Wk 6 Discussion 1 - Confidence Intervals [due Thurs]
docx
keyboard_arrow_up
School
University of Phoenix *
*We aren’t endorsed by this school
Course
710
Subject
Statistics
Date
Feb 20, 2024
Type
docx
Pages
4
Uploaded by JudgeComputerCapybara6655
Wk 6 Discussion 1 - Confidence Intervals [due Thurs]
Due Thursday
When implementing a research study, it is important to know the relationship
between confidence intervals, sample sizes, and estimated standard errors.
Write a 250- to 300-word response to the following:
Explain the relationship between confidence intervals, sample sizes, and estimated standard errors.
How might understanding these elements be useful in understanding your mock data used throughout this course?
Confidence intervals (CI) is the best estimate for the range of a population
value that researchers come up with given the sample value. The confidence interval gets larger as the probability of being correct increases. A standard error of the mean gives a range (the confidence interval) of there the mean and the entire population likely lies. A standard error is the standard deviation of the sampling distribution. Standard errors are related to confidence intervals. A sample size ensures
the validity and reliability of a research study (Frankfort-Nachmias et al., 2019). As a sample size increases the standard error decreases because with a larger sample size, there is less variation between sample statistics. It is important for a researcher to understand these elements in order to better understand the data collected, likewise, making it important for doctoral students to understand the data collected for mock trials during this course. In looking at the data, if the sample size increases, the standard error falls and the chance of variation is reduced. By decreasing error possibilities, this increases the validity and reliability of a researcher’s study. Confidence intervals ensure the researcher how sure they can be about their data and findings and the standard error is used to estimate the efficiency and accuracy of a sample. The standard error is the most useful as a means of determining the confident level. Therefore, all three concepts are interrelated and equally important to a researcher (Altman, 2005). Altman, D. G.
(2005).
Standard deviations and standard errors.
The BMJ
, 331.
https://doi.org/10.1136/bmj.331.7521.903
Frankfort-Nachmias, C., Leon-Guerrero, A., & Davis, G. (2019). Social statistics for a diverse society
(9th ed.). SAGE Publications.
The standard error falls as the sample size increases, as the extent of chance variation is reduced—this idea underlies the sample size calculation for a controlled trial,
A confidence interval (or CI) is the best estimate of the range of a population value (or population parameter) that we can come up with given the sample value (or sample statistic).
the larger range of the confidence interval allows you to encompass a larger number of possible outcomes and you can thereby be more confident. The confidence interval gets larger as the probability of being correct increases.
.
the standard error of the mean is the standard deviation of all the possible means selected from the population. It’s the best estimate of a population mean that we can come up with, given that it is impossible to compute all the possible means. If our sample selection were perfect, and the sample fairly
represents the population, the difference between the sample and the population averages would be zero, right? Right. If the sampling from a population were not done correctly (randomly and representatively), however, then the standard deviation of all the means of all these samples could be huge, right? Right. So we try to select the perfect sample, but no matter how diligent we are in our efforts, there’s always some error. The standard error of the mean gives a range (remember that confidence interval from Chapter 9?) of where the mean for the entire population probably lies. There can be (and are) standard errors for other measures as well.
Earlier in this lesson we learned that the sampling distribution is impacted by sample size. As the sample size increases the standard error decreases. With a larger sample size there is less variation between sample statistics
, or in this case bootstrap statistics. Let's look at how this impacts a confidence interval.
The
standard deviation
is a measure of the variation or dispersion of data, how spread out the values are. The
standard error
is the standard deviation of the sampling distribution.
Standard errors are related to confidence intervals. A
confidence interval
specifies a range of plausible values for a statistic. A confidence interval has an associated
confidence level
. Ideally, we want both small ranges and higher confidence levels.
Confidence intervals for means require a critical value,
t
∗
�
∗
, which is found on the
t
tables. These critical values are dependent upon both the degree of confidence and the sample size, or more precisely, the degrees of freedom.
The confidence interval is equal to two margins of errors
and a margin of error is equal to about 2 standard errors (for 95% confidence). A standard error is the standard deviation divided by the square root of the sample size. The standard error is standard deviation of the sampling distribution of the mean, or in English, it’s basically the amount we expect the sample mean to fluctuate for a given sample size due to random sampling error.
The margin of error, and consequently the interval, is dependent upon the degree of confidence that is desired, the sample size, and the standard error of the sampling distribution
1
.
The
standard error
is a measure of the variability of the sample mean.
It is calculated as the standard deviation of the sampling distribution of the mean
2
.
The larger the sample size, the smaller the standard error will be
3
.
The
confidence interval
is a range of values that is likely to contain an unknown population parameter with a certain degree of confidence.
The degree of confidence is typically expressed as a percentage (e.g., 95%)
4
.
The width of the confidence interval depends on several factors, including the sample size, standard deviation, and level of confidence
5
.
Increasing the sample size decreases the width of confidence intervals, because it decreases the standard error.
The means and their standard errors can be treated in a similar fashion. If
a series of samples are drawn and the mean of each calculated, 95% of the means would be expected to fall within the range of two standard errors above and two below the mean of these means. This common mean would be expected to lie very close to the mean of the population. So the standard error of a mean provides a statement of probability about
the difference between the mean of the population and the mean of the sample.
This is called the 95% confidence interval ,
Confidence intervals provide the key to a useful device for arguing from a
sample back to the population from which it came. With small samples -
Your preview ends here
Eager to read complete document? Join bartleby learn and gain access to the full version
- Access to all documents
- Unlimited textbook solutions
- 24/7 expert homework help
say under 30 observations - larger multiples of the standard error are needed to set confidence limits. These come from a distribution known as the t distribution,
The standard error falls as the sample size increases, as the extent of chance variation is reduced—this idea underlies the sample size calculation for a controlled trial, for example. By contrast the standard deviation will not tend to change as we increase the size of our sample.
So, if we want to say how widely scattered some measurements are, we use the standard deviation
. If we want to indicate the uncertainty around the estimate of the mean measurement, we quote the standard error of the mean. The standard error is most useful as a means of calculating a confidence interval. For a large sample, a 95% confidence interval is obtained as the values 1.96xSE either side of the mean. We will discuss confidence intervals in more detail in a subsequent Statistics Note. The standard error is also used to calculate P values in many circumstances.
https://www.bmj.com/content/331/7521/903.full
Ideally, you want to minimize both Type I and Type II errors, but doing so is not always easy or under your control. You have complete control over the Type I error level or the amount of risk that you are willing to take (because you actually set the level itself). Type II errors, however, are not as directly controlled but instead are related to factors such as sample size. Type II errors are particularly sensitive to the number of subjects in a
sample, and as that number increases, the probability of Type II error decreases. In other words, as the sample characteristics more closely match those of the population (achieved by increasing the sample size), the likelihood that you will accept a false null hypothesis decreases.
If you reject the null you stated, you would be making an error. That type of error is also known as a Type
I error.
Type I error:The probability of rejecting a null hypothesis when it is true.
Type II error:The probability of accepting a null hypothesis when it is false
Related Documents
Recommended textbooks for you
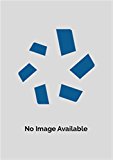
Big Ideas Math A Bridge To Success Algebra 1: Stu...
Algebra
ISBN:9781680331141
Author:HOUGHTON MIFFLIN HARCOURT
Publisher:Houghton Mifflin Harcourt
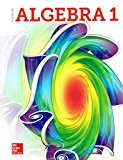
Glencoe Algebra 1, Student Edition, 9780079039897...
Algebra
ISBN:9780079039897
Author:Carter
Publisher:McGraw Hill
Recommended textbooks for you
- Big Ideas Math A Bridge To Success Algebra 1: Stu...AlgebraISBN:9781680331141Author:HOUGHTON MIFFLIN HARCOURTPublisher:Houghton Mifflin HarcourtGlencoe Algebra 1, Student Edition, 9780079039897...AlgebraISBN:9780079039897Author:CarterPublisher:McGraw Hill
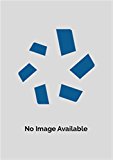
Big Ideas Math A Bridge To Success Algebra 1: Stu...
Algebra
ISBN:9781680331141
Author:HOUGHTON MIFFLIN HARCOURT
Publisher:Houghton Mifflin Harcourt
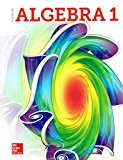
Glencoe Algebra 1, Student Edition, 9780079039897...
Algebra
ISBN:9780079039897
Author:Carter
Publisher:McGraw Hill