1 | 41 | 25 | 2120 |
-1 | 153 | 31 | 1315 |
0 | 55 | 25 | 2600 |
0 | 55 | 31 | 490 |
1 | 69 | 25 | 3110 |
1 | 83 | 25 | 3535 |
![1
2
= [1
X₁ X₂ X1X₂].
T2](/v2/_next/image?url=https%3A%2F%2Fcontent.bartleby.com%2Fqna-images%2Fquestion%2F0072889a-26e4-4c78-b441-61b46ee7eda8%2F7a68652a-8486-421b-9600-85017e32cc89%2Fydbjgim_processed.png&w=3840&q=75)
Transcribed Image Text:1
2
= [1
X₁ X₂ X1X₂].
T2
Expert Solution

This question has been solved!
Explore an expertly crafted, step-by-step solution for a thorough understanding of key concepts.
Step 1: Introduction
VIEWStep 2: Define the feature map function:
VIEWStep 3: Apply the feature map to the input data:
VIEWStep 4: Define the output values as:
VIEWStep 5: Fit a linear regression model using the feature mapped data: scss
VIEWStep 6: To compute the empirical risk, we can use the mean squared error (MSE) as the loss function.
VIEWStep 7: Here's the complete code:
VIEWStep 8: Code Image
VIEWSolution
VIEWStep by step
Solved in 9 steps with 7 images

Recommended textbooks for you
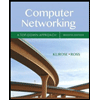
Computer Networking: A Top-Down Approach (7th Edi…
Computer Engineering
ISBN:
9780133594140
Author:
James Kurose, Keith Ross
Publisher:
PEARSON
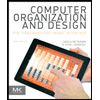
Computer Organization and Design MIPS Edition, Fi…
Computer Engineering
ISBN:
9780124077263
Author:
David A. Patterson, John L. Hennessy
Publisher:
Elsevier Science
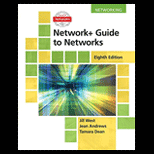
Network+ Guide to Networks (MindTap Course List)
Computer Engineering
ISBN:
9781337569330
Author:
Jill West, Tamara Dean, Jean Andrews
Publisher:
Cengage Learning
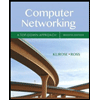
Computer Networking: A Top-Down Approach (7th Edi…
Computer Engineering
ISBN:
9780133594140
Author:
James Kurose, Keith Ross
Publisher:
PEARSON
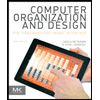
Computer Organization and Design MIPS Edition, Fi…
Computer Engineering
ISBN:
9780124077263
Author:
David A. Patterson, John L. Hennessy
Publisher:
Elsevier Science
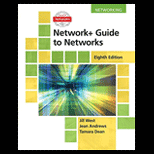
Network+ Guide to Networks (MindTap Course List)
Computer Engineering
ISBN:
9781337569330
Author:
Jill West, Tamara Dean, Jean Andrews
Publisher:
Cengage Learning
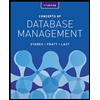
Concepts of Database Management
Computer Engineering
ISBN:
9781337093422
Author:
Joy L. Starks, Philip J. Pratt, Mary Z. Last
Publisher:
Cengage Learning
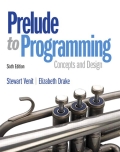
Prelude to Programming
Computer Engineering
ISBN:
9780133750423
Author:
VENIT, Stewart
Publisher:
Pearson Education
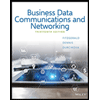
Sc Business Data Communications and Networking, T…
Computer Engineering
ISBN:
9781119368830
Author:
FITZGERALD
Publisher:
WILEY