BUS 308 Week 5 Discussion Forum
docx
keyboard_arrow_up
School
University Of Arizona *
*We aren’t endorsed by this school
Course
308
Subject
Statistics
Date
Feb 20, 2024
Type
docx
Pages
4
Uploaded by MegaKuduPerson789
Suppose you wanted to predict Winnings ($) using only the number of poles won (Poles), the number of wins (Wins), the number of top five finishes (Top 5), or the number of top ten finishes (Top 10). Which of these four variables provides the best single predictor of winnings?
There are a couple ways to approach this question. First, you could run a simple Correlation analysis in Excel, which would return the following result. Based on the analysis, the independent variable with the highest correlation to winnings would be Top 10 finishes with a correlation coefficient of 0.8978 (in green). This would suggest that Top 10 finishes is the best single predictor of winnings, but Top 5 finishes are also highly correlated to winnings (naturally), so additional analysis is appropriate.
Poles
Wins
Top 5
Top 10
Winnings ($)
Poles
1
Wins
0.1331
1
Top 5
0.4373
0.7252
1
Top 10
0.4578
0.6972
0.9017
1
Winnings ($)
0.4061
0.6616
0.8612 0.8978
1
The second way to evaluate the relationship between multiple variables is to look at the multiple coefficient of determination, or the R
2
value, which is the sum of squares due to regression over total sum of squares. According to the text, the R
2 is an indicator of the goodness of fit for the estimated multiple regression equation (Anderson et al., 2021, Ch.15.8). Multiple R
0.905808159
R Square
0.820488422
Adjusted R Square
0.796553544
Standard Error
581382.1968
Observations
35
df
SS
MS
F
Significance F
Regression
4
4.63473E+13
1.15868E+13
34.28003482
8.61942E-11
Residual
30
1.01402E+13
3.38005E+11
Total
34
5.64875E+13
Coefficients
Standard Error
t Stat
P-value
Lower 95%
Upper 95%
Lower 95%
Upper 95%
Intercept
3140367.0869
184229.0243
17.0460
0.0000 2764121.2314 3516612.9424 2764121.2314 3516612.9424
Poles
-12938.9208
107205.0751
-0.1207
0.9047
-231880.8892
206003.0476
-231880.8892
206003.0476
Wins
13544.8127
111226.2163
0.1218
0.9039
-213609.4214
240699.0467
-213609.4214
240699.0467
Top 5
71629.3933
50666.8677
1.4137
0.1677
-31846.1533
175104.9399
-31846.1533
175104.9399
Top 10
117070.5768
33432.8838
3.5017
0.0015
48791.5202
185349.6334
48791.5202
185349.6334
SUMMARY OUTPUT (all)
Regression Statistics
ANOVA
The combined linear regression model in Excel returned an R
2
value of 0.8205 meaning that 82.05% of the variability in Winnings $ can be attributed to the estimated regression equation. Top 10 wins also had the only P-value that was less than α = 0.05 (0.0015 < 0.05) indicating it was the only variable that had a significant impact on Winnings variability which also seems to support the conclusion that Top 10 wins has the most impact on Winnings $.
To be determine which independent variable has the highest individual correlation to Winnings $, separate linear regressions should be modeled for each independent variable (Poles, Wins, Top 5, Top 10) and their R
2
values compared. Excel returns the following R
2
values for each of the independent variables (see attached worksheet): Poles
: 0.164907, Wins
: 0.437664,
Top 5
: 0.741610, Top 10
: 0.805966.
Comparing the R
2
values suggests that Top 10 wins with an R
2
value of .8060 are responsible for the most
variability in Winnings $. This also more clearly defines the correlation between Top 5 wins and Winnings. The initial Correlation model in Excel returned a coefficient of 0.8612 for Top 5 wins but when a linear regression was modeled to compare Top 5 wins independently, the R
2
value is only 0.7416. Based
on this analysis, Top 10 wins is the single best predictor for Winnings $. Conversely, Pole positions appear
to be the weakest predictor of winnings.
Develop an estimated regression equation that can be used to predict Winnings ($) given the number of poles won (Poles), the number of wins (Wins), the number of top five finishes (Top 5), and the number of top ten (Top 10) finishes. Test for individual significance, and then discuss your findings and conclusions.
The multiple regression equation that describes how the dependent variable ŷ (Winnings $) is related to independent variables x
1
- x
4 (Poles, Wins, Top 5, and Top 10, respectively) would be expressed as ŷ = β
0 + β
1
x
1
+
β
2
x
2
+
β
3
x
3
+
β
4
x
4
where: β
0
, β1
,
β
2
,
β
3
, and
β
4 are the parameters, or correlation coefficients of each independent variable. From the Linear Regression results in Excel, the point estimates for β
0
, β1
,
β
2
,
β
3
, and
β
4 are listed as the Coefficients in the ANOVA table from Intercept to Top 10, respectively (in green) and are expressed as b
o
,
b
1
, b
2
, b
3
, and b
4
, in the estimated multiple regression equation: ŷ = b
0 + b
1
x
1
+
b
2
x
2
+
b
3
x
3
+
b
4
x
4
Plugging in the coefficients from the Excel table as the b values gives:
ŷ = 3140367.09 -12938.92
x
1 +
13544.81
x
2 + 71629.39
x
3 + 117070.58
x
4
If the coefficients represent the estimated magnitude and direction (positive/negative) of the relationship between each independent variable and the dependent variable, this equation represent the change in winnings based on a change in the independent variable, holding all others steady. Practically speaking, this would mean that for each Win, one can expect a $13,544.81 increase in overall winnings, for each Top 5, an increase of $71,629.39, and $117,070.58 for every Top 10 finish. Comparing the coefficients confirms Top 10 wins has the most significant This regression would also suggest that for
each pole position, one could anticipate a decrease of $12,938.92 in winnings, suggesting that running the fastest single lap on an empty track is the worst indicator of future winnings.
Using the estimated regression
equation with a value of 1 for poles, wins, Top 5, and Top 10, the estimated winnings for each driver was predicted. 14 of the 35 drivers (40%) actually did better than the estimated regression equation predicted. Those who beat the estimate did so by an average of $568,819,
while those who underperformed were under prediction by $379,212 on average.
Driver
Points
Poles
Wins
Top 5
Top 10
Winnings ($)
Predicted
Result Diff
Tony Stewart
2403
1
5
9
19
6,529,870
6064157.729
Actual was higher
465,712
Carl Edwards
2403
3
1
19
26
8,485,990
7519888.607
Actual was higher
966,101
Kevin Harvick
2345
0
4
9
19
6,197,140
6063551.837
Actual was higher
133,588
Matt Kenseth
2330
3
3
12
20
6,183,580
6343149.018
Actual was lower
-159,569
Brad Keselowski
2319
1
3
10
14
5,087,740
5523344.613
Actual was lower
-435,605
Jimmie Johnson
2304
0
2
14
21
6,296,360
6628750.332
Actual was lower
-332,390
Dale Earnhardt Jr.
2290
1
0
4
12
4,163,690
4818792.661
Actual was lower
-655,103
Jeff Gordon
2287
1
3
13
18
5,912,830
6206515.1
Actual was lower
-293,685
Denny Hamlin
2284
0
1
5
14
5,401,190
5151046.942
Actual was higher
250,143
Ryan Newman
2284
3
1
9
17
5,303,020
5749959.483
Actual was lower
-446,939
Kurt Busch
2262
3
2
8
16
5,936,470
5574804.325
Actual was higher
361,666
Kyle Busch
2246
1
4
14
18
6,161,020
6291689.306
Actual was lower
-130,669
Clint Bowyer
1047
0
1
4
16
5,633,950
5313558.702
Actual was higher
320,391
What did you find in your analysis of the data? Were there any surprising results? What recommendations would you make based on your findings? Include details from your managerial report to support your recommendations.
The results showed that Top 10 and Top 5 finishes have the highest correlation to total winnings which would make sense because they offer the most prize money. The data also showed that winning Poles (having the fastest single lap time on an empty track) is the weakest indicator of winnings. This makes sense because having the fastest lap individual lap time doesn’t necessarily mean you’re even going to finish the race, let alone string together 200 fast laps in a row to get a Top 10 finish. One result I did find interesting was the coefficient for Poles was negative which would suggest for each Pole won, the overall winning total would decrease by $12,938.92. Comparing Poles to Top 5 and Top 10 wins reveals that Poles only have a ~20% correlation to a Top 5 or 10 win, which in turn have higher correlations to winnings. Also interesting is that first place finishes only have a correlation of about 44% to winnings, but not totally surprising given that winning a race is hard to do and there is only one winner per race. This leads to my final thoughts and recommendation. I think the models unfairly favor Top 10 finishes because there are more opportunities to win money in this bracket than the others, so naturally one would expect more overall winnings by volume alone. For example, in 35 races, there are only 35 winners, but there are 140 Top 5 finishers (pos. 2-5 x 35 races) and 175 Top 10 finishers (pos. 6-10 x 35). No matter how much more the winning prize is, it isn’t great enough to surpass the winnings offered through the consistency of Top 5 or 10 finishes. According to the data, a racer has better odds of winning
more money by just shooting for a Top 10 finish every week rather than running hard trying to win first and risk crashing out and winning nothing. I would recommend testing additional independent variables Top 2-5 and Top 6-10 to get a more accurate estimated regression
equation, and to determine which finishing positions are more lucrative, but at the end of the day consistency is key, and based on this data
set, I would recommend a less risky strategy of trying to win races, and just go for Top 10 finishes.
References
Anderson, D. R., Sweeney, D. J., Williams, T. A., Camm, J. D., Cochran, J. J., Fry, M. J., & Ohlmann. J. W. (2021). Essentials of modern business statistics with Microsoft® Excel®
(8th ed.). Cengage Learning.
Matt Kenseth won the 2012 Daytona 500, the most important race of the NASCAR season. His win was no surprise because for the 2011 season he finished fourth in the point standings with 2330 points, behind Tony Stewart (2403 points), Carl Edwards (2403 points), and Kevin Harvick (2345 points). In 2011
Your preview ends here
Eager to read complete document? Join bartleby learn and gain access to the full version
- Access to all documents
- Unlimited textbook solutions
- 24/7 expert homework help
he earned $6,183,580 by winning three Poles (fastest driver in qualifying), winning three races, finishing in the top five 12 times, and finishing in the top ten 20 times. NASCAR’s point system in 2011 allocated 43 points to the driver who finished first, 42 points to the driver who finished second, and so on down to
1 point for the driver who finished in the 43rd position. In addition any driver who led a lap received 1 bonus point, the driver who led the most laps received an additional bonus point, and the race winner was awarded 3 bonus points. But the maximum number of points a driver could earn in any race was 48. Table 15.8 shows data for the 2011 season for the top 35 drivers (NASCAR website).
However if H
0
cannot be rejected, we do not have sufficient evidence to conclude that a significant relationship is present.
A correlation coefficient near 0 indicates no correlation. The excel output showing the sample correlation
coefficients shows that the variable most highly correlated is with winnings dollars is the number of top 10 finishes. Looking at the p-Values corresponding to the t-values for each of the independent variables, the only significant variable based on p-value is Top 10 with a p-value of 0.0015 which is < α = 0.05. The R
Square value is .8205, while the model that included only Top 10 as an independent variable had an r square of .8060. Adding poles, wins and top of the model as independent variables added little to the model’s ability to explain variations in winnings.
T
Recommended textbooks for you
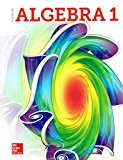
Glencoe Algebra 1, Student Edition, 9780079039897...
Algebra
ISBN:9780079039897
Author:Carter
Publisher:McGraw Hill
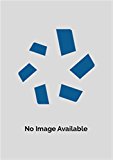
Big Ideas Math A Bridge To Success Algebra 1: Stu...
Algebra
ISBN:9781680331141
Author:HOUGHTON MIFFLIN HARCOURT
Publisher:Houghton Mifflin Harcourt
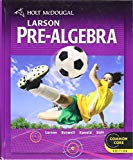
Holt Mcdougal Larson Pre-algebra: Student Edition...
Algebra
ISBN:9780547587776
Author:HOLT MCDOUGAL
Publisher:HOLT MCDOUGAL
Recommended textbooks for you
- Glencoe Algebra 1, Student Edition, 9780079039897...AlgebraISBN:9780079039897Author:CarterPublisher:McGraw HillBig Ideas Math A Bridge To Success Algebra 1: Stu...AlgebraISBN:9781680331141Author:HOUGHTON MIFFLIN HARCOURTPublisher:Houghton Mifflin HarcourtHolt Mcdougal Larson Pre-algebra: Student Edition...AlgebraISBN:9780547587776Author:HOLT MCDOUGALPublisher:HOLT MCDOUGAL
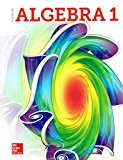
Glencoe Algebra 1, Student Edition, 9780079039897...
Algebra
ISBN:9780079039897
Author:Carter
Publisher:McGraw Hill
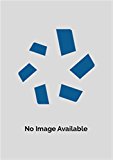
Big Ideas Math A Bridge To Success Algebra 1: Stu...
Algebra
ISBN:9781680331141
Author:HOUGHTON MIFFLIN HARCOURT
Publisher:Houghton Mifflin Harcourt
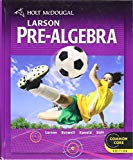
Holt Mcdougal Larson Pre-algebra: Student Edition...
Algebra
ISBN:9780547587776
Author:HOLT MCDOUGAL
Publisher:HOLT MCDOUGAL