a production process, the time (in minutes) taken (run time) for a production run and the number of items produced (run size) for 15 randomly selected orders are analyzed using Minitab statistical software. The Minitab output is as follows: Regression Analysis: Run time versus Run size Analysis of Variance Source DF Adj SS Adj MS F-Value Critical value Regression 1 8737.1 8737.1 29.35 ……… Error 13 3870.5 297.7 Total 14 12607.6 Model Summary S R-sq R-sq(adj) R-sq(pred) 17.2549 ……. 66.94% 61.32% Coefficients Term Coef SE Coef T-Value P-Value VIF Constant 148.4 11.3 13.13 0.000 Run size 0.2627 0.0485 ……. ……. 1.00 1- Write down the least square regression equation to predict the run time for run size. 2- Interpret the coefficients of the fitted model. 3- At 5% significance level, test if run size is a good predictor for run time.
In a production process, the time (in minutes) taken (run time) for a production run and the number of items produced (run size) for 15 randomly selected orders are analyzed using Minitab statistical software. The Minitab output is as follows:
Analysis of Variance
Source |
DF |
Adj SS |
Adj MS |
F-Value |
Critical value |
Regression |
1 |
8737.1 |
8737.1 |
29.35 |
……… |
Error |
13 |
3870.5 |
297.7 |
|
|
Total |
14 |
12607.6 |
|
|
|
Model Summary
S |
R-sq |
R-sq(adj) |
R-sq(pred) |
17.2549 |
……. |
66.94% |
61.32% |
Coefficients
Term |
Coef |
SE Coef |
T-Value |
P-Value |
VIF |
||
Constant |
148.4 |
11.3 |
13.13 |
0.000 |
|
||
Run size |
0.2627 |
0.0485 |
……. |
……. |
1.00 |
||
|
|||||||
1- Write down the least square regression equation to predict the run time for run size.
2- Interpret the coefficients of the fitted model.
3- At 5% significance level, test if run size is a good predictor for run time.

Solution:
n= 15 observation.
Step by step
Solved in 2 steps

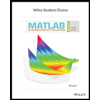
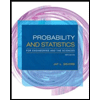
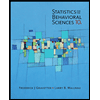
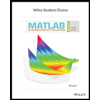
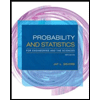
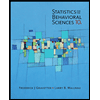
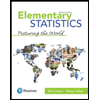
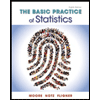
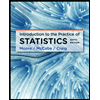