A sales manager collected the following data on x = years of experience and y = annual sales ($1,000s). The estimated regression equation for these data is ý = 80 + 4x. %3D Years of Experience Annual Sales Salesperson ($1,000s) 1 80 2 97 3 4 97 4 4. 102 5 103 8 101 7 10 119 10 118 11 127 10 13 136 (a) Compute the residuals. Years of Experience Annual Sales Residuals ($1,000s) 1 80 97 4 97 4 102 6 103 8 101 10 119 10 118 11 127 13 136
A sales manager collected the following data on x = years of experience and y = annual sales ($1,000s). The estimated regression equation for these data is ý = 80 + 4x. %3D Years of Experience Annual Sales Salesperson ($1,000s) 1 80 2 97 3 4 97 4 4. 102 5 103 8 101 7 10 119 10 118 11 127 10 13 136 (a) Compute the residuals. Years of Experience Annual Sales Residuals ($1,000s) 1 80 97 4 97 4 102 6 103 8 101 10 119 10 118 11 127 13 136
MATLAB: An Introduction with Applications
6th Edition
ISBN:9781119256830
Author:Amos Gilat
Publisher:Amos Gilat
Chapter1: Starting With Matlab
Section: Chapter Questions
Problem 1P
Related questions
Question

Transcribed Image Text:### Residual Plot Analysis
#### Residual Plots Description
The image displays four residual plots, each representing residuals plotted against "Years of Experience." The y-axis is labeled "Residuals," ranging from -16 to 16, while the x-axis is labeled "Years of Experience," spanning from 0 to 14.
1. **Plot A (First Plot)**
- Residuals appear scattered around the horizontal axis with no clear pattern.
2. **Plot B (Second Plot)**
- Residuals exhibit a slight upward funnel shape from left to right.
3. **Plot C (Third Plot)**
- Residuals seem randomly scattered, showing a slightly uniform horizontal band.
4. **Plot D (Fourth Plot)**
- Residuals depict an apparent downward funnel pattern.
#### Evaluation of Error Term Assumptions
The question associated with the plots evaluates whether the assumptions about error terms seem reasonable based on the residual patterns:
- **Option 1**: The plot suggests curvature in the residuals, indicating that the error term assumptions appear reasonable.
- **Option 2**: The plot suggests a funnel pattern in the residuals, indicating that the error term assumptions appear reasonable.
- **Option 3**: The plot suggests a generally horizontal band of residual points, indicating that the error term assumptions appear reasonable.
- **Option 4**: The plot suggests a funnel pattern in the residuals, indicating that the error term assumptions do not appear reasonable.
- **Option 5**: The plot suggests a generally horizontal band of residual points, indicating that the error term assumptions do not appear reasonable.
These options guide the interpretation of the residual plots, focusing on the presence of patterns like curvature or funnel shapes, which affect the validity of the error term assumptions in regression analysis.

Transcribed Image Text:A sales manager collected the following data on \( x = \) years of experience and \( y = \) annual sales (\$1,000s). The estimated regression equation for these data is \( \hat{y} = 80 + 4x \).
| Salesperson | Years of Experience | Annual Sales (\$1,000s) |
|-------------|---------------------|-------------------------|
| 1 | 1 | 80 |
| 2 | 3 | 97 |
| 3 | 4 | 97 |
| 4 | 4 | 102 |
| 5 | 6 | 103 |
| 6 | 8 | 101 |
| 7 | 10 | 119 |
| 8 | 10 | 118 |
| 9 | 11 | 127 |
| 10 | 13 | 136 |
**(a) Compute the residuals.**
| Years of Experience | Annual Sales (\$1,000s) | Residuals |
|---------------------|-------------------------|-----------|
| 1 | 80 | |
| 3 | 97 | |
| 4 | 97 | |
| 4 | 102 | |
| 6 | 103 | |
| 8 | 101 | |
| 10 | 119 | |
| 10 | 118 | |
| 11 | 127 | |
| 13 | 136 | |
**Explanation:**
The table above shows data collected by a sales manager, including years of experience and corresponding annual sales. The estimated regression equation \( \hat{y} = 80 + 4x \) is used to predict annual sales based on years of experience (x). The task is to compute the residuals, which are the differences between the observed sales and the predicted sales from the regression equation. Residuals help in assessing the fit of the regression model.
Expert Solution

This question has been solved!
Explore an expertly crafted, step-by-step solution for a thorough understanding of key concepts.
This is a popular solution!
Trending now
This is a popular solution!
Step by step
Solved in 4 steps with 1 images

Recommended textbooks for you
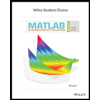
MATLAB: An Introduction with Applications
Statistics
ISBN:
9781119256830
Author:
Amos Gilat
Publisher:
John Wiley & Sons Inc
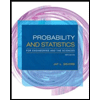
Probability and Statistics for Engineering and th…
Statistics
ISBN:
9781305251809
Author:
Jay L. Devore
Publisher:
Cengage Learning
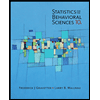
Statistics for The Behavioral Sciences (MindTap C…
Statistics
ISBN:
9781305504912
Author:
Frederick J Gravetter, Larry B. Wallnau
Publisher:
Cengage Learning
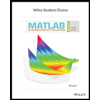
MATLAB: An Introduction with Applications
Statistics
ISBN:
9781119256830
Author:
Amos Gilat
Publisher:
John Wiley & Sons Inc
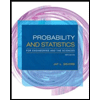
Probability and Statistics for Engineering and th…
Statistics
ISBN:
9781305251809
Author:
Jay L. Devore
Publisher:
Cengage Learning
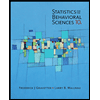
Statistics for The Behavioral Sciences (MindTap C…
Statistics
ISBN:
9781305504912
Author:
Frederick J Gravetter, Larry B. Wallnau
Publisher:
Cengage Learning
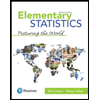
Elementary Statistics: Picturing the World (7th E…
Statistics
ISBN:
9780134683416
Author:
Ron Larson, Betsy Farber
Publisher:
PEARSON
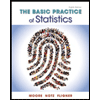
The Basic Practice of Statistics
Statistics
ISBN:
9781319042578
Author:
David S. Moore, William I. Notz, Michael A. Fligner
Publisher:
W. H. Freeman
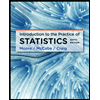
Introduction to the Practice of Statistics
Statistics
ISBN:
9781319013387
Author:
David S. Moore, George P. McCabe, Bruce A. Craig
Publisher:
W. H. Freeman