For this question, create a new column in the dataset where total rainfall is just the sum of our three separate rainfall variables. (a) plot crop yield vs. time. Does yield appear to be stationary? Why or why not? (b) plot total rainfall vs. time. Does total rainfall appear to be stationary? Why or why not? (c) plot the first-difference of crop yield vs. time. Does this series appear to be stationary? Why or why not? (d) formally test whether crop yield, rainfall and the first-difference of crop yield are stationary using the appropriate test. Be sure to do all parts of the hypothesis tests. After these tests, what can you say the order of integration is for each of the variables? (e) estimate a model where yield is a function of rainfall and time. You do not have to worry about the time variable being stationary or not, but the other two must be stationary (you might need to difference one or both of them to make it stationary). Fully report your results. (f) test your model for autocorrelation using both a bounds test and an LM test. Be sure to do all parts of the hypothesis test. Dataset: Yield Time Rg Rd Rf 1.3508 1 1.708 1.475 0.916 0.9476 2 1.544 1.552 0.478 1.2569 3 1.561 1.692 1.005 0.9142 4 2.668 1.476 0.646 1.1022 5 1.163 2.103 0.382 1.1897 6 1.506 3.207 1.322 1.0689 7 2.934 1.204 0.691 0.9007 8 2.624 1.067 0.525 1.2231 9 1.311 2.852 0.496 1.2434 10 1.381 1.078 0.521 1.1427 11 1.787 1.777 0.681 0.7998 12 1.067 1.736 0.395 0.8468 13 2.452 1.386 0.897 0.8938 14 2.81 2.56 0.727 0.9006 15 1.904 3.19 0.63 1.0955 16 1.875 1.779 0.991 0.988 17 1.205 1.527 1.088 0.9408 18 2.664 1.943 0.32 0.8939 19 2.195 1.594 1.004 0.3629 20 1.175 0.756 0.121 1.7111 21 2.025 0.901 0.751 1.5152 22 0.793 1.089 1.415 1.5135 23 0.988 1.788 0.38 1.8519 24 1.782 1.7 1.362 1.2963 25 1.944 1.996 0.636 1.5072 26 1.114 2.076 1.074 1.5867 27 1.058 1.253 0.78 1.3538 28 1.02 1.484 0.76 1.6757 29 1.488 1.48 0.628 1.475 30 1.37 1.172 0.204 1.3289 31 1.192 1.294 0.65 1.28 32 3.158 1.662 0.499 1.5357 33 1.419 1.306 0.606 1.2079 34 1.896 1.87 0.826 1.6154 35 1.514 1.22 0.652 1.6497 36 0.742 1.558 0.456 1.6914 37 1.836 1.676 0.339 2.1408 38 1.464 1.668 0.52 2.3132 39 1.522 1.11 0.77 2.2897 40 0.9 2.076 0.804 2.0983 41 1.992 1.705 0.76 1.9555 42 1.63 1.86 0.906 1.8192 43 1.298 1.558 0.89 2.3881 44 1.939 1.236 0.476 2.2106 45 2.009 2.017 0.87 2.4475 46 1.333 2.307 1.157 2.3959 47 0.892 1.634 0.79 2.9669 48 1.376 1.358 0.656
For this question, create a new column in the dataset where total rainfall is just the sum of our three separate rainfall variables.
(a) plot crop yield vs. time. Does yield appear to be stationary? Why or why not?
(b) plot total rainfall vs. time. Does total rainfall appear to be stationary? Why or why not?
(c) plot the first-difference of crop yield vs. time. Does this series appear to be stationary? Why or why not?
(d) formally test whether crop yield, rainfall and the first-difference of crop yield are stationary using the appropriate test. Be sure to do all parts of the hypothesis tests. After these tests, what can you say the order of integration is for each of the variables?
(e) estimate a model where yield is a function of rainfall and time. You do not have to worry about the time variable being stationary or not, but the other two must be stationary (you might need to difference one or both of them to make it stationary). Fully report your results.
(f) test your model for autocorrelation using both a bounds test and an LM test. Be sure to do all parts of the hypothesis test.
Dataset:
Yield | Time | Rg | Rd | Rf |
1.3508 | 1 | 1.708 | 1.475 | 0.916 |
0.9476 | 2 | 1.544 | 1.552 | 0.478 |
1.2569 | 3 | 1.561 | 1.692 | 1.005 |
0.9142 | 4 | 2.668 | 1.476 | 0.646 |
1.1022 | 5 | 1.163 | 2.103 | 0.382 |
1.1897 | 6 | 1.506 | 3.207 | 1.322 |
1.0689 | 7 | 2.934 | 1.204 | 0.691 |
0.9007 | 8 | 2.624 | 1.067 | 0.525 |
1.2231 | 9 | 1.311 | 2.852 | 0.496 |
1.2434 | 10 | 1.381 | 1.078 | 0.521 |
1.1427 | 11 | 1.787 | 1.777 | 0.681 |
0.7998 | 12 | 1.067 | 1.736 | 0.395 |
0.8468 | 13 | 2.452 | 1.386 | 0.897 |
0.8938 | 14 | 2.81 | 2.56 | 0.727 |
0.9006 | 15 | 1.904 | 3.19 | 0.63 |
1.0955 | 16 | 1.875 | 1.779 | 0.991 |
0.988 | 17 | 1.205 | 1.527 | 1.088 |
0.9408 | 18 | 2.664 | 1.943 | 0.32 |
0.8939 | 19 | 2.195 | 1.594 | 1.004 |
0.3629 | 20 | 1.175 | 0.756 | 0.121 |
1.7111 | 21 | 2.025 | 0.901 | 0.751 |
1.5152 | 22 | 0.793 | 1.089 | 1.415 |
1.5135 | 23 | 0.988 | 1.788 | 0.38 |
1.8519 | 24 | 1.782 | 1.7 | 1.362 |
1.2963 | 25 | 1.944 | 1.996 | 0.636 |
1.5072 | 26 | 1.114 | 2.076 | 1.074 |
1.5867 | 27 | 1.058 | 1.253 | 0.78 |
1.3538 | 28 | 1.02 | 1.484 | 0.76 |
1.6757 | 29 | 1.488 | 1.48 | 0.628 |
1.475 | 30 | 1.37 | 1.172 | 0.204 |
1.3289 | 31 | 1.192 | 1.294 | 0.65 |
1.28 | 32 | 3.158 | 1.662 | 0.499 |
1.5357 | 33 | 1.419 | 1.306 | 0.606 |
1.2079 | 34 | 1.896 | 1.87 | 0.826 |
1.6154 | 35 | 1.514 | 1.22 | 0.652 |
1.6497 | 36 | 0.742 | 1.558 | 0.456 |
1.6914 | 37 | 1.836 | 1.676 | 0.339 |
2.1408 | 38 | 1.464 | 1.668 | 0.52 |
2.3132 | 39 | 1.522 | 1.11 | 0.77 |
2.2897 | 40 | 0.9 | 2.076 | 0.804 |
2.0983 | 41 | 1.992 | 1.705 | 0.76 |
1.9555 | 42 | 1.63 | 1.86 | 0.906 |
1.8192 | 43 | 1.298 | 1.558 | 0.89 |
2.3881 | 44 | 1.939 | 1.236 | 0.476 |
2.2106 | 45 | 2.009 | 2.017 | 0.87 |
2.4475 | 46 | 1.333 | 2.307 | 1.157 |
2.3959 | 47 | 0.892 | 1.634 | 0.79 |
2.9669 | 48 | 1.376 | 1.358 | 0.656 |

Step by step
Solved in 3 steps

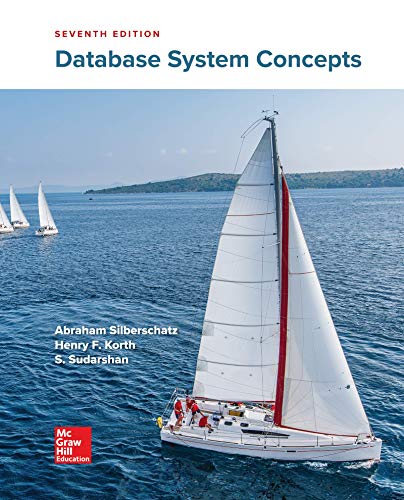
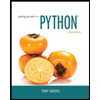
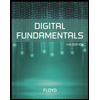
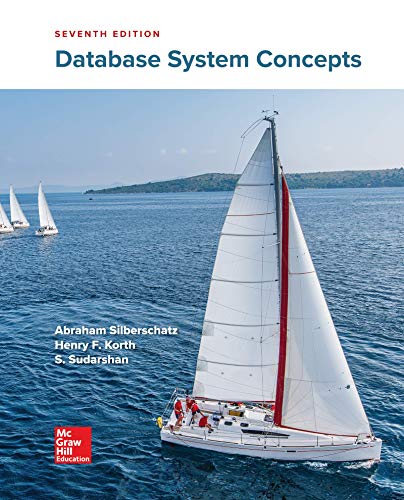
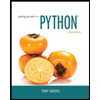
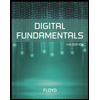
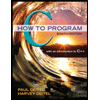
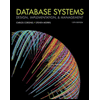
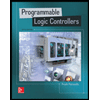