Research PPT_Unchanged
pptx
keyboard_arrow_up
School
GGDC Barikot *
*We aren’t endorsed by this school
Course
Y6555
Subject
Electrical Engineering
Date
Nov 24, 2024
Type
pptx
Pages
14
Uploaded by sowmyareddy98
AI For Predictive Maintenance in IoT
•
Predictive maintenance is an important part of the IoT ecosystem because it enables problems to be found and
fixed before they cause equipment failure or expensive downtime. Predictive maintenance in IoT systems
relies heavily on artificial intelligence (AI) since it can process massive volumes of data from interconnected
devices and provide useful insights. This abstract delves at the potential of AI for predictive maintenance in
the Internet of Things. Predictive maintenance is introduced as a solution to the problems that have plagued
more conventional methods of maintenance (Adhikari et al., 2023). The paper then explores the use of AI in
predictive maintenance, focusing on the advantages of AI in terms of data processing, analysis, and learning.
The article delves at the use of several AI methods, including machine learning and deep learning, in
predictive maintenance. The advantages of using AI for predictive maintenance in the Internet of Things are
discussed in the abstract as well. These advantages include better equipment dependability, more operational
efficiency, and lower maintenance costs. Data quality, privacy, and the requirement for domain knowledge in
constructing AI models for predictive maintenance are all discussed, as are other possible obstacles and
concerns (Adhikari et al., 2023).
Introduction
•
When it comes to maintaining the best possible performance and dependability of devices connected to the
Internet of Things (IoT), predictive maintenance is an essential component. It entails using methods from
artificial intelligence (AI) to evaluate data received from sensors and other sources in order to forecast
probable faults or the need for maintenance before they take place. Organizations are able to enhance their
overall operational efficiency, decrease the amount of time their systems are out, and save money by using
predictive maintenance in IoT systems (Adhikari et al., 2023).
Introduction
•
The paper attempts to solve an issue that has been identified in the field of Internet of Things (IoT) research,
which is the dearth of solutions for predictive maintenance that are both effective and efficient. Traditional
methods of maintenance often depend on either proactive or reactive maintenance, both of which may
consume a lot of time and money and are prone to mistakes (Byun et al., 2016). This results in an increase in
total downtime as well as a drop in overall productivity. As a result, there is a need for innovative AI-based
methodologies that are able to provide accurate predictions on the need for maintenance in IoT systems.
Your preview ends here
Eager to read complete document? Join bartleby learn and gain access to the full version
- Access to all documents
- Unlimited textbook solutions
- 24/7 expert homework help
Introduction
•
This paper is relevant since there is an increasing use of Internet of Things devices across a variety of
different businesses. The growing number of connected devices makes it more important than ever to develop
and implement effective maintenance plans. Organizations are able to proactively anticipate probable
problems, plan maintenance tasks, and optimize resource allocation when they deploy AI for predictive
maintenance in IoT. This has the potential to result in considerable cost savings, enhanced satisfaction for
customers, and increased operational efficiency (Byun et al., 2016).
•
When applied to Internet of Things (IoT) systems, AI's predictive maintenance capabilities have great
relevance and value in terms of increasing operating efficiency and decreasing downtime (Gupta & Quamara,
2020). However, there are obstacles and problems that need to be solved, such as difficulties in data
collection and quality, the complexity of algorithms, integration concerns, and reluctance to change. By
addressing these hurdles, businesses will be able to leverage the potential of artificial intelligence (AI) to
properly forecast the need for maintenance, enhance decision-making, and realize cost savings in IoT
systems.
AI be effectively applied for predictive maintenance in IoT systems
•
AI may be efficiently deployed for predictive maintenance within IoT systems by applying machine learning
algorithms to evaluate massive volumes of data received in real-time from sensors and devices. This opens
the way for AI to play a significant role in this area. These data may include information on the functionality,
health, and patterns of use of the Internet of Things (IoT) devices (Kumar, Tiwari & Zymbler, 2019). Through
the analysis of this data, AI is able to recognize trends, abnormalities, and possible failures, which enables
preventative maintenance steps to be conducted prior to the occurrence of a breakdown. Additionally, AI can
assist in optimizing maintenance schedules and predicting the remaining usable life of the equipment, both of
which enable cost-effective maintenance planning.
Edge Computing and AI Improve Iot Real-Time Predictive Maintenance
•
By moving AI algorithms to locations closer to the devices themselves, the combination of edge computing and artificial intelligence may
improve the actual time predictive maintenance capabilities of internet of things devices. Instead of transmitting all of the data to the cloud in order
to be analyzed there, edge computing processes and analyzes the data at the very edge of the network, which is much closer to the Internet of Things
devices. This results in a reduction in latency and allows decisions to be made more quickly and efficiently.
•
IoT devices are able to evaluate the data they create locally, in real-time, thanks to the combination of edge computing and AI. This allows the
devices to make rapid maintenance forecasts or trigger maintenance actions without depending on cloud access. The responsiveness and
dependability of the predictive maintenance system are both improved as a result of this. In addition, edge computing lessens the quantity of data
that must be sent to the cloud, which in turn decreases the amount of bandwidth that is necessary while simultaneously enhancing users' overall
safety and confidentiality. There is a lower chance of data breaches or privacy issues connected with transferring data to the cloud since sensitive
data may be handled and analyzed locally on the edge devices (Sadeeq &Zeebaree, 2021).
Your preview ends here
Eager to read complete document? Join bartleby learn and gain access to the full version
- Access to all documents
- Unlimited textbook solutions
- 24/7 expert homework help
Data Collection
•
In the Internet of Things, devices like as sensors and actuators are used to gather data in real time about the
circumstances in which machinery is operating. These instruments have the capability to monitor a wide
variety of characteristics, including temperature, pressure, vibration, and many more. The data that was
gathered is then sent to a central repository so that it may be analyzed further (Zanella et al., 2014).
•
The data that has been acquired is being monitored in real time by using AI algorithms. These algorithms are
able to do an analysis on the data streams and locate any irregularities or departures from the typical
operating circumstances. Identifying anomalous behavior may be accomplished via the use of machine
learning strategies such as pattern recognition or anomaly detection.
Continuous Learning and Improvement
•
The precision and utility of AI algorithms may be continually improved by learning from fresh data and
receiving feedback on their performance. The algorithms will be able to improve their models as more data is
gathered and processed, which will ultimately result in improved defect identification and diagnosis over
time. The iterative learning process contributes to the improvement of the predictive maintenance system as a
whole. In general, the use of AI for predictive maintenance in IoT makes it possible for businesses to
transition away from reactive and time-based maintenance methods and toward proactive and condition-based
maintenance (Sadeeq &Zeebaree, 2021). AI algorithms, when given access to real-time data, have the
potential to assist in spotting probable problems and failures before they occur. This may result in better
equipment dependability, decreased costs associated with maintenance, and improved operating efficiency.
AI is used in a number of different facets of predictive maintenance within the IoT
•
Predictive Analytics and Maintenance Planning
•
The previous data may be analyzed by AI systems, which can then detect trends that suggest when it is probable that
equipment will malfunction. Because these failures may be predicted in advance, maintenance personnel have the ability to plan
and schedule repairs or replacements, which in turn reduces downtime and costs.
•
Proactive Maintenance and Condition Monitoring
•
Artificial intelligence algorithms are able to continually monitor the real-time data from IoT devices and discover
deviations from typical operating circumstances. Because of this, maintenance personnel are able to take proactive actions
before any breakdown occurs, such as modifying operational settings or doing preventative maintenance.
Your preview ends here
Eager to read complete document? Join bartleby learn and gain access to the full version
- Access to all documents
- Unlimited textbook solutions
- 24/7 expert homework help
Conclusion
•
Artificial intelligence has shown that it is a useful tool for doing predictive maintenance on IoT equipment. AI is able to
effectively forecast the breakdown of equipment and the need for maintenance before it occurs by using algorithms for machine
learning and doing data analysis in real time. This proactive strategy helps firms limit the amount of downtime they experience,
which in turn helps them lower the expenses associated with maintenance and enhance their overall operating efficiency (Javaid et
al., 2021).
•
AI algorithms are able to evaluate massive amounts of sensor data collected by Internet of Things (IoT) devices, recognize
patterns in the data, and spot abnormalities that may point to impending problems with equipment. This allows firms to schedule
maintenance work in a more strategic and effective way, which in turn helps them to prevent unexpected downtime and maximize
asset usage.
Conclusion
•
In addition, AI-based predictive maintenance may assist in the optimization of maintenance schedules by taking
into consideration a variety of parameters like the utilization of equipment, the conditions of the environment, and
previous data. Because of this, businesses are able to prioritize maintenance chores according to the importance of the
equipment and then distribute resources in accordance with those priorities.
AI can also increase the accuracy of fault
detection by comparing real-time data with previous data and known failure patterns. This may be done by analyzing the
data in many time periods.
•
In addition to this, it may give insights into the underlying reasons of failures, which enables companies to take
preventative actions against problems in the future. Implementing AI for predictive maintenance in IoT systems, on the
other hand, calls for careful planning and attention (Hwang & Choi, 2018). The massive amounts of data produced by
Internet of Things devices need to be collected, stored, and analyzed, and businesses need to make sure they have the
appropriate infrastructure in place to do so. In order to evaluate the efficacy of a predictive maintenance program, they
need to first set crystal-clear goals and objectives for the program, and then identify its key performance indicators.
References
•
Adhikari, D., Wei Jiang, Jinyu Zhan, Zhiyuan He, Rawat, D. B., Aickelin, U., & Khorshidi, H. A. (2023). A
Comprehensive Survey on Imputation of Missing Data in Internet of Things.
ACM Computing Surveys
, 55(7), 1–38.
•
Byun, J., Kim, S., Sa, J., Kim, S., Shin, Y. T., & Kim, J. B. (2016). Smart city implementation models based on IoT
technology.
Advanced Science and Technology Letters
, 129(41), 209-212.
•
Elazhary, H. (2019). Internet of Things (IoT), mobile cloud, cloudlet, mobile IoT, IoT cloud, fog, mobile edge, and
edge emerging computing paradigms: Disambiguation and research directions.
Journal of network and computer
applications
, 128, 105-140.
Your preview ends here
Eager to read complete document? Join bartleby learn and gain access to the full version
- Access to all documents
- Unlimited textbook solutions
- 24/7 expert homework help
References
•
Gupta, B. B., & Quamara, M. (2020). An overview of Internet of Things (IoT): Architectural aspects,
challenges, and protocols. Concurrency and Computation:
Practice and Experience,
32(21), e4946.
•
Hwang, D., & Choi, Y. (2018). Internet of Things (IoT): Research trends, challenges, and future directions.
Journal of Electrical Engineering and Electronic Technology
, 7(2), 1-7.
•
Javaid, M., Haleem, A., Singh, R. P., Rab, S., & Suman, R. (2021). Upgrading the manufacturing sector via
applications of the industrial Internet of Things (IoT).
Sensors International
, 2, 100129.
References
•
Kim, D. S., Charpentier, P., Krommenacker, and Tran-Dang, H. (2020). Perspectives and difficulties about the internet of things for the physical internet.
Journal of the IEEE Internet of Things
, 7(6), 4711–4736.
•
Kumar, S., Tiwari, P., & Zymbler, M. (2019). Internet of Things is a revolutionary approach for future technology enhancement: a review.
Journal of Big
data
, 6(1), 1-
•
Sadeeq, M. A., &Zeebaree, S. (2021). Energy management for the Internet of Things via distributed systems.
Journal of Applied Science and Technology
Trends
, 2(02), 59-71.
•
Zanella, A., Bui, N., Castellani, A., Vangelista, L., & Zorzi, M. (2014). Internet of Things for Smart Cities.
IEEE Internet of Things Journal
, 1(1), 22-32.
•
•
Related Questions
Please answer question 1C, 1D with details on why its true or false. Please make handwriting legible. Thank you
arrow_forward
build an Arduino project that solves a real-world problem. This can be anything such asa motion detector, alarm, temperature reader, etc. It can be as simple or as complicated as youwould.
NameDetail1. What problem does it solve?2. What improvements can be made to your device?3. Research: Is there a device that already solves this problem? (it’s ok if there is, how isit different and/or similar?)4. Hardware Parts Used:5. Photo or Screenshot of your Project (top view, pin layout should be clear):
6. Code (minimal 25 lines of code)://code goes here
arrow_forward
Q3 Figure Q3 shows a functional block diagram of a digital circuit.
Уз
y2
Yo
X3
X2
C3
C2
FA
FA
FA
FA
$3
$2
So
V
N
(overflow)
(negative)
(zero)
Figure Q3
(a) Name the circuit and explain the operation of the given FBD.
(b) Discuss the importance of this circuit in digital hardware.
arrow_forward
can someone explain how to do this problem step by step
arrow_forward
Explain the term “four-quadrant operation” in the context of power electronicconverters. Explain why it is important in EVs and how it can be implemented. Usesketches and drawings to support your answer
arrow_forward
Don't use ai to answer I will report you answer
arrow_forward
Hello expert, I need a solution for the choices,
but I need you to clarify the correct choice by
drawing a diagram or image of the EMU8086
processor.
Q1:Choose the correct answer for the following sentences
1. The 8086 microprocessor has ---- data pin's
2. The 8086 microprocessor has
d. 32
a. 8 b. 16
c. 20
a. 16 b. 8
c. 32 d. 20
3. SP &
a. BP b. IP
address pin's
used with stack segment register (SS) to access memory within the stack segment
c. SI d. DI
4. If CS= 002AH and IP- 0023H, the physical address is -----
a. 002C3H b. 0023H1 c. 002A1H d. 0023H
5. POP instruction used to
a. Read b. Write c. Reset
the stack
d. Set
6. PIC16F877A microcontroller has ------ digital I/O ports
a. 2 b. 4 c. 5 d. 8
7. PIC16F877A microcontroller has analog multiplexed ports
b. 2 b. 4 c. 5 d. 8
8.
used to control the brightness of LED connected to Arduino
a. Analog input b. analog output c. digital input d. digital output
9. The content of SP -------when execute PUSH instruction
a. Increased by 2 b.…
arrow_forward
a) Buses are the conductive channels found in the circuitry of a microprocessor. This means that there is potential for current to flow among the various parts. Expertly classify these buses according to their function and describe how they interact with microprocessors.b) In a microprocessor, what is the distinction between data and instructions?
arrow_forward
Using any design approach, plan and design the following problem:
(show the Block Diagram, Truth Table, Simplification Approach Solution, Circuit Diagram)
2. Simple Security System
A simple security system for two doors consists of a card reader and a keypad.
Cand Reader
To Door
To Dor 2
To Alam
Logic
Circuit
D
E
Keypad
A person may open a particular door if he or she has a card containing the corresponding
code and enters an authorized keypad code for that card. The outputs from the card reader
are as follows:
A B
No card inserted
Valid code for door 1 0 1
0 0
Valid code for door 2 1 1
Invalid card code
10
To unlock a door, a person must hold down the proper keys on the keypad and, then, insert
the card in the reader. The authorized keypad codes for door 1 are 101 and 110, and the
authorized keypad codes for door 2 are 101 and 011. If the card has an invalid code or if the
wrong keypad code is entered, the alarm will ring when the card is inserted. If the correct
keypad code is…
arrow_forward
an someone explain to me the logic how to do this problem step by step I have the answers but I want to know how to do each part and understand the concepts of what is happening
in the circuit shown below
arrow_forward
Please answer the question in the image with reason/detail. Thank you
arrow_forward
(CONT.) (Sequential Logic) Complete the timing diagrams for the following
devices. To simplify your answer, assume that the devices have propagation, setup,
and hold time delays of zero and that asynchronous inputs have higher priority
than synchronous inputs.
Complete the timing diagram by drawing the waveforms for the two outputs for
the following device. To simplify your answer, assume that the device has
propagation, setup, and hold time delays of zero.
Preset
Reset
G
Preset
Reset
D.
arrow_forward
SEE MORE QUESTIONS
Recommended textbooks for you
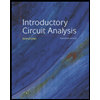
Introductory Circuit Analysis (13th Edition)
Electrical Engineering
ISBN:9780133923605
Author:Robert L. Boylestad
Publisher:PEARSON
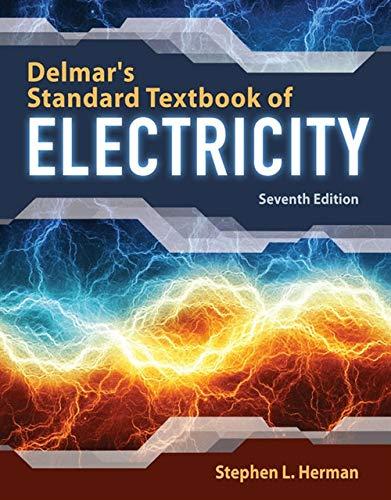
Delmar's Standard Textbook Of Electricity
Electrical Engineering
ISBN:9781337900348
Author:Stephen L. Herman
Publisher:Cengage Learning
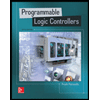
Programmable Logic Controllers
Electrical Engineering
ISBN:9780073373843
Author:Frank D. Petruzella
Publisher:McGraw-Hill Education
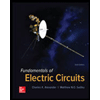
Fundamentals of Electric Circuits
Electrical Engineering
ISBN:9780078028229
Author:Charles K Alexander, Matthew Sadiku
Publisher:McGraw-Hill Education
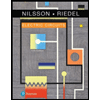
Electric Circuits. (11th Edition)
Electrical Engineering
ISBN:9780134746968
Author:James W. Nilsson, Susan Riedel
Publisher:PEARSON
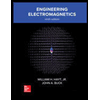
Engineering Electromagnetics
Electrical Engineering
ISBN:9780078028151
Author:Hayt, William H. (william Hart), Jr, BUCK, John A.
Publisher:Mcgraw-hill Education,
Related Questions
- Please answer question 1C, 1D with details on why its true or false. Please make handwriting legible. Thank youarrow_forwardbuild an Arduino project that solves a real-world problem. This can be anything such asa motion detector, alarm, temperature reader, etc. It can be as simple or as complicated as youwould. NameDetail1. What problem does it solve?2. What improvements can be made to your device?3. Research: Is there a device that already solves this problem? (it’s ok if there is, how isit different and/or similar?)4. Hardware Parts Used:5. Photo or Screenshot of your Project (top view, pin layout should be clear): 6. Code (minimal 25 lines of code)://code goes herearrow_forwardQ3 Figure Q3 shows a functional block diagram of a digital circuit. Уз y2 Yo X3 X2 C3 C2 FA FA FA FA $3 $2 So V N (overflow) (negative) (zero) Figure Q3 (a) Name the circuit and explain the operation of the given FBD. (b) Discuss the importance of this circuit in digital hardware.arrow_forward
- can someone explain how to do this problem step by steparrow_forwardExplain the term “four-quadrant operation” in the context of power electronicconverters. Explain why it is important in EVs and how it can be implemented. Usesketches and drawings to support your answerarrow_forwardDon't use ai to answer I will report you answerarrow_forward
- Hello expert, I need a solution for the choices, but I need you to clarify the correct choice by drawing a diagram or image of the EMU8086 processor. Q1:Choose the correct answer for the following sentences 1. The 8086 microprocessor has ---- data pin's 2. The 8086 microprocessor has d. 32 a. 8 b. 16 c. 20 a. 16 b. 8 c. 32 d. 20 3. SP & a. BP b. IP address pin's used with stack segment register (SS) to access memory within the stack segment c. SI d. DI 4. If CS= 002AH and IP- 0023H, the physical address is ----- a. 002C3H b. 0023H1 c. 002A1H d. 0023H 5. POP instruction used to a. Read b. Write c. Reset the stack d. Set 6. PIC16F877A microcontroller has ------ digital I/O ports a. 2 b. 4 c. 5 d. 8 7. PIC16F877A microcontroller has analog multiplexed ports b. 2 b. 4 c. 5 d. 8 8. used to control the brightness of LED connected to Arduino a. Analog input b. analog output c. digital input d. digital output 9. The content of SP -------when execute PUSH instruction a. Increased by 2 b.…arrow_forwarda) Buses are the conductive channels found in the circuitry of a microprocessor. This means that there is potential for current to flow among the various parts. Expertly classify these buses according to their function and describe how they interact with microprocessors.b) In a microprocessor, what is the distinction between data and instructions?arrow_forwardUsing any design approach, plan and design the following problem: (show the Block Diagram, Truth Table, Simplification Approach Solution, Circuit Diagram) 2. Simple Security System A simple security system for two doors consists of a card reader and a keypad. Cand Reader To Door To Dor 2 To Alam Logic Circuit D E Keypad A person may open a particular door if he or she has a card containing the corresponding code and enters an authorized keypad code for that card. The outputs from the card reader are as follows: A B No card inserted Valid code for door 1 0 1 0 0 Valid code for door 2 1 1 Invalid card code 10 To unlock a door, a person must hold down the proper keys on the keypad and, then, insert the card in the reader. The authorized keypad codes for door 1 are 101 and 110, and the authorized keypad codes for door 2 are 101 and 011. If the card has an invalid code or if the wrong keypad code is entered, the alarm will ring when the card is inserted. If the correct keypad code is…arrow_forward
- an someone explain to me the logic how to do this problem step by step I have the answers but I want to know how to do each part and understand the concepts of what is happening in the circuit shown belowarrow_forwardPlease answer the question in the image with reason/detail. Thank youarrow_forward(CONT.) (Sequential Logic) Complete the timing diagrams for the following devices. To simplify your answer, assume that the devices have propagation, setup, and hold time delays of zero and that asynchronous inputs have higher priority than synchronous inputs. Complete the timing diagram by drawing the waveforms for the two outputs for the following device. To simplify your answer, assume that the device has propagation, setup, and hold time delays of zero. Preset Reset G Preset Reset D.arrow_forward
arrow_back_ios
arrow_forward_ios
Recommended textbooks for you
- Introductory Circuit Analysis (13th Edition)Electrical EngineeringISBN:9780133923605Author:Robert L. BoylestadPublisher:PEARSONDelmar's Standard Textbook Of ElectricityElectrical EngineeringISBN:9781337900348Author:Stephen L. HermanPublisher:Cengage LearningProgrammable Logic ControllersElectrical EngineeringISBN:9780073373843Author:Frank D. PetruzellaPublisher:McGraw-Hill Education
- Fundamentals of Electric CircuitsElectrical EngineeringISBN:9780078028229Author:Charles K Alexander, Matthew SadikuPublisher:McGraw-Hill EducationElectric Circuits. (11th Edition)Electrical EngineeringISBN:9780134746968Author:James W. Nilsson, Susan RiedelPublisher:PEARSONEngineering ElectromagneticsElectrical EngineeringISBN:9780078028151Author:Hayt, William H. (william Hart), Jr, BUCK, John A.Publisher:Mcgraw-hill Education,
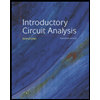
Introductory Circuit Analysis (13th Edition)
Electrical Engineering
ISBN:9780133923605
Author:Robert L. Boylestad
Publisher:PEARSON
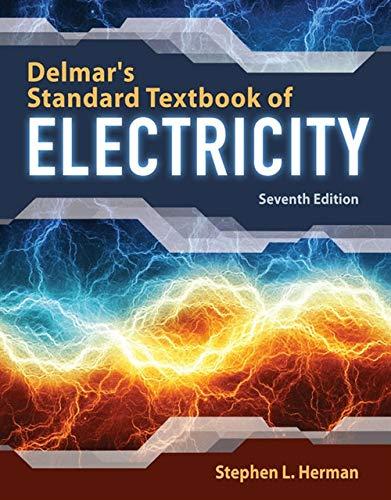
Delmar's Standard Textbook Of Electricity
Electrical Engineering
ISBN:9781337900348
Author:Stephen L. Herman
Publisher:Cengage Learning
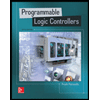
Programmable Logic Controllers
Electrical Engineering
ISBN:9780073373843
Author:Frank D. Petruzella
Publisher:McGraw-Hill Education
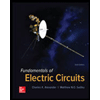
Fundamentals of Electric Circuits
Electrical Engineering
ISBN:9780078028229
Author:Charles K Alexander, Matthew Sadiku
Publisher:McGraw-Hill Education
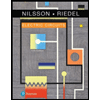
Electric Circuits. (11th Edition)
Electrical Engineering
ISBN:9780134746968
Author:James W. Nilsson, Susan Riedel
Publisher:PEARSON
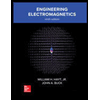
Engineering Electromagnetics
Electrical Engineering
ISBN:9780078028151
Author:Hayt, William H. (william Hart), Jr, BUCK, John A.
Publisher:Mcgraw-hill Education,