(a) Suppose that fe is a probability mass function i.e. the data is discrete. Prove in this special case that if equation (1) holds then the distribution of X doesn't depend on once you condition on T(X) = t. (b) Prove that if T is a sufficient statistic for the model then the score function only involves the data through the sufficient statistic. (c) Use the answer in the previous part to explain why if (R1)-(R4) hold and T is a sufficient statistic then the MLE only depends on the sufficient statistic and no other function of the data. (d) Suppose that T(X) is a sufficient statistic for some statistical model with feo for some unknown o. Assume further that X is a discrete RV. X
(a) Suppose that fe is a probability mass function i.e. the data is discrete. Prove in this special case that if equation (1) holds then the distribution of X doesn't depend on once you condition on T(X) = t. (b) Prove that if T is a sufficient statistic for the model then the score function only involves the data through the sufficient statistic. (c) Use the answer in the previous part to explain why if (R1)-(R4) hold and T is a sufficient statistic then the MLE only depends on the sufficient statistic and no other function of the data. (d) Suppose that T(X) is a sufficient statistic for some statistical model with feo for some unknown o. Assume further that X is a discrete RV. X
MATLAB: An Introduction with Applications
6th Edition
ISBN:9781119256830
Author:Amos Gilat
Publisher:Amos Gilat
Chapter1: Starting With Matlab
Section: Chapter Questions
Problem 1P
Related questions
Question

Transcribed Image Text:3. Warning: This question is HARDER so expect to spend a little longer on this
one.
Let X = (X₁,..., Xn) be an iid sample with Xi~ foo. We have seen in lectures
that if we have a statistic T(X) of the data instead of the full data then
IT(X) (00) ≤ Ix (00)
A statistic is a summary of the data and so intuitively you can lose information
about the unknown model parameters if you get given the statistic instead of
the full data. However, this is not always the case.
Definition 0.1 (Sufficient Statistic). A statistic T is said to be sufficient for a
statistical model {fe : 0 } of X if the conditional distribution of X given
T = t is independent of 0 for all t.
What this means is that once you know the value of the statistic the distribution
of X has no aspect that any longer depends on 0.
What this really means is revealed in the following Theorem:
Theorem 0.1 (Factorization Criterion). Let P = {fe : 0 € O} be a statistical
model. A statistic T is sufficient for P if and only if there exists non-negative
functions ge() and h() such that
fo(x) = go(T(x))h(x)
(1)
Thus this is saying that the bit of the distribution that involves is interlocked
with the sufficient statistic T and ONLY T (which could in many exampled be
multi dimensional!).
(a) Suppose that fe is a probability mass function i.e. the data is discrete.
Prove in this special case that if equation (1) holds then the distribution
of X doesn't depend on once you condition on T(X) =
= t.
(b) Prove that if T is a sufficient statistic for the model then the score function
only involves the data through the sufficient statistic.
(c) Use the answer in the previous part to explain why if (R1)-(R4) hold and T
is a sufficient statistic then the MLE only depends on the sufficient statistic
and no other function of the data.
(d) Suppose that T(X) is a sufficient statistic for some statistical model with
X foo for some unknown o. Assume further that X is a discrete RV.
Prove that
IT(x) (00) = Ix (00)
Note: This is significant because it shows that we lose no information
about o by only being told the sufficient statistic.
(e) Show that 1X₂ is a sufficient statistic for the setting in Question 1.
(f) Find a Sufficient Statistic in the setting of Question 2. Hint: This will be
2 dimensional.
Expert Solution

This question has been solved!
Explore an expertly crafted, step-by-step solution for a thorough understanding of key concepts.
Step 1: Define sufficient statistic
VIEWStep 2: Prove independence for PMF
VIEWStep 3: Show that the score function involves data only through the sufficient statistic T(X)
VIEWStep 4: Explain why MLE only depends on the sufficient statistic
VIEWStep 5: Show that full data Fisher information is equal to sufficient statistc Fisher information
VIEWSolution
VIEWStep by step
Solved in 6 steps with 7 images

Recommended textbooks for you
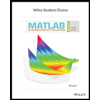
MATLAB: An Introduction with Applications
Statistics
ISBN:
9781119256830
Author:
Amos Gilat
Publisher:
John Wiley & Sons Inc
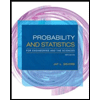
Probability and Statistics for Engineering and th…
Statistics
ISBN:
9781305251809
Author:
Jay L. Devore
Publisher:
Cengage Learning
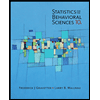
Statistics for The Behavioral Sciences (MindTap C…
Statistics
ISBN:
9781305504912
Author:
Frederick J Gravetter, Larry B. Wallnau
Publisher:
Cengage Learning
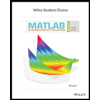
MATLAB: An Introduction with Applications
Statistics
ISBN:
9781119256830
Author:
Amos Gilat
Publisher:
John Wiley & Sons Inc
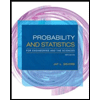
Probability and Statistics for Engineering and th…
Statistics
ISBN:
9781305251809
Author:
Jay L. Devore
Publisher:
Cengage Learning
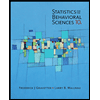
Statistics for The Behavioral Sciences (MindTap C…
Statistics
ISBN:
9781305504912
Author:
Frederick J Gravetter, Larry B. Wallnau
Publisher:
Cengage Learning
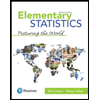
Elementary Statistics: Picturing the World (7th E…
Statistics
ISBN:
9780134683416
Author:
Ron Larson, Betsy Farber
Publisher:
PEARSON
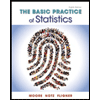
The Basic Practice of Statistics
Statistics
ISBN:
9781319042578
Author:
David S. Moore, William I. Notz, Michael A. Fligner
Publisher:
W. H. Freeman
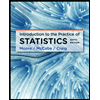
Introduction to the Practice of Statistics
Statistics
ISBN:
9781319013387
Author:
David S. Moore, George P. McCabe, Bruce A. Craig
Publisher:
W. H. Freeman