Assignment 2 Solved(1)
docx
keyboard_arrow_up
School
University Of Connecticut *
*We aren’t endorsed by this school
Course
5604
Subject
Statistics
Date
Feb 20, 2024
Type
docx
Pages
8
Uploaded by EarlMusicCrab33
Part 1
– Refer to page 89 of your textbook and answer the following problems. Use the attached RidingMowers and LaptopSalesJanuary2008 datasets to answer the following questions. You may complete this part in either JMP or Python.
3.2 a – With most of the owners (as indicated by the blue dots) occurring in the top right area of the data space, it seems that people are people are more likely own a riding lawnmower then they have a larger lot and a higher income. This makes sense because riding lawnmowers are more expensive than push mowers so a higher income would be required to decide to make that
expensive purchase. Also, riding lawnmowers are more useful on a larger lot. If a lot is too small, it might be hard to navigate a riding lawnmower in the small space. In fact, when the lot is smaller than 16,000 sqft, nobody owns a riding lawnmower. When the lot is large, you can relax and ride your mower around instead of doing the hard work of pushing it across the yard. The data show that everyone with a lot size over 21,000 sqft owns a riding lawnmower.
3.3 a – The store in postcode N17 6OA has the highest average retail price of 495. The store in postcode W4 3PH has the lowest retail price of 481.
Part 2
– Continue working in the LaptopSalesJanuary2008 dataset to answer the following questions. You may complete this part in either JMP or Python. 1.
Assuming the dataset includes all laptop models sold by the stores, would you want to use the Screen Size column to predict Retail Price? Why or why not? Justify your choice with an appropriate visualization.
When I look at the distribution of the screen size column, I see that all values in that column are the same, exactly 15 inches. Both the max and min values are 15 inches. Because there is no variability in the data for screen size, it can’t impact the price. When a column is all one value, we don’t use it for modeling. 2.
What has a bigger impact on Retail Price – RAM or Processor Speed? Make comparative box plots to support your answer. Include screen shots of the visualizations you made. (Hint: For boxplots you should have one continuous variable and one categorical variable.)
When looking at the boxplots of the distribution of retail price subsetted by RAM, we can see that laptops with 2 GB of RAM are generally priced higher than laptops with 1 GB of RAM. The median price of laptops with 2GB of RAM is 500 compared to 470 when there’s 1 GB of RAM. The quartiles, min, and max are all higher when the RAM is 2 GB.
Your preview ends here
Eager to read complete document? Join bartleby learn and gain access to the full version
- Access to all documents
- Unlimited textbook solutions
- 24/7 expert homework help
When looking at the boxplots of the distribution of retail price subsetted by processor speed, we
can see that the medians are almost the same. They only differ by 15. The higher processor speed is higher at each key point in the box plot, but only by a little. When comparing the two variables as potential predictors, the RAM drives more differentiation in price than the processor speed. So I would expect the RAM to be a better predictor. 3.
Check the correlations among Configuration, Retail Price, and CustomerStoreDistance. Make three observations about the correlations beyond just the numerical value. What do the numbers and patterns indicate about the meaning of the data? Include a screenshot of the correlations and scatterplot matrices.
Here are some observations that occur to me from the output above:
- The configuration variable is not truly continuous. That is why you see the banding in the scatterplots that include the configuration column. There is data in the ranges from 1-80, 145-
224, and 289-368.
- As the configuration number increases, the laptop probably also has higher end features which drive up price. A little bit of extra exploration shows that the configuration ranges are based off of battery life. - At first I thought that customers seem to be a little bit more willing to travel further to purchase
the higher battery life laptop configurations. This is a very weak pattern though, as evidenced by
the 0.0021 correlation. I’m just noticing that there seems to be a bit more dots on the right side of the scatterplot for the highest group of configurations. So I binned the configurations in the ranges that indicate the different battery life and RAM groupings. I then looked at the distribution of the distance traveled for each of these. There is no noteworthy pattern here. So the configuration does not influence the distance people travel to purchase.
Your preview ends here
Eager to read complete document? Join bartleby learn and gain access to the full version
- Access to all documents
- Unlimited textbook solutions
- 24/7 expert homework help
- Price doesn’t seem to be a factor in how far people travel to purchase a laptop. They will travel
about the same distances for all different price points. To further show this relationship, I binned
the distances (assuming that within each range the person’s perception of the distance isn’t that different) and then looked at the distribution of the price for each distance. The only variability that appears is at the upper end where the data gets very thin. NOTE: In the cases where I did an extra vis to justify my answers for this question, that isn’t necessary for this assignment. Just interpreting the correlations and scatterplot matrices is
enough. I just wanted to go ahead with the next step to show you how I approach what I’m seeing.
Related Documents
Related Questions
The entirety of the data set will be in the two pictures
arrow_forward
Please use the given info to answer the subquestion Part B
arrow_forward
Please use the given info to answer the subquestion Part A
arrow_forward
The whole data set will be in the two pictures
arrow_forward
Spend at least 20 minutes looking at a few of the different unique data visualization ideas foundat this blog: http://flowingdata.com/. Discuss one of the posts in a few sentences, copying inany appropriate (and appropriately resized) graphics.
arrow_forward
The r code for side by side boxplot of vitamind v newage and vitamin d v country.
Scatterplot code for relationship between vitamin d level and age.
arrow_forward
please. help me answer this question. thank you
arrow_forward
In IBM SPSS, what does clicking on this icon do?
arrow_forward
Give a detailed outline for this:
Also, do not give plagirised answer.
Suppose that you have two sets of data. The first set is a list of all the
injuries that were seen in a clinic in a month's time. The second set
contains data on the number of minutes that each patient spent in the
waiting room of a doctor's office. You can make assumptions about
other information or variables that are included in each data set.
For each data set, propose your idea of how best to represent the key
information.
To organize your data, would you choose to use a frequency table, a
cumulative frequency table, or a relative frequency table? Why?
What type of graph would you use to display the organized data from
each frequency distribution? What would be shown on each of the
axes for each graph?
Minimum of 1 scholarly source and one appropriate resource such as
the textbook, math video, and/or math website
arrow_forward
You will use the following data set to answer all parts of the project. This data set is the number of students enrolled at CCA from 2015 to 2019 by semester
Fall 2015
6933
Summer 2015
2495
Spring 2015
7518
Fall 2016
7386
Summer 2016
2301
Spring 2016
8056
Fall 2016
8025
Summer 2016
2235
Spring 2016
8725
Fall 2018
7982
Summer 2018
2140
Spring 2018
8436
Fall 2019
5859
Summer 2019
2089
Spring 2019
9048
1) Find the mean, median, and mode for CCA Student enrollment data set. Then using the formulas for samples, find the variance and standard deviation.
2) Organize the data set on student enrollment by creating a frequency distribution and include the relative frequency. Group the data into seven logical equal intervals starting with 2,000 ≤ x < 10,000 and so on.
x
f
relative f…
arrow_forward
The basketball coach at a local college believes that his team scores more points at home games when more people show up. Below is a list of all home games last year with scores and corresponding attendance. Use Excel, SPSS, or work by hand to show your work finding r. Show your work on the attached pages.
Score
Attendance
Score
Attendance
54
380
67
410
57
350
78
215
59
320
67
113
80
478
56
250
82
451
85
450
75
250
101
489
73
489
99
472
53
451
a. What is the correlation between Score and Attendance rounded to 2 decimals?
b. In terms of strength and direction, how would you describe this correlation?
c. What is the obtained t-score for this correlation?
d. What is the critical t-score for a two-tailed test with a ?
e. Is this correlation significant based on the t-scores?
f. Based on Table 10.4, approximately how many cases would you expect to need to…
arrow_forward
The basketball coach at a local college believes that his team scores more points at home games when more people show up. Below is a list of all home games last year with scores and corresponding attendance. Use Excel, SPSS, or work by hand to show your work finding r. Show your work on the attached pages.
Score
Attendance
Score
Attendance
54
380
67
410
57
350
78
215
59
320
67
113
80
478
56
250
82
451
85
450
75
250
101
489
73
489
99
472
53
451
What is the obtained t-score for this correlation?
What is the critical t-score for a two-tailed test with a = 0.05?
Is this correlation significant based on the t-scores?
arrow_forward
The basketball coach at a local college believes that his team scores more points at home games when more people show up. Below is a list of all home games last year with scores and corresponding attendance. Use Excel, SPSS, or work by hand to show your work finding r. Show your work on the attached pages.
Score
Attendance
Score
Attendance
54
380
67
410
57
350
78
215
59
320
67
113
80
478
56
250
82
451
85
450
75
250
101
489
73
489
99
472
53
451
g. What is the coefficient of determination for this relationship?
h. Interpret for these two variables presuming a causal relationship was expected.
arrow_forward
An business reviews data on the daily amount of calls it receives. Are the data discrete or continous?
arrow_forward
Hi i’m in grade 12 Data Management and i need help with this practice question
arrow_forward
I need help with this problem please.
arrow_forward
A popular summer event is Skee-Ball. For $2, a customer purchases three balls and attempts to roll each ball into a central target. The customer wins their $2 back and wins an additional $1 if they hit the target once, an additional $3 if they hit the target twice and an additional $5 if they hit the target three times. If the customer does not hit the target at all, they lose their initial $2. Access the data set labeled ”Skee-Ball” which reports the outcomes of 2,500 games for a single day’s operation of a Skee-Ball booth.
(a) Define a random variable C (Customer Score) equal to the number of times a customer hits the target in each set of three rolls. How many possible outcomes of C are there? Report your answer as an integer.
(b) Report the net profit ($) that the Skee-Ball booth achieved for the day. Report your answer as an integer. Hint: Estimate the PDF of C based on the relative frequencies: P(C = c) = Frequency of C = c / 2,500
(c) Report P(C = 0). Round your answer to three…
arrow_forward
please do with rstudio and provide all the codes.
arrow_forward
Please help!!
From these following topics in CANADA, choose one of the 3, and formulate a research question, in which you could analyze the data.
Sawn wood: Sawn wood, production, deliveries and stocks by species
Weather: Weather data for Hamilton January 2020
Basketball - The Raptors: Statistics with the players
arrow_forward
On a cold day in Minneapolis, the afternoon temperature was 48 degrees before a cold front moved through. As
the front moved through the temperature dropped an average of 5 degrees per hour for a total of 5 hours.
14
2/1
Identify the domain of the data set.
arrow_forward
Please help me answer these and understand
arrow_forward
I need help with this problem
arrow_forward
Q3 needed to be solved correctly in 30 minutes and get the thumbs up please show neat and clean work
arrow_forward
Answer to this?
arrow_forward
Walter is a sales manager for a chain of car dealerships. He encourages the managers at each store to
spend as much time on the sales floor as they can. He is curious if this has any effect on the number of
cars sold. Each manager reports the number of hours per day he or she spends on the sales floor. From
this, Walter creates the scatterplot below showing sales and time on the floor. What information can
Walter infer from the scatterplot? Select all that apply.
10
9
8
1
1
3
4
7
Average Hours on Sales Floor
O There is a positive correlation between hours on the sales floor and sales.
O There is no correlation between hours on the sales floor and sales.
O There is a negative correlation between hours on the sales floor and sales.
O Walter should require all managers to spend more hours on the sales floor.
O Walter should make no changes to policies regarding hours on the sales floor for managers.
O Walter should help managers who spend little time on the sales floor find ways to spend…
arrow_forward
PLEASE ONLY RESPOND TO PART D, E, AND F THE REST HAS BEEN SOLVED
The average waiting time for a patient at an El Paso physician's office is just over 29 minutes, well above the national average of 21 minutes. In order to address the issue of long patient wait times, some physician's offices are using wait tracking systems to notify patients of expected wait times. Patients can adjust their arrival times based on this information and spend less time in waiting rooms. The following data show wait times (minutes) for a sample of patients at offices that do not have an office tracking system and wait times for a sample of patients at offices with an office tracking system.
Without WaitTracking System
With WaitTracking System
24
31
67
11
17
14
20
18
31
12
44
37
12
9
23
13
16
12
37
15
(a)
What are the mean and median patient wait times (in min) for offices with a wait tracking system?
mean minmedian min
What are the mean and median patient wait times (in min)…
arrow_forward
SEE MORE QUESTIONS
Recommended textbooks for you
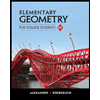
Elementary Geometry for College Students
Geometry
ISBN:9781285195698
Author:Daniel C. Alexander, Geralyn M. Koeberlein
Publisher:Cengage Learning
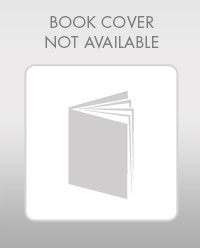
Mathematics For Machine Technology
Advanced Math
ISBN:9781337798310
Author:Peterson, John.
Publisher:Cengage Learning,
Related Questions
- The whole data set will be in the two picturesarrow_forwardSpend at least 20 minutes looking at a few of the different unique data visualization ideas foundat this blog: http://flowingdata.com/. Discuss one of the posts in a few sentences, copying inany appropriate (and appropriately resized) graphics.arrow_forwardThe r code for side by side boxplot of vitamind v newage and vitamin d v country. Scatterplot code for relationship between vitamin d level and age.arrow_forward
- please. help me answer this question. thank youarrow_forwardIn IBM SPSS, what does clicking on this icon do?arrow_forwardGive a detailed outline for this: Also, do not give plagirised answer. Suppose that you have two sets of data. The first set is a list of all the injuries that were seen in a clinic in a month's time. The second set contains data on the number of minutes that each patient spent in the waiting room of a doctor's office. You can make assumptions about other information or variables that are included in each data set. For each data set, propose your idea of how best to represent the key information. To organize your data, would you choose to use a frequency table, a cumulative frequency table, or a relative frequency table? Why? What type of graph would you use to display the organized data from each frequency distribution? What would be shown on each of the axes for each graph? Minimum of 1 scholarly source and one appropriate resource such as the textbook, math video, and/or math websitearrow_forward
- You will use the following data set to answer all parts of the project. This data set is the number of students enrolled at CCA from 2015 to 2019 by semester Fall 2015 6933 Summer 2015 2495 Spring 2015 7518 Fall 2016 7386 Summer 2016 2301 Spring 2016 8056 Fall 2016 8025 Summer 2016 2235 Spring 2016 8725 Fall 2018 7982 Summer 2018 2140 Spring 2018 8436 Fall 2019 5859 Summer 2019 2089 Spring 2019 9048 1) Find the mean, median, and mode for CCA Student enrollment data set. Then using the formulas for samples, find the variance and standard deviation. 2) Organize the data set on student enrollment by creating a frequency distribution and include the relative frequency. Group the data into seven logical equal intervals starting with 2,000 ≤ x < 10,000 and so on. x f relative f…arrow_forwardThe basketball coach at a local college believes that his team scores more points at home games when more people show up. Below is a list of all home games last year with scores and corresponding attendance. Use Excel, SPSS, or work by hand to show your work finding r. Show your work on the attached pages. Score Attendance Score Attendance 54 380 67 410 57 350 78 215 59 320 67 113 80 478 56 250 82 451 85 450 75 250 101 489 73 489 99 472 53 451 a. What is the correlation between Score and Attendance rounded to 2 decimals? b. In terms of strength and direction, how would you describe this correlation? c. What is the obtained t-score for this correlation? d. What is the critical t-score for a two-tailed test with a ? e. Is this correlation significant based on the t-scores? f. Based on Table 10.4, approximately how many cases would you expect to need to…arrow_forwardThe basketball coach at a local college believes that his team scores more points at home games when more people show up. Below is a list of all home games last year with scores and corresponding attendance. Use Excel, SPSS, or work by hand to show your work finding r. Show your work on the attached pages. Score Attendance Score Attendance 54 380 67 410 57 350 78 215 59 320 67 113 80 478 56 250 82 451 85 450 75 250 101 489 73 489 99 472 53 451 What is the obtained t-score for this correlation? What is the critical t-score for a two-tailed test with a = 0.05? Is this correlation significant based on the t-scores?arrow_forward
arrow_back_ios
SEE MORE QUESTIONS
arrow_forward_ios
Recommended textbooks for you
- Elementary Geometry for College StudentsGeometryISBN:9781285195698Author:Daniel C. Alexander, Geralyn M. KoeberleinPublisher:Cengage LearningMathematics For Machine TechnologyAdvanced MathISBN:9781337798310Author:Peterson, John.Publisher:Cengage Learning,
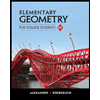
Elementary Geometry for College Students
Geometry
ISBN:9781285195698
Author:Daniel C. Alexander, Geralyn M. Koeberlein
Publisher:Cengage Learning
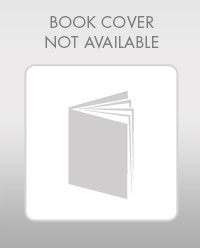
Mathematics For Machine Technology
Advanced Math
ISBN:9781337798310
Author:Peterson, John.
Publisher:Cengage Learning,