A justification for job training programs is that they improve worker productivity. Suppose that you are asked to evaluate whether more job training makes workers more productive. However, rather than having data on individual workers, you have access to data on manufacturing firms in the US. In particular, for each firm, you have information on hours of average job training per worker (training) and number of items produced per worker hour (output). You can assume for this question that these firms are all producing the same items. The data looks like the following: Average hours of training per year Firm 1 Firm 2 Firm 3 Firm 4 ...Firm 500 16 12 24 20 Number of items produced per worker hour 1.7 1 1.6 2.9 2.4 1. Carefully state the ceteris paribus assumption for analyzing the data in this way. 2. Does it seem likely that a firm's decision to train its workers will be independent of worker characteristics? What are some of those measurable and unmeasurable worker characteristics? 3. Name a factor other than worker characteristics that can affect worker productivity. 4. You collect data on hours of job training per worker (training) and number of items produced per worker hour (output) for a sample of 500 manufacturing firms in the US. You use this data to estimate the following regression equation by OLS: output = po + Bitraining + u. You obtain the following sample regression function: output = 1.2 + 0.25 training. (a) Which assumption about the error term do we need to make to be able to give a causal "ceteris paribus" interpretation to f₁? Write out the assumption in the context of this specific regression model. (b) Interpret the estimates of the intercept (Bo) and slope (ß 1) in this regression. (c) Are you worried about omitted variable bias in this regression? If so, would you expect 1 to be upward or downward biased in the above regression? Explain your answer giving at least one example of a potential omitted variable and discussing their effect on ß^ 1.
A justification for job training programs is that they improve worker productivity. Suppose that you are asked to evaluate whether more job training makes workers more productive. However, rather than having data on individual workers, you have access to data on manufacturing firms in the US. In particular, for each firm, you have information on hours of average job training per worker (training) and number of items produced per worker hour (output). You can assume for this question that these firms are all producing the same items. The data looks like the following: Average hours of training per year Firm 1 Firm 2 Firm 3 Firm 4 ...Firm 500 16 12 24 20 Number of items produced per worker hour 1.7 1 1.6 2.9 2.4 1. Carefully state the ceteris paribus assumption for analyzing the data in this way. 2. Does it seem likely that a firm's decision to train its workers will be independent of worker characteristics? What are some of those measurable and unmeasurable worker characteristics? 3. Name a factor other than worker characteristics that can affect worker productivity. 4. You collect data on hours of job training per worker (training) and number of items produced per worker hour (output) for a sample of 500 manufacturing firms in the US. You use this data to estimate the following regression equation by OLS: output = po + Bitraining + u. You obtain the following sample regression function: output = 1.2 + 0.25 training. (a) Which assumption about the error term do we need to make to be able to give a causal "ceteris paribus" interpretation to f₁? Write out the assumption in the context of this specific regression model. (b) Interpret the estimates of the intercept (Bo) and slope (ß 1) in this regression. (c) Are you worried about omitted variable bias in this regression? If so, would you expect 1 to be upward or downward biased in the above regression? Explain your answer giving at least one example of a potential omitted variable and discussing their effect on ß^ 1.
Chapter1: Making Economics Decisions
Section: Chapter Questions
Problem 1QTC
Related questions
Question

Transcribed Image Text:A justification for job training programs is that they improve worker productivity. Suppose
that you are asked to evaluate whether more job training makes workers more productive.
However, rather than having data on individual workers, you have access to data on
manufacturing firms in the US. In particular, for each firm, you have information on hours of
average job training per worker (training) and number of items produced per worker hour
(output). You can assume for this question that these firms are all producing the same items.
The data looks like the following:
Average hours of training per year
Firm 1
Firm 2
Firm 3
Firm 4
...Firm 500
16
12
24
20
Number of items produced per worker hour
1.7
1
1.6
2.9
2.4
1. Carefully state the ceteris paribus assumption for analyzing the data in this way.
2. Does it seem likely that a firm's decision to train its workers will be independent of
worker characteristics? What are some of those measurable and unmeasurable worker
characteristics?
3. Name a factor other than worker characteristics that can affect worker productivity.
4. You collect data on hours of job training per worker (training) and number of items
produced per worker hour (output) for a sample of 500 manufacturing firms in the US.
You use this data to estimate the following regression equation by OLS: output = po +
Bitraining + u. You obtain the following sample regression function: output = 1.2 +
0.25 training.
(a) Which assumption about the error term do we need to make to be able to give a
causal "ceteris paribus" interpretation to f₁? Write out the assumption in the context
of this specific regression model.
(b) Interpret the estimates of the intercept (Bo) and slope (ß 1) in this regression.
(c) Are you worried about omitted variable bias in this regression? If so, would you
expect 1 to be upward or downward biased in the above regression? Explain your
answer giving at least one example of a potential omitted variable and discussing
their effect on ß^ 1.
Expert Solution

This question has been solved!
Explore an expertly crafted, step-by-step solution for a thorough understanding of key concepts.
This is a popular solution!
Trending now
This is a popular solution!
Step by step
Solved in 1 steps

Recommended textbooks for you
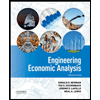
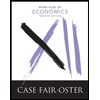
Principles of Economics (12th Edition)
Economics
ISBN:
9780134078779
Author:
Karl E. Case, Ray C. Fair, Sharon E. Oster
Publisher:
PEARSON
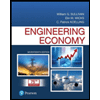
Engineering Economy (17th Edition)
Economics
ISBN:
9780134870069
Author:
William G. Sullivan, Elin M. Wicks, C. Patrick Koelling
Publisher:
PEARSON
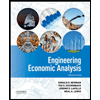
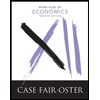
Principles of Economics (12th Edition)
Economics
ISBN:
9780134078779
Author:
Karl E. Case, Ray C. Fair, Sharon E. Oster
Publisher:
PEARSON
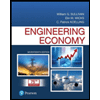
Engineering Economy (17th Edition)
Economics
ISBN:
9780134870069
Author:
William G. Sullivan, Elin M. Wicks, C. Patrick Koelling
Publisher:
PEARSON
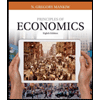
Principles of Economics (MindTap Course List)
Economics
ISBN:
9781305585126
Author:
N. Gregory Mankiw
Publisher:
Cengage Learning
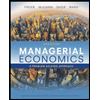
Managerial Economics: A Problem Solving Approach
Economics
ISBN:
9781337106665
Author:
Luke M. Froeb, Brian T. McCann, Michael R. Ward, Mike Shor
Publisher:
Cengage Learning
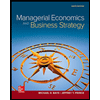
Managerial Economics & Business Strategy (Mcgraw-…
Economics
ISBN:
9781259290619
Author:
Michael Baye, Jeff Prince
Publisher:
McGraw-Hill Education