finalProject
pdf
keyboard_arrow_up
School
Arizona State University *
*We aren’t endorsed by this school
Course
20610
Subject
Electrical Engineering
Date
Feb 20, 2024
Type
Pages
8
Uploaded by smian
EEE 554: Random Signal Theory
Fall 2023
Final Project: Due on December 6
Lecturer: Gautam Dasarathy
Note:
The project is
due by 11:59 pm
on the date indicated. Several of these ideas were put together
by Prof. Kosut, who has graciously allowed us to use them.
This project concerns the simulation of random variables.
This is an important task in a wide variety of
applications such as verification of systems in uncertain environments, quantifying uncertainty in statistical
inference, and performing Bayesian inference. In fact, there is a strong connection between sampling and
optimization, which is crucial to several Bayesian techniques in computational science and engineering.
Modern “GenAI” applications (e.g., chatGPT and DALL-E 3) are at their core, algorithms that sample from
complex distributions.
In this project, you will implement and analyze an algorithm for simulating a given distribution.
That
is, given a PDF, your goal would be to effciently generate samples from this distribution.
First, read
and understand this document explaining the simulation algorithm in its entirety
. Then, you
may write code to implement and analyze.
(Python or Matlab is recommended, but not required.)
You
should turn in a report which includes your well-documented code, as well as a write-up describing your
implementation details, and the answers to specific questions asked below.
Specific tasks that
must
be
covered in your project report will be labeled by the bold word
Task
.
You should make sure that your
response to these tasks are
clearly presented
in your final report.
Your grade for the project will be 50% based on the correctness of your algorithm implementation, and 50%
based on the clarity of your project report. You may discuss the project in broad terms with other students,
but you must work independently.
Any incident of academic dishonesty will result in a zero in the project.
Note:
The instructor reserves the right to interview the student to determine incidence of
academic dishonest
1.1
Rejection Sampling and The Ziggurat Algorithm
The idea of rejection sampling is to define a two-dimensional region
R
that contains the target PDF
f
S
. This
idea can be generalized slightly by using a function
g
(
x
)
which is proportional to the target PDF
f
S
(
x
)
; that
is,
f
S
(
x
) =
cg
(
x
)
for some constant
c
. Then the region
R
should be such that it includes the function
g
(
x
)
.
(See Figure
1.1
.) A random pair
X, Y
is generated uniformly in the region
R
. If
Y < g
(
X
)
, then the sample
X
is accepted. Otherwise the sample is rejected and another random pair is generated, repeating until a
sample is accepted. This accepted sample will be returned as a random sample from the given distribution.
1-1
1-2
Final Project: Due December 6
Figure 1.1: The basic rejection sampling concept.
Task 1: Theory
You will first establish why such a procedure could work. You may safely postpone this problem to
until after you tackle the rest of the project.
Let us suppose that we want a sample from the distribution
f
S
(
x
)
, and let us suppose that it much
easier to sample from another distribution
h
(
x
)
that is such that
Mh
(
x
)
> f
S
(
x
)
for all
x
, where
M
≥
1
is some constant.
Now, let us suppose you do the following procedure: (a) sample
(
X, Y
)
uniformly from the region
R
that lies under
Mh
(
x
)
(notice that this is equivalent to sampling
X
from
h
(
x
)
and then sampling
Y
uniformly from
(0
, Mh
(
X
))
), and (b) return
X
if the sample drawn
lies under the envelope of
f
S
. If (b) fails, continue until you get one sample.
Show that the sample
X
returned by the above procedure has the distribution
f
S
(
x
)
as desired.
Hint:
Let
A
be the event that the step (b) succeeds. Now, notice that what you’re required to show
is that
f
(
X
|
A
)
, the PDF of
X
conditioned on the event
A
happening, is equal to
f
S
(
x
)
. For this,
you can use the Bayes’ rule as follows:
f
(
X
|
A
) =
P
(
A
|
X
)
f
(
X
)
P
(
A
)
,
where
f
(
X
)
is the unconditional distribution of
X
. What is this equal to? Once this is clear, note
that all that you need to do is to understand what
P
(
A
)
and
P
(
A
|
X
)
look like.
To make rejection sampling effcient, two objectives must be satisfied:
1. The region
R
should be designed so that it is easy to generate random pairs
X, Y
uniformly from the
region.
2. The probability of accepting a given pair should be as large as possible. This requires that the region
R
does not include much area above
g
(
x
)
.
A particularly effcient way to do this is via the Ziggurat algorithm.
This algorithm assumes that the
function
g
(
x
)
is monotonically decreasing; the cases where it is not may be handled quite easily. The region
R
consists of
n
sub-regions stacked on top of each other:
n
−
1
of these sub-regions are rectangles, and the
n
th is rectangle adjoined to the tail of the distribution. This idea is illustrated in Figure
1.2
, where each of
the
n
regions (in the figure
n
= 4
) is shaded in a different color.
The rectangles are set up so that each of the
n
sub-regions (including the
n
th region, which is not a rectangle)
have exactly the same area.
Thus, a point
X, Y
can be uniformly chosen from the full region as follows:
Homework 1: December 6
1-3
Region 1
Region 2
Region 3
Region 4
Figure 1.2: Diagram of the Ziggurat algorithm with
n
= 4
. The name “Ziggurat algorithm” comes from the
fact that this picture looks a little bit like a Ziggurat.
Figure 1.3: Diagram of the
k
th region of the Ziggurat algorithm.
First, choose a sub-region from a discrete uniform distribution—i.e., each with probability
1
/n
—in Matlab
this can be done with the function
randi
.
Then, for the rectangular regions,
X
can be chosen from a
uniform distribution along the length of the rectangle, and
Y
can be chosen from a uniform distribution
along the height of the rectangle.
The
n
th region requires a more complicated algorithm, but since this
is only needed with probability
1
/n
, this more complicated algorithm only needs to be run rarely.
Thus,
choosing a candidate
X, Y
is easy, which satisfies objective 1. If the number of regions
n
is large enough,
then the stack of regions closely matches the function
g
(
x
)
, which satisfies objective 2.
If we focus on a single one of the rectangular regions, we can see another feature that makes the Ziggurat
algorithm so fast. Figure
1.3
shows the
k
th region, for some
k < n
. The
k
th region consists of a rectangle
extending horizontally from
x
1
to
x
k
+1
, and vertically from
y
k
+1
to
y
k
. As shown in Figure
1.2
,
y
k
=
g
(
x
k
)
for each
k
. Since
g
(
x
)
is decreasing,
y
k
+1
< y
k
. We can easily choose a point uniformly from this rectangle
by generating
X
∼ U
(
x
1
, x
k
+1
)
and
Y
∼ U
(
y
k
+1
, y
k
)
. After generating this point, we need to check whether
Y < g
(
X
)
. However, if
X < x
k
, then
Y < g
(
X
)
no matter what
Y
is! This means that we do not even
need to generate
Y
, nor evaluate the function
g
(
X
)
, unless
X > x
k
. If
n
is chosen to be large enough, each
rectangle will be much wider than it is tall, so the case where
X > x
k
occurs only rarely.
To implement the Ziggurat algorithm, first the values
x
1
, x
2
, . . . , x
n
and
y
1
, y
2
, . . . , y
n
must be pre-computed.
This pre-computation requires some effort, but it only needs to be done once. After these values are com-
puted, the algorithm to generate one sample from the target distribution is summarized by the following
pseudo-code.
Your preview ends here
Eager to read complete document? Join bartleby learn and gain access to the full version
- Access to all documents
- Unlimited textbook solutions
- 24/7 expert homework help
1-4
Final Project: Due December 6
Result:
Returns one sample from distribution
f
S
while
true
do
Generate
K
uniformly from
{
1
,
2
, . . . , n
}
if
K < n
then
Generate
X
∼ U
(
x
1
, x
K
+1
)
if
X < x
K
then
return
X
else
Generate
Y
∼ U
(
y
K
+1
, y
K
)
if
Y < g
(
X
)
then
return
X
end
end
else
Generate a point from the
n
th region
end
end
The above pseudo-code does not give details about how do deal with the
n
th region. We’ll get back to that!
Note that, most of the time, to generate a sample of the target distribution, all we need to do is generate one
uniform discrete variable
K
, generate one continuous uniform random variable
X
, and do one comparison
X < x
k
. Even though the alternative paths—when
k
=
n
, or
X > x
k
, or
Y > g
(
X
)
—are more complicated,
these alternative paths are rare, so most of the time we do not need to execute them.
1.2
Implement a baseline sampling algorithm
The baseline sampling algorithm uses a well known trick to generate samples from a given distribution. One
can simply draw a sample
U
from the uniform random variable on
[0
,
1]
(i.e.,
U
∼
Unf(0
,
1)
), and return
F
−
1
S
(
U
)
, where
F
S
is the CDF of the desired distribution.
Task 2: Theory
Suppose that
U
∼
Unf(0
,
1)
. Show that the random variable
Z
=
F
−
1
S
(
U
)
has the desired distribution.
Hint:
Consider the CDF of
Z
.
Each of you is assigned a continuous distribution with a PDF of the form
f
S
(
x
) =
{
cg
(
x
)
,
x > x
1
0
,
x < x
1
where
g
(
x
)
is a monotonically decreasing function in the range
x > x
1
.
This assignment is randomized
and will appear on your Canvas page.
Task 3
Find the constant
c
so that your PDF is normalized correctly.
Calculating
c
requires evaluating the integral
∫
∞
x
1
g
(
x
)
dx.
Homework 1: December 6
1-5
For some distributions, this integral is solvable in closed form. If it is not, you may use numerical integration
software to calculate
c
numerically. In Matlab, you could use the
integral
function. In Python, you could
use SciPy’s numerical integration function
scipy.integrate
. You are also welcome to use Wolfram Alpha to
do this, if possible. If you take the last route, please include a screenshot of the computation you performed
on Wolfram Alpha in the report.
Task 4
Write a function that computes the CDF of your distribution.
As above, for some distributions the CDF will have a closed form expression, but for others it will require
numerical integration.
Task 5
Implement a baseline sampling algorithm via the transformation
S
=
F
−
1
S
(
U
)
where
U
∼ U
(0
,
1)
.
This baseline algorithm will be used to compare against the more effcient Ziggurat algorithm. To implement
this baseline algorithm requires inverting the CDF. If you cannot find a closed-form expression for this inverse
function, you may need to implement the bisection algorithm, which is summarized below. This algorithm
can be used for any monotonic function
h
(
x
)
. The idea is, in order to find a value
x
where
h
(
x
) =
u
, maintain
values
x
min
and
x
max
where
h
(
x
min
)
< u
and
h
(
x
max
)
> u
. A point
x
is chosen at the midpoint between
x
min
and
x
max
. Based on the value of
h
(
x
)
, either
x
min
or
x
max
is updated to halve the difference between
them. (This pseudo-code assumes that
h
is increasing; the same algorithm can be used for monotonically
decreasing functions with a slight variation.)
‘
Input:
u
,
x
min
,
x
max
where
h
(
x
min
)
< u
and
h
(
x
max
)
> u
Output:
x
where
h
(
x
) =
u
while
x
max
−
x
min
>
tol
do
x
←
x
min
+
x
max
2
if
h
(
x
)
> u
then
x
max
←
x
;
else
x
min
←
x
;
end
end
The tolerance parameter tol determines exactly how precise the result is. Setting tol
= 10
−
12
or smaller is
often a good choice.
1-6
Final Project: Due December 6
Task 6
Generate at least 1000 samples from your baseline sampling algorithm.
Keep track of the time it
takes to run on your computer. In Python, you can use the package
time
to do this as follows:
import
time
t0 = time.time()
code_block
t1 = time.time()
time_elapsed = t1 − t0
In Matlab, this can be done with the commands
tic
and
toc
. Plot an estimated PDF from these
samples, and make sure it matches the true PDF.
1.3
Set up the Ziggurat
Before generating samples with the Ziggurat algorithm, the values
x
1
, x
2
, . . . , x
n
must be pre-computed,
as well as the corresponding values
y
1
, y
2
, . . . , y
n
, where
y
k
=
g
(
x
k
)
for each
k
. These numbers should be
selected so that each of the
n
regions have exactly the same area. For
k < n
, the
k
th region is a rectangle,
so its area is
(
x
k
+1
−
x
1
)(
y
k
−
y
k
+1
)
.
For the
n
th region, the area is
(
x
n
−
x
1
)
y
n
+
∫
∞
x
n
g
(
x
)
dx.
To set up the Ziggurat, first choose a guess
A
for the area of each region. Based on this
A
, find
x
2
so that the
1st region has area
A
. This can be done using the bisection algorithm. Then find
x
3
so that the 2nd region
has area
A
.
Continue computing
x
4
, . . . , x
n
.
Given the value that you get for
x
n
, compute the resulting
area of the
n
th region. If this area is less than
A
, then the original guess for
A
must have been too large; if
greater than
A
, then the original choice of
A
must have been too small. Again, the bisection algorithm can
be used to find the exact value of
A
.
Task 7
Calculate
x
1
, x
2
, . . . , x
n
and
y
1
, y
2
, . . . , y
n
for three values of
n
: 4, 32, and 256. Check to make sure
x
1
< x
2
<
· · ·
< x
n
. Be sure to include the code that you used. Also list the
x
k
and
y
k
numbers for
the
n
= 4
case. (Your report does not need to list the numbers for
n
= 32
,
256
.)
1.4
Sampling from the
n
th region
Since the
n
th region of the Ziggurat is not a rectangle, it requires a different algorithm to sample from.
However, since this region only comes up with probability
1
/n
, the algorithm does not need to be especially
effcient.
Figure
1.4
shows the
n
th region.
It can be separated into two parts: (i) a rectangle extending
horizontally from
x
1
to
x
n
and vertically from
0
to
y
n
, and (ii) an infinitely long tail extending from
x
n
to
∞
, and upper bounded by
g
(
x
)
.
Your preview ends here
Eager to read complete document? Join bartleby learn and gain access to the full version
- Access to all documents
- Unlimited textbook solutions
- 24/7 expert homework help
Homework 1: December 6
1-7
Figure 1.4: Diagram of the
n
th region of the Ziggurat algorithm.
Task 8
If the point
X, Y
is chosen uniformly from this region, compute the probability
p
that it falls into the
rectangular part of the
n
th region.
Remember that we do not really need
Y
, we only need
X
. When
X, Y
is in the rectangular part, then
X
is
simply uniform from
x
1
to
x
n
. Thus, we can do the following: with probability
p
, generate
X
∼ U
(
x
1
, x
n
)
.
Otherwise, generate
X
from the tail distribution.
Generating
X
from the tail distribution is the last piece of the Ziggurat algorithm. This requires generating
a random variable from the PDF
f
T
(
x
) =
{
c
′
g
(
x
)
,
x > x
n
0
,
x < x
n
where the constant
c
′
is chosen appropriately.
Task 9
Find and implement a method to sample from the tail distribution
f
T
. One of two methods can be
used, depending on your distribution:
1. If
g
(
x
)
has a closed-form integral, then a version of the inverse-CDF transformation can be
used, as in your baseline sampling algorithm.
2. If
g
(
x
)
does not have a closed-form integral, you can use another rejection sampling algorithm.
Here you can use the normal rejection sampling algorithm: choose a distribution
f
R
defined on
x > x
n
that is easy to sample from, and a constant
M
such that
Mf
R
(
x
)
> g
(
x
)
for all
x > x
n
.
For the distribution
f
R
, you may wish to use the exponential distribution restricted to
x > x
n
;
another option is the Pareto distribution, given by PDF
f
R
(
x
) =
{
αx
α
n
x
α
+1
,
x > x
n
0
,
x <
0
where
α >
0
is a parameter. The exact choice of distribution
f
R
is up to you, but be sure to
explain your choice. To implement this rejection sampling algorithm, generate
X
∼
f
R
, and
Y
∼ U
(0
, Mf
R
(
X
))
. Accept if
Y < g
(
X
)
, and repeat if not. Note that this rejection sampling
loop should be done inside a single iteration of the outer rejection sampling loop.
1-8
Final Project: Due December 6
1.5
Implement and analyze the Ziggurat algorithm
The final step is to complete the implementation of the Ziggurat for various values of
n
.
Task 10
Complete the implementation of the Ziggurat algorithm for
n
= 4
,
n
= 32
, and
n
= 256
.
Congratulations! You now have your own shiny stochastic simulator! Let us analyze it to understand how
well it does.
Task 11
For each of the three
n
values, run your Ziggurat algorithm to generate at least 1,000,000 samples.
Make sure you compute the
x
1
, . . . , x
n
and
y
1
, . . . , y
n
constants only once. For each of the
n
values,
use these samples to plot an estimated PDF, and compare against the true PDF. Carefully check that
these match for your
n
= 4
algorithm, to make sure that your tail algorithm is working correctly.
The next is something you should be doing throughout your implementation. However, have an explicitly
labeled part of your report that reports on the following statistics.
Task 12
As you generate samples from the three variants of your algorithm, keep track of the following data:
1. How long it takes to run. Compare the time per sample with your baseline algorithm.
a
Make
sure you run your code on the same computer, so that the comparison in meaningful.
2. How often each of the following possible outcomes occurs in the rejection loop:
(a)
X
is accepted because
X < x
k
,
(b)
X > x
k
but
X
is accepted because
Y < g
(
X
)
,
(c)
Y > g
(
X
)
so
X
is rejected,
(d)
k
=
n
and a sample is drawn from the rectangular part of the
n
th region,
(e)
k
=
n
and the tail algorithm is run.
a
Some of you may find that your baseline algorithm actually runs faster than the Ziggurat algorithm.
If this is
happens even for
n
= 256
, be sure to check your Ziggurat implementation to make sure it is working correctly. However,
even if everything is working right, the baseline algorithm may still run faster.
This could happen for two reasons.
First, for some distributions with closed-form CDFs, computing the inverse CDF
F
−
1
S
can be quite fast. Second, since
Matlab is an interpreted language, it sometimes runs quite slowly compared to the same algorithm implemented in a
language like C. For example, Matlab is notoriously slow when it comes to loops. Since the goal of this project is for
you to understand the algorithm rather than to build a commercial product, this is nothing to worry about.
Task 13
Can you think of any ways to further improve the performance of your algorithm?
Related Documents
Related Questions
In signal processing
1.what are the singularity problem of CMA algorithm
2. Why such singularity problem induced by using CMA
3.how to prevent the problem
It would be appreciated if you can answer in handwritten notes.
Please don't direct copied from the internet, I need explanation, thank you
arrow_forward
If you were to program a device that had a 4-bit ADC converter to read an input signal, and then command an output PWM signal, what are the duty cycle percentages of the output signals using the assumption that they are evenly separated to give you the full range of potential signals. (i.e. 0% --> 100%). Show your work or explain how you found your answer.
arrow_forward
Explain the concept of discrete-time system modeling and its application in digital control systems and signal processing.
arrow_forward
This question would help revise concepts related to MOS device and basic circuit design. Please mark all
correct answers to the following multiple choice questions.
1.1. Which of the following are true about MOSFET devices?
(a) MOS stands for "Metallurgy Of Semiconductors"
(b) MOSFET current conduction is due to drift phenomenon
(c) An externally induced voltage at the gate is fundamental to inversion layer
(d) Naming of "Source" and "Drain" regions are only dependent on aspect ratio
1.2. Which of the following are true about NMOS transistors
(a) The threshold voltage can be positive or negative depending on geometry
(b) Channel length modulation parameter (A) is always positive
(c) Current flows from drain to source
(d) Threshold voltage is a fixed value based on process, it cannot be changed by external voltages
1.3. In a MOS device
(a) Cas and CGp both have both geometry and voltage dependencies
(b) Cas is takes minimum value at saturation while CGD goes to maximum value
(c) CoB and…
arrow_forward
Explain State Space Representation of Nth order Linear Differential Equation.
arrow_forward
Don't give handwritten.
arrow_forward
Uzman Soru-Cevap
Bitti
The terms RMS, MSE, and SNR were used in Section 3.6.2 in connection with
tization. These tems are important in many aspects of signal processing besides
quantization.
(a) Explain in words the meaning of RMS, MSE, and SNR. What physical charac-
teristic do they represent?
quan-
(b) MSE is defined as
MSE = Ew(x) – x(1)°.
%3D
Explain each term of the equation.
(c) RMS is defined as
RMS =
x(n)
Explain each term of the equation.
(d) SNR in decibels is defined as
x*(n)
SNR = 10 logio
arrow_forward
SEE MORE QUESTIONS
Recommended textbooks for you
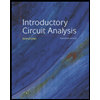
Introductory Circuit Analysis (13th Edition)
Electrical Engineering
ISBN:9780133923605
Author:Robert L. Boylestad
Publisher:PEARSON
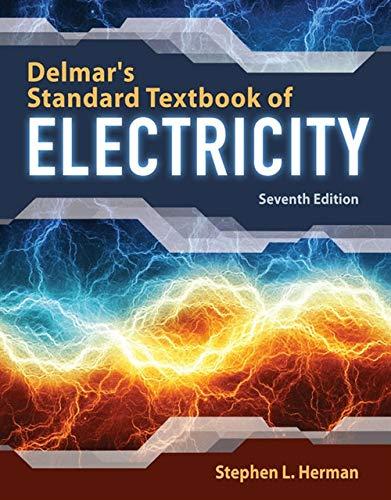
Delmar's Standard Textbook Of Electricity
Electrical Engineering
ISBN:9781337900348
Author:Stephen L. Herman
Publisher:Cengage Learning
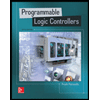
Programmable Logic Controllers
Electrical Engineering
ISBN:9780073373843
Author:Frank D. Petruzella
Publisher:McGraw-Hill Education
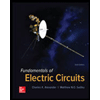
Fundamentals of Electric Circuits
Electrical Engineering
ISBN:9780078028229
Author:Charles K Alexander, Matthew Sadiku
Publisher:McGraw-Hill Education
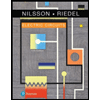
Electric Circuits. (11th Edition)
Electrical Engineering
ISBN:9780134746968
Author:James W. Nilsson, Susan Riedel
Publisher:PEARSON
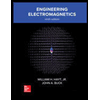
Engineering Electromagnetics
Electrical Engineering
ISBN:9780078028151
Author:Hayt, William H. (william Hart), Jr, BUCK, John A.
Publisher:Mcgraw-hill Education,
Related Questions
- In signal processing 1.what are the singularity problem of CMA algorithm 2. Why such singularity problem induced by using CMA 3.how to prevent the problem It would be appreciated if you can answer in handwritten notes. Please don't direct copied from the internet, I need explanation, thank youarrow_forwardIf you were to program a device that had a 4-bit ADC converter to read an input signal, and then command an output PWM signal, what are the duty cycle percentages of the output signals using the assumption that they are evenly separated to give you the full range of potential signals. (i.e. 0% --> 100%). Show your work or explain how you found your answer.arrow_forwardExplain the concept of discrete-time system modeling and its application in digital control systems and signal processing.arrow_forward
- This question would help revise concepts related to MOS device and basic circuit design. Please mark all correct answers to the following multiple choice questions. 1.1. Which of the following are true about MOSFET devices? (a) MOS stands for "Metallurgy Of Semiconductors" (b) MOSFET current conduction is due to drift phenomenon (c) An externally induced voltage at the gate is fundamental to inversion layer (d) Naming of "Source" and "Drain" regions are only dependent on aspect ratio 1.2. Which of the following are true about NMOS transistors (a) The threshold voltage can be positive or negative depending on geometry (b) Channel length modulation parameter (A) is always positive (c) Current flows from drain to source (d) Threshold voltage is a fixed value based on process, it cannot be changed by external voltages 1.3. In a MOS device (a) Cas and CGp both have both geometry and voltage dependencies (b) Cas is takes minimum value at saturation while CGD goes to maximum value (c) CoB and…arrow_forwardExplain State Space Representation of Nth order Linear Differential Equation.arrow_forwardDon't give handwritten.arrow_forward
arrow_back_ios
arrow_forward_ios
Recommended textbooks for you
- Introductory Circuit Analysis (13th Edition)Electrical EngineeringISBN:9780133923605Author:Robert L. BoylestadPublisher:PEARSONDelmar's Standard Textbook Of ElectricityElectrical EngineeringISBN:9781337900348Author:Stephen L. HermanPublisher:Cengage LearningProgrammable Logic ControllersElectrical EngineeringISBN:9780073373843Author:Frank D. PetruzellaPublisher:McGraw-Hill Education
- Fundamentals of Electric CircuitsElectrical EngineeringISBN:9780078028229Author:Charles K Alexander, Matthew SadikuPublisher:McGraw-Hill EducationElectric Circuits. (11th Edition)Electrical EngineeringISBN:9780134746968Author:James W. Nilsson, Susan RiedelPublisher:PEARSONEngineering ElectromagneticsElectrical EngineeringISBN:9780078028151Author:Hayt, William H. (william Hart), Jr, BUCK, John A.Publisher:Mcgraw-hill Education,
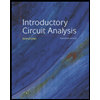
Introductory Circuit Analysis (13th Edition)
Electrical Engineering
ISBN:9780133923605
Author:Robert L. Boylestad
Publisher:PEARSON
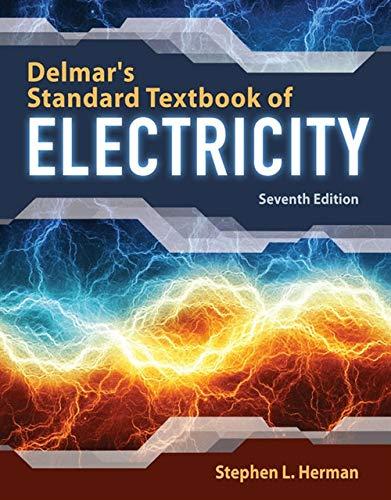
Delmar's Standard Textbook Of Electricity
Electrical Engineering
ISBN:9781337900348
Author:Stephen L. Herman
Publisher:Cengage Learning
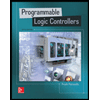
Programmable Logic Controllers
Electrical Engineering
ISBN:9780073373843
Author:Frank D. Petruzella
Publisher:McGraw-Hill Education
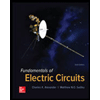
Fundamentals of Electric Circuits
Electrical Engineering
ISBN:9780078028229
Author:Charles K Alexander, Matthew Sadiku
Publisher:McGraw-Hill Education
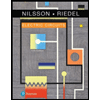
Electric Circuits. (11th Edition)
Electrical Engineering
ISBN:9780134746968
Author:James W. Nilsson, Susan Riedel
Publisher:PEARSON
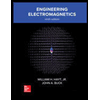
Engineering Electromagnetics
Electrical Engineering
ISBN:9780078028151
Author:Hayt, William H. (william Hart), Jr, BUCK, John A.
Publisher:Mcgraw-hill Education,