Exam Notes (2)
docx
keyboard_arrow_up
School
Carleton University *
*We aren’t endorsed by this school
Course
2200
Subject
Computer Science
Date
Jan 9, 2024
Type
docx
Pages
74
Uploaded by GrandSummer12878
xCOMS 2200: Group Exam Notes
WEEK 1: MEASURING AND COUNTING
-
September 6, 2023
SUMMARY
-
focuses upon the emergence of metrology as an art, craft, a science as well as a political
act. -
Discussion about why we count and the process of datafication.
DATA NOW BIGGER AND BETTER! THE SECRET LIFE OF BIG DATA
GENEVIEVE BELL
LINK
https://reserves.library.carleton.ca/LMSweb//ares.dll?
SessionID=K030018680D&Action=10&Type=10&Value=408986
NOTES
Socio-technical imagination
-
Technological history
-
Space-age visions of the future
-
Popular debate over privacy and the indifference about the surrender of vast quantities of information
Defining Big Data
-
Today's "big data" is made up of the same three things
-
facts (data)
-
frameworks (visualizations and analytics) -
extracted value (algorithms)
-
Macho talk about how big, fast and multitasking data can be: these ideas are as old as still dominant forms of Euro-American masculinity. -
notion that those big sets of data might' frame the way we think; how we are identified, how we identifY ourselves-these ideas are not new
Chinese invention of bureaucracy
-
Creating formulation for managing what was an ever-increasing trove of material
-
Notion of big sets of data is not new
Will everything produce data?
-
everything is going to produce data.
-
There are a lot of reasons why that might not be a useful thing or why, on the other hand, it might be potentially a very useful thing.
-
QUESTION- how do we understand the framing of a popular argument in the tech community that says that everything will be or will produce data? -
What does that mean about the notion of production, about notions of objects and subjects, and about notions of data?
-
A future in which everything is "connected" and producing data is both overwhelming and spectacularly interesting. -
It signals both serious plenitude and networked singularity.
-
The quantified self is a big deal
-
Everything can produce data depending on devices Will everyone produce data?
-
We live in a world where the internet and it forms of connectivity are remarkably unevenly distributed.
-
This is due to politics and economics: the pricing structures of the internet are different in different places. -
Certain governments regulate the internet in different ways, in terms of content, availability, appropriate forms of activity, and appropriate in-points. -
The adoption of devices is remarkably different in different places in the hands of different people. -
It is also due to infrastructure: in some places, the internet is strongly gated for downloads versus uploads.
-
Undersea cables move data at different rates between different countries. -
There are already hot spots of data production and then big vast empty gaps
-
Some people are producing more data than others. -
Some data appear to be from one human organism, but it may actually be from a whole collection of people.
-
more data there would be, the more truth we would have.
-
Everyone would produce data, thus we would understand everyone better. -
the ways, that data is produced, coded and understood is always being read through a series of cultural lenses
-
Who has agency to produce data, who has agency to control data, who even knows if there is data out there about them?
-
Maybe not- the digital divide means ppl cannot create data equally
What does data want? And what is wanted from it?
-
Data wants more data, and 14 if more data wants even more data then soon all that data wants an algorithm and then several algorithms.
-
Big data wants the accumulation of more of itself.
-
What is going on right now that makes us say we need data?
Data keeps it real
-
virtuality permits its infinity
-
a whole lot of data has physical manifestations and physical representations
-
I want to leave something behind
-
Not all data is going to be invisible. Some data is going to be physically
represented in objects. -
No missed context/background information sometimes
Data loves a good relationship
-
Most data doesn't exist in isolation. -
As a human being engaged in anything on the internet, chances are you have done something that is making someone or something that collects
data absolutely crazy:
-
you have more than one person logged into your Amazon account, more than one person uses your iTunes account, -
you are sharing your Netflix password with people who don't live in your house
-
At every single one of those points you look like an individual. -
You are someone who confuses the Amazon recommendation engine
-
We have any number of digital traces that don't actually connect
except through our bodies. -
All the things we know about social power, hierarchy, notions of what talks to what, in what manner and why apply.
Data doesn't always have the best network
-
Not all data is created equal to flow on the network
-
Undersea cables that are controlled by 3rd parties
-
Depending on the physical gateways that data is running through, some governments and private enterprises can have a look inside, creating
Your preview ends here
Eager to read complete document? Join bartleby learn and gain access to the full version
- Access to all documents
- Unlimited textbook solutions
- 24/7 expert homework help
even more data, and also determining what data does and doesn't flow on the network.
-
Video moves differently than text. Photos move differently than sound. Television and content move in a completely different formulation than
all of those things. -
There are arguments about how the network has been architected and scaled that suggest that certain sorts of data are going to be more effective than others
-
So imagining all the data will move freely everywhere in. a kind of universal moment of splendor may not be the case
Data has a country
-
It is already produced under certain policy regimes.
-
Medical information is created in a certain kind of way to comply with state regulations. -
Data has things on its proverbial body that will tell us where it came from
-
if you know what you're looking for, you'll be able to see that place and
those styles. -
There will be the clues and the traces of its country, its point of origin, the moment that it appeared. There may also be traces of its travels. -
Countries with strong ideologies
Data is feral -
data is going to have the capacity to defy the expectations of the places where it was created and traveled, and will start to have another life. It will be repurposed.
-
Algorithms create new forms of data, and that data finds lives in unexpected places
-
Some of the data that is produced in one format will be used in a different one to do something completely different
-
Most technology doesn't end up in the hands of the people it was anticipated for, doing the work it was anticipated to do. -
People imagine that we are going to connect data sets to the internet, that many devices are going to create data and that somehow all of that data is going to stay stunningly controlled and doing exactly what it is told.
-
In the hands of corporations it leads to coupons! And muted, if audible at all, political critique
-
Once they are there- it is hard to come back
Data has Responsibilities
-
custodians of those stories, they are also the custodians of the responsibility of doing the right thing with those stories. -
Some of the responsibilities I suspect will not be about shutting the conversation down, but about opening it up
-
If the notion is that some data is meant to be told, then other data probably has a story that isn't imperative
-
What would it mean to architect a system where big data had responsibilities? -
Laws, regulations, ethics
-
Every person is represented in datasets
Data keeps it messy but likes to look good
-
Data will be messy
-
There will be data sets produced by the same person on parallel devices
that look completely different from each other. -
There will be data produced on the same human being in the same moment that will suggest conflicting ideas that may reveal two completely different realities and truths
-
The temptation is going to be to constantly tidy up the data, to neaten up the data, to make it fit the algorithm
-
Data is also never going to be a complete set
-
most of this data is being either directly produced by human beings or produced by devices, services, and applications that were created by human beings and evep the algorithms doing the work were ultimately created by human beings and even when the algorithms go feral and create their own algorithms those will all somewhere encode entanglements of human and non-human thought, assumptions, cognition, ideas, responsibilities, cultural practices. -
Partial truths
Data doesn't last forever
-
Snapchat allows people to share images and words but does not build an archive of those exchanges on its servers and does not permit storage
on people's individual phones. -
What does it mean to imagine the data that's effectively unsayable?
-
Disappear
-
lost
What are the next questions?
-
data wants, it wants to be real, it wants a country and a responsibility, it's going to be feral, and messy. It's going to want to last forever and be
ephemeral and tell lies and look good all at the same time
How to read an algorithm
-
cultural objects that are a little hard for most of us to 23 read in quite the ways we know how to read an object or a story. To read them as objects, as practices, as moments is more complicated
-
it's going to require if not retooling, then certainly engagement with a different community
-
It's time to learn some math, some coding, and to actually engage with some programmers. -
Learn about algorithms and alchemists- who are these people and what do they do?
Studying the new priest and alchemists
-
you should trust the quants and the geeks even though near as I could tell they were exactly the same people as the priests and the alchemists, just in a different company and contexts
Critiquing the new empiricism and countering it with our own
-
some data didn't equal truth, but more data absolutely equaled truth. -
why it is that the language around big data and its social-technical imaginary involves such a remarkable reassertion of empiricism.
-
passionate conversations about all the things that to my mind have always mattered: gender, race, class, sexuality, history, nationality, and oh, by the way, power that runs through all of this. -
especially important as critical social scientists and humanists to stay true to the big data, too. Again, this is going to involve some retooling and making friends with the custodians and alchemists, geeks and quants. -
as soon as anyone asserts that data equals truth, you know there is a whole other set of conversations we should be having
-
What is it about big data as an aspiration, as a socio-technical category, as a point on the map of our cultural development-
what is the work that it is doing for us right now?
-
socio-technical imagination. This also entails asking, "what does it mean to have that imagination?"
Your preview ends here
Eager to read complete document? Join bartleby learn and gain access to the full version
- Access to all documents
- Unlimited textbook solutions
- 24/7 expert homework help
Musée des Arts et Métiers, 2018, Made to Measure: The World’s 7 Units, Catalogue d'exposition, Bureau International des Poids et Mesures et Laboratoire National de Métrologie et d'Essais (LNE), Flammarion.
LINK
NOTES
LECTURE NOTES
WEEK 1- SEPT. 6- INTRODUCTION- WHY DO WE MEASURE AND COUNT YOU?
Pre-class introduction
-
We will get to know each other, and discuss what the course is about and the expectations and objectives of the class. -
The 1st lecture is about the emergence of metrology as an art, craft, science as well as a political act. -
We then proceed with a discussion about why we count and the process of datafication. -
Datafication:
-
A technological trend -
Turns many aspects of our life into data which is subsequently transferred into information realized as a new form of value.
-
refers to the collective tools, technologies, and processes used to transform an organization to a data-driven enterprise. -
This buzzword describes an organizational trend of defining the key to core business operations through a global reliance on data and its related infrastructure.
-
The 1st in-class activity involves applying critical data studies observation techniques to a set of indicators about mass shootings.
Bell, Genevieve, 2015, The Secret Life of Big Data, Chapter 2 in Boellstorff, T. and Maurer, B.
Eds. In Data, Now Bigger and Better, Paradigm Press.
Introduction
-
How do we start talking about the socio-technical imagination
-
Popular debate hovers between concerns over privacy and government snooping, and
indifference about the surrender of vast quantities of information just by turning on one's phone
-
arguments about how to define big data.
-
Today's "big data" is made up of the same three things:
-
facts (data)
-
frameworks (visualizations and analytics) -
extracted value (algorithms).
-
the difference is the awe-inspiring scale made possible through computation (mathematical calculation)
-
the Chinese government - notions of big data sets are not new, the notion that big sets
of data might frame the way we think; how we are identified, how we identify ourselves-these ideas are not new
-
creating a formulation for managing what was an ever-increasing trove of material. -
Its files have existed for hundreds if not at this point thousands of years.
Will everything produce data?
-
everything is going to produce data.
-
There are a lot of reasons why that might not be a useful thing or why, on the other hand, it might be potentially a very useful thing.
-
QUESTION- how do we understand the framing of a popular argument in the tech community that says that everything will be or will produce data? -
What does that mean about the notion of production, about notions of objects and subjects, and about notions of data?
-
A future in which everything is "connected" and producing data is both overwhelming and spectacularly interesting. -
It signals both serious plenitude and networked singularity.
-
Quantified self is a big deal
-
Everything can produce data depending on devices Will everyone produce data?
-
We live in a world where the internet and it forms of connectivity are remarkably unevenly distributed.
-
This is due to politics and economics: the pricing structures of the internet are different in different places. -
Certain governments regulate the internet in different ways, in terms of content,
availability, appropriate forms of activity, and appropriate in-points. -
The adoption of devices is remarkably different in different places in the hands of different people. -
It is also due to infrastructure: in some places, the internet is strongly gated for downloads versus uploads.
-
Undersea cables move data at different rates between different countries. -
There are already hot spots of data production and then big vast empty gaps
-
Some people are producing more data than others. -
Some data appear to be from one human organism, but it may actually be from
a whole collection of people.
-
more data there would be, the more truth we would have.
-
Everyone would produce data, thus we would understand everyone better. -
the ways, that data is produced, coded and understood is always being read through a series of cultural lenses
-
Who has agency to produce data, who has agency to control data, who even knows if there is data out there about them?
-
Maybe not- the digital divide means ppl cannot create data equally
What does data want? And what is wanted from it?
-
Data wants more data, and 14 if more data wants even more data then soon all that data wants an algorithm and then several algorithms.
-
Big data wants the accumulation of more of itself.
-
What is going right now that makes us say we need data?
Data keeps it real
-
virtuality permits its infinity
-
a whole lot of data has physical manifestations and physical representations
-
I want to leave something behind
-
Not all data is going to be invisible. Some data is going to be physically represented in objects. -
No missed context/background information sometimes
Data loves a good relationship
-
Most data doesn't exist in isolation. -
As a human being engaged in anything on the internet, chances are you have done something that is making someone or something that collects data absolutely crazy:
-
you have more than one person logged into your Amazon account, more than one person uses your iTunes account, -
you are sharing your Netflix password with people who don't live in your house
-
At every single one of those points you look like an individual. -
You are someone who confuses the Amazon recommendation engine
-
We have any number of digital traces that don't actually connect except through our bodies. -
All the things we know about social power, hierarchy, notions of what talks to what, in what manner and why apply.
Data doesn't always have the best network
-
Not all data is created equal to flow on the network
-
Undersea cables that are controlled by 3rd parties
-
Depending on the physical gateways that data is running through, some governments and private enterprises can have a look inside, creating even more data, and also determining what data does and doesn't flow on the network.
-
Video moves differently than text. Photos move differently than sound. Television and content moves in a completely different formulation than all of those things. -
There are arguments about how the network has been architected and scaled that suggests that certain sorts of data are going to be more effective than others
Your preview ends here
Eager to read complete document? Join bartleby learn and gain access to the full version
- Access to all documents
- Unlimited textbook solutions
- 24/7 expert homework help
-
So imagining all the data will move freely everywhere in. a kind of universal moment of
splendor may not be the case
Data has a country
-
It is already produced under certain policy regimes.
-
Medical information is created in a certain kind of way to comply with state regulations.
-
Data has things on its proverbial body that will tell us where it came from
-
if you know what you're looking for, you'll be able to see that place and those styles. -
There will be the clues and the traces of its country, its point of origin, the moment that it appeared. There may also be traces of its travels. -
Countries with strong ideologies
Data is feral -
data is going to have a capacity to defY the expectations of the places where it was created and traveled, and will start to have another life. It will be repurposed.
-
Algorithms create new forms of data, and that data finds lives in unexpected places
-
Some of the data that is produced in one format will be used in a different one to do something completely different
-
most technology doesn't end up in the hands of the people it was anticipated for, doing
the work it was anticipated to do. -
people imagine that we are going to connect data sets to the internet, that many devices are going to create data and that somehow all of that data is going to stay stunningly controlled and doing exactly what it is told.
-
In the hands of corporations it leads to coupons! And muted, if audible at all, political critique
-
Once they are there- it is hard to come back
Data has Responsibilities
-
custodians of those stories, they are also the custodians of the responsibility of doing the right thing with those stories. -
Some of the responsibilities I suspect will not be about shutting the conversation down,
but about opening it up
-
If the notion is that some data is meant to be told, then other data probably has a story
that isn't imperative
-
What would it mean to architect a system where big data had responsibilities? -
Laws, regulations, ethics
-
Every person is represented in datasets
Data keeps it messy but likes to look good
-
Data will be messy
-
There will be data sets produced by the same person on parallel devices that look completely different from each other.
-
There will be data produced on the same human being in the same moment that will suggest conflicting ideas that may reveal two completely different realities and truths
-
The temptation is going to be to constantly tidy up the data, to neaten up the data, to make it fit the algorithm
-
Data is also never going to be a complete set
-
most of this data is being either directly produced by human beings or produced by devices, services, and applications that were created by human beings and evep the algorithms doing the work were ultimately created by human beings and even when the algorithms go feral and create their own algorithms those will all somewhere encode entanglements of human and non-human thought, assumptions, cognition, ideas, responsibilities, cultural practices. -
Partial truths
Data doesn't last forever
-
Snapchat allows people to share images and words but does not build an archive of those exchanges on its servers and does not permit storage on people's individual phones. -
What does it mean to imagine the data that's effectively unsayable?
-
Disappear
-
lost
What are the next questions?
-
data wants, it wants to be real, it wants a country and a responsibility, it's going to be feral, and messy. It's going to want to last forever and be ephemeral and tell lies and look good all at the same time
How to read an algorithm
-
cultural objects that are a little hard for most of us to 23 read in quite the ways we know how to read an object or a story. To read them as objects, as practices, as moments is more complicated
-
it's going to require if not retooling, then certainly engagement with a different community
-
It's time to learn some math, some coding, and to actually engage with some programmers. -
Learn about algorithms and alchemists- who are these people and what do they do?
Studying the new priest and alchemists
-
you should trust the quants and the geeks even though near as I could tell they were exactly the same people as the priests and the alchemists, just in a different company and contexts
Critiquing the new empiricism and countering it with our own
-
some data didn't equal truth, but more data absolutely equaled truth. -
why it is that the language around big data and its social-technical imaginary involves such a remarkable reassertion of empiricism.
-
passionate conversations about all the things that to my mind have always mattered: gender, race, class, sexuality, history, nationality, and oh, by the way, power that runs
through all of this. -
especially important as critical social scientists and humanists to stay true to the big data, too. Again, this is going to involve some retooling and making friends with the custodians and alchemists, geeks and quants. -
as soon as anyone asserts that data equals truth, you know there is a whole other set of conversations we should be having
-
What is it about big data as an aspiration, as a socio-technical category, as a point on the map of our cultural development-what is the work that it is doing for us right now?
-
socio-technical imagination. This also entails asking, "what does it mean to have that imagination?"
Musée des Arts et Métiers, 2018, Made to Measure: The World’s 7 Units, Catalogue d'exposition, Bureau International des Poids et Mesures et Laboratoire National de Métrologie
et d'Essais (LNE), Flammarion.
Metrology
-
Metrology:
the science of measurement & its application. -
Metrology includes all theoretical & practical aspects of measurement, whatever the measurement uncertainty & field of application
-
World Metrology Day- the meter became the standard
-
Units of measure took hundreds of years of refining before international agreement
-
A meter is a social construction
Measuring
-
“measuring, is merely comparing an unknown physical quantity, with a quantity of the same nature taken as a reference using an instrument” Himbert, p.23 in Made to Measure.
-
Fundamentally an immaterial operation, that transforms a mass, volume or temperature into a numeric value – but it does need a tangible object to do so!
-
Edme Régnier constructed a dynamometer in about 1790 that measured tension and pressure exerted by a person or animal.
Unit of measurement -
“is a particular representation of the quantity measured, taken as a reference and chosen by convention to express the results of measurement” p.25 in Made to Measure.
-
It is a social construction!
-
Put into practice to enable commercial exchanges, taxes, quantify currency, build buildings, develop transport, science and etc.
-
Measurement laid the foundation for science.
Your preview ends here
Eager to read complete document? Join bartleby learn and gain access to the full version
- Access to all documents
- Unlimited textbook solutions
- 24/7 expert homework help
Measuring is a social moderator
-
Before measuring became an act of science, it was intended to establish social justice and peace
-
Market want olive oil- how do you know we are getting a leater -
Fair trade exchange
-
Survival
-
Democratizing the market (town square where people traded product)
-
“is a response to the necessity of equitable distribution of resources among inhabitants
of the same place”… “is an act of power” and “an exercise in delimitation, a matter of decision rather than precision” p.45 in Made to Measure
Measuring as social control
-
We give authority to measurement, and there are authorities with the responsibility to ensure good measures!
-
Inspectors measuring kit- -
Metrology
-
Metrology Research Centre
-
Change of standards- measure is traceable
-
Monitoring, enforcing, ensuring..
-
Providing a continuous chain of standards and traceability
Proportions: principal of justice
-
The power of measurement provides control and regulation without recourse to moral considerations
-
A means to “equitably regulating relations between citizens in their relationships to community property, wealth and honors (responsibilities, posts, political appointments)” Aristotle on p.49 in Made to Measure
-
Morality and civic responsibility
-
Communal activity!
-
Found in public squares- people could measure themselves
Measures and order
-
The Allegory of Good and Bad Government is a series of three fresco panels painted by Ambrogio Lorenzetti between February 1338 and May 1339.
-
Ruling kingdom that are equitable, fair, clean, and generally population is happy
-
Countryside- farmers well managed- fair trade for what they produce- idealization
Measurement & In-direct knowledge
-
Measurement is based on “wide-ranging theoretical knowledge, …an array of extremely sophisticated instruments… and today’s high-capacity data processing capabilities” p.68 in Made to Measure
-
Made a measuring meter 1875- standard measure- went global- object sent
around the world
-
Training workers and industry and people about measuring- Industrial revolution
Dematerialization
-
It is equal to one ten-millionth of the length of the quadrant of the meridian between Dunkerque and Barcelona through the Paris Observatoire
-
The metre is adopted as a unit of measure & in June 1799 2 platinum standard metres
were created -
The metric system goes international in 1867 & a new metre prototype was created -
1875 the Metre Convention is signed
-
In 2019 the metre becomes:
-
symbol m, is the SI unit of length. It is defined by taking the fixed numerical value of the speed of light in vacuum c to be 299792458 when expressed in the unit m⋅s−1, where the second is defined in terms of the caesium frequency ΔνCs.
-
Now based “on the rules of nature to create the rules or measurement linking measurements at the atomic and quantum scales to those of the macroscopic level” Director of BIPM 2018
-
Now scientific construct- physics- measured by the speed of light now
Datafication
-
Datafication:
the process of taking information about all things under the sun…and transforming it into a data format to make it quantified
-
“render into data many aspects of the world that have never been quantified before” (Cukier & Mayer-Schoenberger, 2013, p. 29)
-
“To datafy a phenomenon is to put it in a quantified format so it can be tabulated and analyzed” and “to datafy, we need to know how to measure and how to record what we measure” Mayer-Schonbeger and Cukier p.78
-
To transform things that we think cannot be measured into things that can be measured
The Secret life of Big Data!
-
Genevieve Hall 2015
-
Dynasties in China- best admins of big data
-
Domes Day book 1086- king sent out people into society to document everything
-
To understand how the kingdom was doing
-
Males- for war
-
Bounty derived from farming and lords in taxation
-
First time that a major count of that nature was taken
-
Recreated 100 years later and done by a social science project in British Society and produced on these discs
-
Domesday reloaded- view info on book
-
John Talon Building
-
Took the first census called census in the world in 1666 in Canada
-
Sent back to France
QUESTIONS
1.
Will everything produce data?
2.
Will everyone produce data?
3.
What do data want?
4.
Data keep it real?
5.
Data love good relationships
-
Smart Cities, Internet of things
-
Phones, toaster, refrigerators, bodies, etc. Does that make you an objects that produces data? Are you the subject being studied by an object or a system?
-
Do we let everything produce data? Data production? Objects? Subjects? Networked singularity?
-
Digital divide? Uneven networks, regulation and law, gated internet, infrastructure and networks of cables, will there be more truth? Who reads the data, how has the truth? How are they coded? Agency? Ethnography of data.
-
Would data have a wish? A psyche? Data want more data and algorithms to make more data, what is this new empirical turn? Being seduced by data?
-
Data are also material, they are not virtual. Data become things, actions, objects? If they are open they also speak about things.
-
We are always sharing our data? My data develops a relationship with your data. Algorithms are helping you make decisions with recommendations and thus connecting you with things or more data. Coders and algorithm writers are
making the connections and data are interoperable or linked
6.
Data dont always have the best network
7.
Data have a country
8.
Data are feral
9.
Data have responsibilities
10. Data keep it messy but like to look good
-
Data need an infrastructure and the infrastructure is uneven, there are gateways, hops, stores, flow controls, some are throttled, environment from faraday cages to rain
as it relates to Wi-Fi
-
Countries have policies, laws, regulations, source, a geography, has a style
-
Data are repurposed, reused and repackaged this data are hard to control, sneaks up on you when you least expect them to, they do unanticipated and unexpected things, it
sneaks past the firewall, it gets hacked, data can be misuded, think of the Nazis!
-
Stories and secrets, ethics, values, licences, copyright, privacy, -
Incomplete data, platforms and software change how they look, they tell different stories to different people, there are errors, data lie to look good, think of online dating,
sometimes algorithms lie
11. Data dont last forever
Your preview ends here
Eager to read complete document? Join bartleby learn and gain access to the full version
- Access to all documents
- Unlimited textbook solutions
- 24/7 expert homework help
12. How to read algorithms
13. Studying the new priests and alchemists
14. Critiquing the new empiricism and countering it with out own
-
Right to be forgotten, data disappear, snapchat, bad management and poor archiving, Aborigines and the names of those who died, and some cultures pass the names along for generations
-
Algorithms are cultural objects that we need to learn to read them, coding, math and the ability to interrogate
-
Quants and geeks, coders and programmers, data live here but algorithms are there?
-
More data, therefore more facts, thus more truth, we will understand people better, people are data and data are people!
-
We need to bring more theory to the game, and critical thinking in the arena,
WEEK 2: WHAT ARE DATA?
-
September 13
SUMMARY
-
Definition of data -
Data characteristics -
Data as the bedrock of information, knowledge and wisdom -
Socio-technical processes
Kitchin, R. 2022, Introducing Data, Chapter 1 in the Data Revolution: A Critical Analysis of Big Data, Open Data and Data Infrastructures. 2nd Edition, Sage.
LINK
https://reserves.library.carleton.ca/LMSweb/ares.dll?
SessionID=C061108907P&Action=10&Type=10&Value=410399
NOTES
Data Definition:
Data are representative pieces of information about phenomena and the input for and output from computational processes reflecting some aspect of the real world or experiments (4). ➔
What constitutes data started to become defined by how they were generated, processed, and stored
Quantitative forms of data are more robust evidence than personal testimony (5)
How data are conceived and used varies between those who capture, analyse, and draw conclusions from them (6).
The Characteristics of Data
Form:
Quantitative being numeric and categorical or qualitative being words, images. (7)
Structure:
Structured
➔
Structured data are those that can be easily organized, stored, and transferred in a defined relational data model with a consistent format (8)
Semi-Structured ➔
Loosely structured data that has no predefined data model/schema (8)
Unstructured ➔
Do not have a defined data model or common identifiable structure (8)
➔
Unstructured data are usually qualitative in nature (8)
➔
Each individual element such as narrative text or photo may have a specific
structure but that structure is not shared throughout the data (8)
Source: Captured Data: ➔
Data can be captured directly through measurement like observation, survey labs, and field experiments (9)
Exhaust Data
➔
Is inherently produced by a device or system (9)
➔
Transient in nature (9)
◆
Is never examined, processed or stored because they are too voluminous or costly to handle
Captured and exhaustive data are considered raw
Derived Data
➔
Produced through additional processes or analysis of captured data (9)
◆
Captured data might be individual traffic counts through an intersection and derived would be a total number of counts or counts per hour (9)
➔
Derived data is data derived from captured and exhaust data (9)
Producer
Primary Data
➔
Generated by a researcher and their instruments within a research design of
their making (9)
Secondary Data
➔
Data made available to others to reuse and analyze that are made by
someone else (10)
➔
One person’s primary data can be another person’s secondary data (10)
Tertiary Data
➔
A form of derived data (10)
Type
Indexical Data ➔
Enable identification, linking ◆
Passports, social security numbers etc
Attribute Data
➔
Non-indexical data that represents aspects of a phenomenon (11)
◆
In respect to a person attribute data would be age, sex, height, weight etc Metadata
➔
Data about data (11)
➔
Metadata about content includes names and descriptions of specific fields (11)
➔
Descriptive metadata (11)
◆
Titles, authors, publishers, subject descriptions
➔
Structural metadata (11)
◆
Organisation and coverage of a dataset ➔
Administrative metadata (11)
◆
When and how dataset was created, details on technical aspects of it;s creation, file format etc
Data, information, knowledge, wisdom Three types of information
(12)
➔
Factual: Information as reality (patterns, fingerprints, tree rings)
➔
Instructional: Information for reality (commands, algorithms, recipes)
➔
Semantic: Information about reality (trian timetables, maps, biographies)
5 Issues of differences in definition
(13) ➔
Uncertainty
Your preview ends here
Eager to read complete document? Join bartleby learn and gain access to the full version
- Access to all documents
- Unlimited textbook solutions
- 24/7 expert homework help
➔
Physicality
➔
Structure/ process
➔
Intentionality ➔
Truth
Commodity Properities (13)
➔
Non-rivalrous: More than one entity can posses the same information ➔
Non-excludable: Easily shared and takes effort to limit sharing (paywalls)
➔
Zero-marginal costs: Once information is available, the cost of reproduction is often negligible
Limits and control to circulation
(13) ➔
Occurrence: discovering, designing, authoring
➔
Transmission: networking, distributing, accessing, retrieving
➔
Usage: Monitoring, modelling, analyzing, explaining
Critically framing data
Objectivity / Neutrality (14 -15)
➔
Sensors produce an objective, realist view of the world revealing things as they really are
➔
If data are seen as lacking neutrality or objectivity, then it is the people corrupting data and twisting it for their own ends ➔
A sensor has no politics or agenda
Data are not static
LECTURE NOTES
L2 - COMS 2200 Big Data & Society
WEEK 3: SMALL DATA AND YOU -
September 20th
SUMMARY
Anderson, B., 1991, Census, Map, and Museum; in Imagined Communities: Reflections on the Origin and Spread of Nationalism. Revised Edition, Verso, New York.
LINK
https://www-fulcrum-org.proxy.library.carleton.ca/epubs/dz010s66b?
locale=en#page=179
NOTES
This reading focuses exclusively on census making in Southeast Asia. ➔
Rapid, arbitrary series of changes in which categories are extraordinarily rapid superficially arbitrary changes but politically powerful identities categories always lead the list. 2 Principles Hirshchman draws from Malaysian Census
1.
As colonial period wore on, the census categories became exclusively racial (164)
2.
Religious identity gradually disappeared from the census (164)
Colonial powers separated the Malaysian identity into ‘Malay’, ‘Javanese’, ‘Sakai’, ‘Banjarese’ etc. (165)
Spanish influence in the Philippines
➔
Separated class categories (166)
◆
Principales, hidalogs, pecheros, and esclavos (prince, nobleman, commoner and slave)
➔
The real innovation of the census-takers of the 1870s was, therefore, not in the construction
of ethnic-racial classifications, but rather in their
systematic qualification
. (168)
➔
After 1850 colonial authorities were using increasingly sophisticated administrative means to enumerate populations (168)
◆
Including women and children who had been historically ignored
In Malaysia, ‘Islamic’ was treated as another name for ‘Malay’ (170)
➔
Making the country more homogeneous
Maps
Cosmograph (171)
➔
Formal symbolic representation of the Three Worlds of traditional
Buddhist cosmology
➔
Not horizontal like our own maps; rather a series of supraterrestial heavens and hells
Diagramatic Guides (171)
➔
Military campaigns, coastal shipping
➔
Organized roughly by quadrant
➔
Covering only terrestrial space
➔
These maps were always local
Two Avatars of Political Topography of the Map
Legal Transferability
➔
European-style maps worked on the basis of totalizing classification (173)
➔
As interlopers, europeans tried to legitimize the spread of their power via quasi-legal methods (174)
➔
The appearance of ‘historical maps’ were designed to demonstrate, in the new cartographic discourse in the late nineteenth century. (174)
Map-as-logo
➔
The practice of imperial states of colouring their colonies on maps with imperial dye (175)
◆
British were pink-red
◆
French were purple-blue
◆
Dutch were yellow-brown
➔
Colouring of the map led to a jigsaw effect (175)
➔
Detached from geographic and cultural context (175)
Museums The musemizing imagination are profoundly political (178)
➔
New museums in Indonesia postcolonialism reflected its immediate ancestor, the colonial Netherlands East Indies (178)
The commercial-colonial regimes of the two great East India Companies and the rise of the true modern colony, directly attached to the metropole (180)
➔
Archaeological efforts were on the restoration of imposing monuments (180)
◆
Coincided with the first political struggle over educational policies (180)
➔
The formal ideological programme of the reconstructions always placed
builders of the monuments and the colonial natives in a certain hierarchy (180)
Your preview ends here
Eager to read complete document? Join bartleby learn and gain access to the full version
- Access to all documents
- Unlimited textbook solutions
- 24/7 expert homework help
➔
Monuments of colonial powers show those left that “Our very presence shows that you have always been, or have long become incapable of greatness or self-rule. (181)
Progression 1.
Massive, technically sophisticated archaeological reports, complete with photographs, recording the process of reconstruction of particular distinct ruins (182)
2.
Illustrated books for public consumption (182)
3.
A general logoization including monuments (182)
Post-independent states exhibited marked continuities with their colonial predecessors inherited this form of political museumizing (183)
➔
On the 15th anniversary of Cambodia’s independence a large wood papier-mâche replica of an ancient temple was displayed at the national sports stadium
Library and Archives Canada (LAC). 2022. Historical Language Advisory: Notice about the Collection. August 23, 2022. https://library-
archives.canada.ca/eng/Pages/notices-collection.aspx.
LINK
NOTES
LECTURE NOTES
L3 COMS 2200 - Small Data and You
WEEK 4: BIG DATA AND YOU
-
September 30th
Your preview ends here
Eager to read complete document? Join bartleby learn and gain access to the full version
- Access to all documents
- Unlimited textbook solutions
- 24/7 expert homework help
SUMMARY
Lupton, D. 2017, ‘Know Thyself’ Self-Tracking Technologies and Practices, Chapter 1 in The Quantified Self, Polity.
LINK
ARES
NOTES
REFLECTION
LECTURE NOTES
WEEK 5: DATA INFRASTRUCTURE
-
October 4
SUMMARY
Holt, J. and Vondereau, P. 2015, “Where the Internet Lives”: Data Centers as Cloud Infrastructure, Chapter 3 in Signal Traffic: Critical Studies of Media Infrastructures eds. By L. Parks and N. Starosielski.
LINK
NOTES
Your preview ends here
Eager to read complete document? Join bartleby learn and gain access to the full version
- Access to all documents
- Unlimited textbook solutions
- 24/7 expert homework help
Hogan, Mél, Dustin Edwards, and Zane Griffin Talley Cooper. 2022. “5 Things about Critical Data Center Studies.” Commonplace, October. https://doi.org/10.21428/6ffd8432.af5934aa.
LINK
NOTES
News Article
Glanz, James. 2012. “Power, Pollution and the Internet.” The New York Times, September 23, 2012, sec. Technology. https://www.nytimes.com/2012/09/23/technology/data-centers-waste-vast-
amounts-of-energy-belying-industry-image.html.
LINK
NOTES
LECTURE NOTES
L5 COMS 2200 - Infrastructure
WEEK 6: DATA INSTITUTIONS AND YOU -
October 11
SUMMARY
Your preview ends here
Eager to read complete document? Join bartleby learn and gain access to the full version
- Access to all documents
- Unlimited textbook solutions
- 24/7 expert homework help
Lauriault, T. P. 2022, Looking Back Toward a “Smarter” Open Data Future, Chapter 1 in The Future of Open Data edited by P. Robinson and T.
Scassa, University of Ottawa Press. (Open Access version of the book available here https://press.uottawa.ca/the-future-of-open-data-4542.html).
LINK
NOTES
KEY QUOTES
-
“open data, and how it is technologically conceptualized, might be too narrow a focus, and instead calls for the adoption of a broader and more integrated openness approach, especially as open data are being subsumed by smart systems or digital twins”
-
“ the future of open data requires looking back at th eepistemic groups involved in its creation, overcoming its technological legacy to ensure that when smart systems and digital twins come online, they do not suffer the same fate in terms of quality and a lack of systems thinking”
-
“a broader concept of openness be adopted, especially if there is to be an integrated and systems-based approach to smart systems, as seen in the case of the emerging open smart city.”
-
“propose that if we want open data-driven decision making, we will have to think about openness more broadly; to govern data as more than simply technical objects and, instead, reconceptualize them as open social and technical processes.
Abstract
-
History of data sharing
-
Tracing Canada’s data sharing genealogy
-
To demonstrate that epistemic groups, institutions, materialities, and legalities have uniquely shaped the practice
-
Open data is a discursive regime
-
Critical data-studies approach
-
Social and technical data assemblage framework
-
Data do not exist independently from the context within which they were created and the systems and processes that produce them
-
Moving beyond the narrow technological conceptions of open data seen in most definitions
-
As “ large “smart” social and technological systems (Hughes, 1987) are built, such as smart cities, smart grids, or digital twins”
-
Greater attention to epistemic groups and their subjectivities
-
Avoid past mistakes -
Open data-driven decision making
-
Think of openness more broadly
-
Govern data
-
Not as technical objects
-
Reconceptualize them as open social and technical processes
Your preview ends here
Eager to read complete document? Join bartleby learn and gain access to the full version
- Access to all documents
- Unlimited textbook solutions
- 24/7 expert homework help
-
Citizen engagement
-
Technological citizenship
-
Better govern open systems
-
Important to avoid technological colonialism
-
Normalized within smart systems, digital twins, and not addressed in digital strategies
1.
Open Data Social and Technological Assemblage
-
Open data thought of as an assemblage
-
“Implies that context frames how such data are socially understood in their environment, while technologies, processes, and materialities are the content that perform the tasks of making data open”.
-
Open data differs from place to place/
-
As an assemblage they are consistent and known.
-
Actors and their subjectivities also matter, as they bring different approaches, priorities, skills, and knowledge
-
Open data are different for a scientist, an app developer, a chief data officer, a company, a government administrator, and an environmentalist. -
looking at open data as a discursive regime, and examining how its constitutive elements have evolved in different places across time
-
becomes possible to imagine what this social and technological assemblage might look like in a smart context.
-
This framing may lead to greater systems thinking
2.
The Legacy of Technical Conceptualizations of Open Data
-
Critical data scholars
-
“accept that the usual technological conceptualization of data as unbiased, objective, and neutral scientific facts about the world is limited and narrow”
-
Open data assessed against these criteria
-
Global Open Data Index (GODI)- lacks critical assessment of data
-
Critical assessment of data
-
Quality -
lineage or provenance
-
positional accuracy
-
attribute accuracy,
-
Completeness
-
logical consistency
-
semantic accuracy
-
temporal information
-
Gaps
-
Equity and inclusion
-
Disability and accessibility
-
Canada
Your preview ends here
Eager to read complete document? Join bartleby learn and gain access to the full version
- Access to all documents
- Unlimited textbook solutions
- 24/7 expert homework help
-
“Even though Canada ranked high according to GODI, a
lack of data quality or a spatial referent means that important social and political analytical work, such as comparing the outcomes of national health programs or educational achievement”
-
these are not the data types listed as being important according to GODI’s criteria
-
Pandemic
-
GODI- the list of essential datasets a nation state ought to publish also proved to be lacking
-
while data were published, there remained a distinct absence of foundational open data on retirement homes, residences for people with disabilities, and disaggregated equity and health data in general
-
Impact
-
“Technical conceptualizations of open data have
resulted in many open datasets being available in open data portals”
-
“we cannot create much evidence-
informed policy with them because of a lack of quality, interoperability, and data gaps”
-
Open data indices generally assess data on a national scale
-
Canada is a federation
-
Data less likely to be interoperable or standardized
-
GODI does not assess inter-jurisdictional
cooperation or data from a systems perspective
-
Mechanisms beyond standardization to support coordinated efforts
-
Focus in geomatics and not on framework data related to health regions or socio-economi data
-
Spacial data infrastructures
-
Indigenous data considerations
-
Absent from technical conceptualizations
-
“cosmologies, ontologies, and epistemologies about what constitute Indigenous data, data models, and open data differ from conceptions found in Western notions of empiricism and science”
-
First Nations Information Governance Centre FNIGC
-
Sovereignty over data in the possession of the Crown
-
Considered to belong to FN in a post-colonial and reconciliation
Your preview ends here
Eager to read complete document? Join bartleby learn and gain access to the full version
- Access to all documents
- Unlimited textbook solutions
- 24/7 expert homework help
context
-
Actors involved with open data in public administrations are different from actors in science, and both differ from Indigenous conceptions
-
OCAP- principles of ownership, control, access and possession
-
Open Data Charter 2015- more nuanced than GODI
-
Six principles that state that data are to be
-
(1) open by default
-
Come under scrutiny
-
Indigenous data
-
Labour-intensive process for govt -
Shift- publishing with a purpose
-
Open Data for Results- mitigate data gaps and invisabilities -
(2) timely and comprehensive
-
(3) accessible and usable
-
(4) comparable and interoperable
-
Lag time in adoption of the Open Data Charter- issues with this
-
(5) for improved governance and citizen engagement
-
Data governance
-
(6) for inclusive development and innovation
-
Data for governance
-
Experiences for International Organizations
-
Value chains
-
Values: fairness, equity, inclusion,
and the identification of data gaps
-
Police-shooting data
-
Data on missing and murdered aboriginal women
-
No stats programs about disabled people
-
Open Data Barometer
-
“Barometer applies the technical criteria of the Open Definition, the technical and policy principles of the Open Data Charter, and the G20 Anti-Corruption Open Data Principles -
to assess open data readiness in terms of the ability to deliver open data, how that delivery is done, and the political, social, and economic impacts of open
Your preview ends here
Eager to read complete document? Join bartleby learn and gain access to the full version
- Access to all documents
- Unlimited textbook solutions
- 24/7 expert homework help
data—and it does so quantitatively and qualitatively”
-
“considering open data more broadly, even though the legacy of the technological criteria for
open data persist, as does the lack of attention to data quality”
-
City of Ottawaa
-
Data-sharing cultures inside government
-
Make data accessible and interoperable within organizations
-
Cross-institutional teams
-
“legacy administrative data systems in different business units preclude their ability to share data internally, and this is changing how new technologies are procured”
-
City of Ottawa’s Community and Social Services Department
-
“collects data in seven different information-
management systems, some of which belong to other levels of government, and is now trying to reconcile how it will standardize the collection of equity and inclusion data across these to better inform service delivery, most notably the ongoing public-health response to the COVID-19 pandemic”
-
Technical and data interoperability have been identified as a new priority in how data are produced, as has the need for semantic interoperability if processes are to be automated, such as in the case of artificial intelligence and machine learning (AI/ML).
-
Accessibility
-
requirements in North America that digital government systems be accessible, which is also the case for open data and their visualization
Your preview ends here
Eager to read complete document? Join bartleby learn and gain access to the full version
- Access to all documents
- Unlimited textbook solutions
- 24/7 expert homework help
-
this form of accessibility is not assessed by GODI, the Open Data Charter, or the Open Data Barometer, as data about accessibility are not considered to be a key dataset to be published by any of these indicator systems nor is the notion of the accessibility of data for disabled people.
3.
Global Data Sharing -
Genealogical approach
-
“access to data conceptually differs from open data, as it is more about data sharing between a set of specific actors, but it is from this social and technological practice of sharing data that an environment conducive for the emergence of open data exists”
-
“Subjecticities of the data-producing cultures”
-
The early narrative on access to scientific and spatial data was grounded in systems and infrastructure thinking
-
data are part of technical, nstitutional, organizational, collaborative, research, and results-based systems, -
data-sharing practices were purposedriven (e.g.,
climate modelling).
-
multi-billion-dollar Earth observation (EO) community
-
EO principles
-
sharing data for sustainable development, resource management, evidence-based decision-making in those
areas, a“benefit of humankind,” and economic viability
-
Open data- discursive regime of sharing publicly funded data in
the absence of restrictions
-
“Open data thinking also coincides with the development of techniques and technologies related to the spatial web and the launch of Google Maps, the advent of OpenStreetMap, and crowdsourcing, and to Web 2.0 platforms such as Facebook and Wikipedia, as
well as mobile devices such as smartphones”
-
The social web and mobile devices enabled people not only to be consumers of data but also to be content producers
-
creating a new set of data actors who were not necessarily scientists, data authorities, or producers in government but people skilled with coding, open source, APIs, and data science.
-
Data sharing
-
Domesday Book of 1086
-
Invention of the printing press
-
Late Enlightenment Period- Statistical, social-science, and scientific associations -
Antartic Treaty of 1959 principles
Your preview ends here
Eager to read complete document? Join bartleby learn and gain access to the full version
- Access to all documents
- Unlimited textbook solutions
- 24/7 expert homework help
-
UN Earth Summit 1992- making data accessible
-
Indigenous Peoples
-
Local knowledge- translating it into digital data for sustainable development
-
Access to data
-
Capacity building
-
Open science -
Restructuring of government institutions involved in science and statistics -
Buildng open data and data-sharing infrustractures
-
GEOSS
-
Spatial data infrastructures
-
Open Data as an International Concept (2005-2006)
-
Normalized Practices
-
becoming routinized and operationalized in governments
-
it is disjointed as the focus is primarily on administrative data and, to a lesser extent, survey and science data
-
Although evolving “open data is not a systems-based approach, it is a policy, as it was in the sciences, where there was a purpose to sharing data within a community
of practice or an epistemic community”
-
public-sector administrators who create data for the purpose of managing and operating government programs. New open data institutions are forming
-
situated within a datamanagement and an information-technology (IT) context
4.
Open Data in Canada
-
1979-Canada Institute for Scientific and Technical Information
-
created to ensure that scientists had access to the data and information they need to do their work
-
1988- Canadian Association of Research Libraries
-
created a research data consortium
-
1988- Canadian Association of Public Data Users
-
Discourse of access to data
-
featured in government reports, most notably the mid-1980s Ministerial Task Force on Program Review, commonly known as the Nielsen Task Force
-
Led by Deputy Prime Minister Erik Nielsen 1984
-
Brian Mulroney
-
Sociologist Paul Bernard
-
“knowledge is fundamental to economic development and democratic life in advanced societies; and the information gathered by statistical agencies is an important component of that knowledge. It is essential that such information be made available to researchers and to the public so that it can be used in debates and decision-making.”
-
Federal Dagta Liberation Initative
-
Operationalizing open data
Your preview ends here
Eager to read complete document? Join bartleby learn and gain access to the full version
- Access to all documents
- Unlimited textbook solutions
- 24/7 expert homework help
-
Nanaimo, British Columbia (2009)
5.
Open Data in Smart Systems
-
Open Data programs “subsumed as part of smart cities initiative”
-
Becoming a component of smart cities
-
Not necessarily open data and tech systems- not being developed according to practices of open science or spacial data infrustractures
-
2017 Infrastructure Canada- Smart Cities Challenge- the call
-
meaningful smart city outcomes included making data, decisionmaking, and technological processes open, transparent, and interoperable
-
mandated that chosen technologies be transferable, and preferably open source and standards-based, for reuse by other communities; that communities have ownership over their data; and that technologies empower and enable communities large and small, as well as traditional and non-traditional partners, to collaborate and strengthen relationships between residents and public organizations, including gender-
based analysis
-
The procurement of technology and the ownership of data became part of the strategy, and cities had to define challenges to address with technology and also had to consult with their residents to do so
-
Sidewalk Labs- Toronto Quayside project
-
No data-governance strategy- data not going to be oen
-
Open-smart city aspirations are becoming a reality; the definition counters the enclosure of data and technology, and it is becoming a made-in-Canada model
-
the lack of systems and infrastructural thinking overall in
data and technology spaces; be they large social and technological systems such as smart cities, digital twins,
or smart grids for utilities, we do not yet see data-
governance plans that are systems-based, integrated, interoperable, standardized, and open.
-
Will open data and open smart cities be included in digital strategies and become a core principle of data-
governance plans?
-
open smart cities, “where residents, civil society, academics, and the private sector collaborate with public officials to mobilize data and technologies when warranted in an ethical, accountable and transparent way to govern the city as a fair, viable and livable commons and balance economic development, social progress and environmental responsibility”
6.
Conclusion
-
“Canada has a unique open data social and technological assemblage and genealogy”
-
technical conceptualizations of open data persist, which limit the scope
Your preview ends here
Eager to read complete document? Join bartleby learn and gain access to the full version
- Access to all documents
- Unlimited textbook solutions
- 24/7 expert homework help
of open data.
-
argued that open data will need to be governed as part of large social and technological systems; that its discourse ought to be about democratic deliberation and not just a new managerialist exercise; and that we move beyond narrow technological conceptions and include ethics, public good, and sustainability.
-
Future:
-
“The future of open data, as it normalizes, will be subsumed as part of large and smart-technological systems, and we need to ensure that data and the systems that generate them remain open”. -
“Open data actors will need to work with open smart city and digitaltwin actors to ensure that data remain open, and city actors need to think of smart cities and digital twins as more than operations, and to consider them to be part of urban plans and as part of public space that ought to be deliberated.”
-
“good governance includes, among other things, data and technological governance,and an inherent part of doing technological citizenship (Barney, 2004), since we do live in technological societies
LECTURE NOTES
WEEK 7: SPATIAL DATA AND YOU -
October 18
SUMMARY
Meier, P. 2015. The Rise of Digital Humanitarianism in Digital Humanitarians: How Big Data is Changing the Face of Humanitarian Response, CRC Press.
LINK
NOTES
Your preview ends here
Eager to read complete document? Join bartleby learn and gain access to the full version
- Access to all documents
- Unlimited textbook solutions
- 24/7 expert homework help
LECTURE NOTES
WEEK 8: DATA WALK
-
November 1
SUMMARY
Powell, A. 2018, The Data Walkshop and Radical Bottom-up Data Knowledge; Chapter 9 in Ethnography for a Data-Saturated World by editors H. Knox and D. Nafus, Manchester University Press.
LINK
NOTES
ABSTRACT
-
Produce big data from the bottom up
-
he kinds of civic assemblages that we identified as examples of bottom-up big data don’t operate in the same way as those from the top down.
-
Assembelges part of the production and positioning of big data are large scale
-
Computer power and the institutional meaning-making apparatus required to create frameworks and application spaces for this data are all easier to mobilise top down.
-
individual agency and reflexivity as well as the variable ways in which power and participation are constructed and enacted
-
Examines
-
strategies for examining public matters of concern in relation to data production
-
following from and developing from previous efforts at surfacing and valorising situated knowledge in particular
urban contexts
-
identifying how ‘bottom-up’ data subjectivity could become collaborative and collective through the use of participatory
Your preview ends here
Eager to read complete document? Join bartleby learn and gain access to the full version
- Access to all documents
- Unlimited textbook solutions
- 24/7 expert homework help
meaning-making processe
-
Approach
-
who is asking the questions about big data
-
lets us think about how data gets to be ‘big’ in the first place, who asks the questions that make it big (in size as well as importance)
-
how one might ask different kinds of questions
-
Chapter focus
-
genesis and development of the Data Walking project as a means of asking different questions about ‘big’ data, space and local knowledge
The Data Walk
-
Definition of Data Walk
-
The "data walk" or "data walkshop" is a grassroots approach to exploring and defining data, particularly big data and data politics.
-
It involves citizens forming groups to walk, observe, discuss, and document connections between data, datafication processes,
and the places they live.
-
Process
-
Begins with a large group discussion to establish an understanding of data.
-
Participants are assigned specific observational roles based on their interests.
-
Small groups take walks in local areas, focusing on identifying "data calm" and "data rich" spaces and "data activations" where data intersects with other aspects of life.
-
The participants are encouraged to find places of data resistance.
-
At the end of the walk, each group documents their journey, observations, and collects physical objects, creating a narrative of their experience.
-
Applications
-
Used as a tool for civic engagement and in broader reflections on social or economic processes.
-
Aims to create a phenomenology of data and connects with ethnographic explorations of space, movement, and context in knowledge production.
-
Interdisciplinary Approach
Your preview ends here
Eager to read complete document? Join bartleby learn and gain access to the full version
- Access to all documents
- Unlimited textbook solutions
- 24/7 expert homework help
-
Highlights the contribution of artistic practice to social science research.
-
Emphasizes the need for interdisciplinary collaboration to address data-related phenomena.
-
Beyond Data Subjectivity
-
Challenges the dominant focus on "data subjectivity" as the primary way datafication is experienced.
-
Aims to surface everyday experiences and reflections on data by
involving people in ethnographic practices.
-
Alterative Modes of Sense-Making
-
Suggests that challenging top-down data assemblages doesn't always require parallel bottom-up ones but rather alternative modes of making sense of data.
-
Emphasizes the creative and expressive knowledge production in response to data challenges.
-
Community Engagement and Conceptual Tool
-
Data walk serves as a form of community and civic engagement.
-
It functions as a conceptual tool for generating alternative epistemologies and ontologies for big data and datafication.
-
Emphasizes the importance of countering narrow, instrumental, or coercive data use with creative and expressive knowledge production.
Genesis of the data walkshop
-
Philosophical and Urban Plannning Background
-
Walking reflections have been used by philosophers, psychogeographers, urban planners, and community organizations.
-
These walks aim to explore relationships between people, ideas,
knowledge, and space, and sometimes to identify local assets
-
Educational Origin
-
The author's version of the data walk started as a teaching tool for students.
-
It aimed to provide students with a physical, spatial, and sensory
understanding of the ethnographic experience of data proliferation.
-
The concept of situated knowledge, as proposed by Haraway (1988), was central to this exercise.
-
Counterpoint to Big Data Celebrations
Your preview ends here
Eager to read complete document? Join bartleby learn and gain access to the full version
- Access to all documents
- Unlimited textbook solutions
- 24/7 expert homework help
-
The data walk was designed as a conceptual counterpoint to the enthusiastic discussions around big data.
-
Students engaged with both proponent views of big data and critical viewpoints to gain a holistic perspective.
-
Diverse applications
-
The data walk concept was applied in various contexts, including collaborations with artists, urban planners, data ethics PhD students, social activists, and researchers.
-
These walks facilitated the exchange of different ideas and helped people from diverse backgrounds understand and define data.
-
Collaborative Knowledge Production
-
Over time, the data walk evolved into an event that enabled new
forms of collaborative knowledge production.
-
It became a platform for sharing expertise and generating insights collectively.
-
Inspiration from Flashmob Ethnography and Network Walkshops:
-
The data walk was inspired by the "flashmob ethnography" framework, which aimed to make ethnographic practice more participatory.
-
The walk also incorporated elements from Adam Greenfield's network walkshops, which focused on digital networks in city spaces.
-
Structured Roles and Goals:
-
Participants were assigned specific roles within small groups, such as photography, map-making, thick description, and interviewing.
-
The goal was to observe the role of values in urban infrastructure and public spaces, encouraging the documentation
of tensions and counter-intuitive findings.
-
Focus on Spaces of Mediation:
-
The walk emphasized spaces where information is collected by networks, where networked information is displayed, and where
networked information influences choices and actions.
-
where networked information influences people's actions.
-
Critical Making Workshops
-
The data walk was followed by workshops involving "critical making" to analyze observed relationships and envision alternative interpretations or critical futures.
-
Critical making as a pedagogical tool aimed to inspire active
Your preview ends here
Eager to read complete document? Join bartleby learn and gain access to the full version
- Access to all documents
- Unlimited textbook solutions
- 24/7 expert homework help
citizenship and encourage everyday political and social critique.
-
Influence from Cultural Life of Cities:
-
The concept of walking and observing was influenced by de Certeau's attention to everyday life, Benjamin's celebration of walking, and psychogeographic traditions.
-
Walking and observing were seen as practices that contribute to the cultural life of cities, and these practices were linked to technological mediations and the idea of the "smart city."
-
New Understandings of Data:
-
The data walk aimed to bring new spaces, phenomena, and knowledge into existence through attentive walking and observing.
-
It was seen as a way to foster new understandings of data in the context of urban environments and everyday life.
Conceptual antecedents: rethinking the smart city and the objective god-eye -
Inspiration from Haraway's Situated Knowledge:
-
The observational approach in the text was influenced by Donna
Haraway's concept of "situated knowledge."
-
Haraway emphasized that scientific observation is influenced by
a particular perspective, challenging the notion of an objective "god-eye" view of the world.
-
Constructive Orientation:
-
The data walk had a constructive focus, encouraging students to use ethnographic data collected during the walks for future interventions in the observed city spaces.
-
It aimed to provoke reflections on the possibility of influencing how cities are mediated and experienced.
-
Interest in Smart Cities and Technological Mediation:
-
The author had a longstanding interest in the role of technology in mediating city experiences, particularly in the context of "smart cities."
-
Considered how technology and data played a role in reshaping urban environments and experiences.
-
Focus on Procedure and Data Understanding:
-
The concept of "procedure" reframed the understanding of data in relation to prediction and potential.
-
Explored how algorithmic processes were linked to information systems committed to computational knowledge generation.
-
Challenges in Expanding Data Understanding:
Your preview ends here
Eager to read complete document? Join bartleby learn and gain access to the full version
- Access to all documents
- Unlimited textbook solutions
- 24/7 expert homework help
-
Despite exploring citizen science, open data, and civic movements, the author found it challenging to step outside the technological framework and see data in a broader context.
-
Critical Scholarship on the Smart City:
-
Current critical scholarship on the "smart city" often assumes that specific types of data are generated through large-scale civic projects, leading to the ideal data city.
-
This vision is criticized for its generic and totalizing nature.
-
Transformation of Citizenship in the Data City:
-
In the datafied "smart city," citizenship can become more about citizens as sensors, collecting and presenting computational information.
-
This view of citizenship shifts from traditional democratic engagement to a form of "biopolitics 2.0" or "soft biopolitics."
-
Possibilities of Citizen Data Production:
-
Some scholars explore the potential of citizen data production, such as collaborative mapping, to slow down the intensification of calculation and display different sensitivities.
-
This aligns with a broader project of cosmopoliticization, where
new potentials are made public without seeking praise or criticism.
-
DIY Approaches and Experimental Data Citizenship:
-
Scholars and movements like DIY technical and cultural subcultures experiment with computational worlds and urban spaces.
-
These approaches consider how people can question and intervene in the idea that city life has become datafied or computational.
-
Data Walk as a Discussion and DIY Practice Tool:
-
The data walk emerged as a way to stimulate discussions and DIY practices beyond those already engaged in these concepts and movements.
Interdisciplinary encounters
-
Collaboration with Artists and Geographers:
-
The author's ideas about walking, data, and open engagement with data and cities were significantly enriched through interdisciplinary collaborations with artists and geographers.
-
The collaboration was initiated with artist and geographer Paula
Your preview ends here
Eager to read complete document? Join bartleby learn and gain access to the full version
- Access to all documents
- Unlimited textbook solutions
- 24/7 expert homework help
Crutchlow for the "Museum of Contemporary Commodities" (MoCC) project.
-
MoCC Project's Research-Creation-Engagement Approach:
-
MoCC aimed to examine trade (in)justices as collective future heritage, integrating research, creation, and engagement.
-
The project sought to combine critical art practice with the pedagogical focus on trade (in)justices and cultural activism.
-
Transformation of the Data Walk Perspective:
-
The interdisciplinary collaboration transformed the perspective and process of the data walk.
-
Paula Crutchlow's background as a performance artist and her focus on movement and disruptive creative acts added a new dimension to the data walk.
-
Reframing the Walk's Purpose:
-
Paula's perspective highlighted the potential for the data walk to
provide a phenomenological experience and generate alternative
knowledge about the city.
-
It was seen as a means to destabilize social hierarchy and create opportunities for collective experience.
-
Datafication of Urban Space and Poetic Tactics:
-
Paula raised concerns about the impact of pervasive datafication
on urban space, suggesting that walking as a poetic tactic might contribute to administrative strategies of consumption and security.
-
She questioned the desirability and feasibility of developing subjectivities resistant to datafication processes.
-
The Value of Structured Small Groups:
-
Working with structured small groups helped create shared definitions of "data," "information," and "knowledge" in a non-
hierarchical way.
-
This approach acknowledged the existence of partial perspectives and different ways of constructing knowledge.
-
Decentring Research Expertise:
-
The collaboration emphasized a decentring of research expertise
by involving participants in a structured experience similar to a performance.
-
Playfulness and shifts in subjectivities characterized the walkshops, where participants observed the world and experimented with the act of observation
-
Shift in Situated Knowledge and Reflective Experience:
Your preview ends here
Eager to read complete document? Join bartleby learn and gain access to the full version
- Access to all documents
- Unlimited textbook solutions
- 24/7 expert homework help
-
Rather than assuming that ethnographic observation collects objective truths, this approach highlighted the construction of situated knowledge through experience.
-
Participants were encouraged to be reflective about how their experience influenced their understanding.
-
Narrative Reporting of the Walks:
-
After the walk, embodied and imaginary practices were used to perform a re-journeying of the route.
-
Participants, as experts in their own experiences, were collectively responsible for representing the group's findings.
-
Interrogating the Significance of Data:
-
The interdisciplinary encounter prompted a careful consideration of the significance of data, highlighting its dependence on mediation and interpretation.
-
It raised fundamental questions about what data constitutes, how
it can be contested, and how experiences of datafication can be challenged and reimagined through shared experiences.
Data walk process: data walkers make trouble -
Focus on Phenomenal Experienc
-
Building on Paula's insights, the author began to emphasize the phenomenal experience of the data walk.
-
This perspective is rooted in social science and art practice, particularly works by Lee and Ingold (2006) and Cantarella et al.
-
Introduction of New Ideas about Expertise and Data Politics:
-
The author saw the data walk as a process of experience and inquiry, introducing new ideas about expertise and data politics.
-
Data walks were opened up to various groups in different contexts to create complementary learning experiences.
-
Diverse Participants and Destabilizing Expertise
-
Data walks involved various groups, including urban planners, community advocates, PhD students, and residents from different neighborhoods and cities.
-
The experience of destabilizing expertise and learning while walking in small groups created new pathways for knowledge sharing and individual reflection on expertise.
-
Exchange of Expertise
-
Participants appreciated the opportunity to meet and understand how different people thought about common issues.
Your preview ends here
Eager to read complete document? Join bartleby learn and gain access to the full version
- Access to all documents
- Unlimited textbook solutions
- 24/7 expert homework help
-
In a small group with defined responsibilities and no clear leader, all knowledge was revealed as situated.
-
Subjectivity and Data Interpretation:
-
Participants developed their subjectivity through critical maneuvers for interpreting data.
-
The experience challenged the notion that subjectivization through data is the sole way people engage with data.
-
Moving from Individual to Collective:
-
The performative method used in data walks helped shift the focus from individual experiences of data to collective engagement.
-
Participants highlighted the collective nature of the experience as a valuable aspect.
-
Creation of DataWalking.org Website:
-
As the project matured, the author established the DataWalking.org website to explain the data walk process and foster exchanges with others experimenting with similar approaches.
Situating and reflecting on surveillance -
Urban Mediation and Data Produced by Objects:
-
Some work in geography and communication studies assumes that urban mediation primarily involves "data produced by objects," such as sensors and cameras.
-
This data becomes integrated into organizational, calculative, and decision-making processes, shaping the experience of urban
space.
-
Construction of Subjectivity through Observation and Narrative:
-
While a narrow view might suggest that data produced by objects constructs subjectivity, data walks reveal that it is the performance of observation and narrative reconstruction that produces subjectivity.
-
This process can be transformative, generating new forms of collective and collaborative knowledge.
-
Critique of Data-Based Surveillance:
-
Participants in data walks often critiqued and observed traces of data-based surveillance.
-
They photographed surveillance architecture, including surveillance cameras, passcode-protected gates, and RFID readers at railway stations.
Your preview ends here
Eager to read complete document? Join bartleby learn and gain access to the full version
- Access to all documents
- Unlimited textbook solutions
- 24/7 expert homework help
-
Inscrutability of Surveillance Installations:
-
Many of these surveillance installations are inscrutable on their own; it's challenging to determine their functionality and data usage, let alone who it serves.
-
The frustration with this "calculative frame" led data walking groups to explore alternative data assemblages.
-
Violation of Social Expectations:
-
In some instances, data walking groups violated social expectations to draw attention to inscrutable installations.
-
An example from Copenhagen involved a group photographing a metal pole near a public library, which was part of a perimeter
security project.
-
Revealing a New Understanding of Geographies and Politics:
-
During a lengthy interview with a security guard, it was revealed that the perimeter system was installed to prevent graffiti on the library building.
-
This raised questions about the "graffiti zone" concept and highlighted how attention to data and its ambiguities can lead to new relations and understandings of geographies and politics.
Investigating data assemblages -
Focus on 'The Digital' in the City:
-
In various data walks, participants concentrated on identifying signs of 'the digital' within the city.
-
They observed web addresses, telephone numbers, and indicators of networked information systems overlaid onto physical spaces.
-
Exploration of Web Addresses in Physical Spaces:
-
Participants photographed and described instances where web addresses were displayed on buildings, raising questions about the connection between the physical and online worlds.
-
For example, a non-functional web address was inscribed on a community gardening project's wooden hut in Copenhagen, prompting walkers to consider the interplay between online information and tangible data surrounding the shed.
-
Data Geographies and Layers:
-
This experience resonates with previous research on data geographies, highlighting the layers and complexities of data in physical spaces.
-
The relationship between the digital and the physical is a subject
Your preview ends here
Eager to read complete document? Join bartleby learn and gain access to the full version
- Access to all documents
- Unlimited textbook solutions
- 24/7 expert homework help
of exploration (references to Kitchin, Graham, Marvin, Crang, and Graham).
-
Datafication in Everyday Life:
-
Data walks revealed that the apparatus of data subjectivation is not the sole or most significant aspect of datafication in daily life.
-
Critical data scholars often emphasize how data operations, like classification, organization, processing, and visualization, shape
the value and power of data.
-
Challenging Classification Processes:
-
Initially, data walks were thought to intervene in classification processes by allowing citizens to observe, audit, or resist surveillance and data classification.
-
However, the diverse interpretations of 'what is data?' by participants exceeded expectations and exploded preconceived notions.
-
Moving Beyond Technological Focus:
-
Data walkers developed nuanced approaches that moved beyond
focusing on technologically mediated data.
-
They delved into the conceptual challenge of identifying and observing various types of data, even attempting to 'see the invisible.'
-
Data and Information Collapse:
-
In Montreal, participants reflected on the blurring of lines between data and information, examining the consequences of permanent tracking systems for shared transportation versus temporary appearances of 'non-datafied' versions.
-
They explored the significance of different data types in understanding place and potential inequalities, such as contrasting 'non-datafied' bus stops with sensor-enabled car-
sharing systems.
-
Data Defined by Community:
-
Participants redefined data as both quantitative and qualitative elements that hold importance within a specific community.
-
The Montreal walkshops contributed to this definition, emphasizing the context-dependent nature of data.
Data for someone else – decentred perspectives on data -
Fascination with Uninterpreted Data:
-
Participants in data walks often found themselves intrigued by
Your preview ends here
Eager to read complete document? Join bartleby learn and gain access to the full version
- Access to all documents
- Unlimited textbook solutions
- 24/7 expert homework help
data or information within the urban environment that they knew was significant to a system but could not interpret.
-
Reading Markings Under Train Tracks:
-
In Finsbury Park, one group of walkers was captivated by markings under train tracks, interpreting combinations of letters and numbers as important data meant for another audience.
-
Data Beyond "Data Produced by Objects":
-
While "data produced by objects" is a common focus for critiques, datafication in urban life involves various other processes.
-
Processes related to classification and knowledge production are
equally relevant.
-
Reflecting on What Deserves Attention:
-
Walkshops encouraged participants to reflect and reimagine what aspects of the urban environment are worth attending to.
-
Some groups used technological tools to expand their observation of the "invisible."
-
Data Glitchy Photography in Finsbury Park:
-
In Finsbury Park, an artist-participant repurposed a domestic scanner to produce data-glitchy photographs, distorting the city's visual perception as if it were data.
-
This intervention highlighted the faulty production of data in this assemblage.
-
Algorithmic Intervention in Copenhagen:
-
In Copenhagen, a group programmed a random walk generator as an algorithmic intervention to influence their walk's direction.
-
A significant portion of their walk time was dedicated to negotiating with and eventually reprogramming the algorithm.
-
WiFi Signal Mapping in Montreal:
-
In Montreal, one group used their mobile phones to display the number of WiFi signals at different points along a busy street.
-
They marked the locations with the most WiFi spots on maps they created during the walk.
-
Changing the Perception of the City:
-
The act of attending to the city as a site of data transforms its essence.
-
Historical monuments become just one element that can emerge from a space characterized by invisibilities and power relations.
-
Unintentional Ignorance of Historical Significance:
Your preview ends here
Eager to read complete document? Join bartleby learn and gain access to the full version
- Access to all documents
- Unlimited textbook solutions
- 24/7 expert homework help
-
In London, a group of walkers expressed melancholic reflection on their inability to truly comprehend the historical significance of the locations they passed in the city.
-
Without deliberate attention, they inadvertently overlooked numerous historically important sites.
-
Data walk outcomes -
Processes of counter-subjectivication -
Creating Different Data Subjectivities:
-
Data walking has the potential to generate diverse experiences of data subjectivity that involve new definitions, contentions, and resistant positions.
-
These collective subjectivities are constructed through relationships between participants as they collaboratively seek to define and derive meaning from data.
-
Moving Beyond Helpless Passivity:
-
Data walk outcomes challenge the notion that data subjects must passively submit to the use of their data by
corporations or engage in entrepreneurial subject positions.
-
They provide a space for people to respond to the demand "not to be reduced to data" and to interact with data in ways that defy state-determined limitations.
-
Change in Data-Related Behavior:
-
Participants often considered how the practice of data walking, involving walking, thinking, and paying attention, changed their city and heightened their awareness of potential places for intervention.
-
Instead of limiting data production or altering their behavior to avoid generating certain data, they explored how their engagement with data transformed their surroundings.
-
Filtering New Perspectives into View:
-
By performing expertise within a data walk, individuals can make previously unnoticed aspects of the environment "become data" and connect with other people and concerns.
-
Data walking can be seen as a strategy for becoming a
Your preview ends here
Eager to read complete document? Join bartleby learn and gain access to the full version
- Access to all documents
- Unlimited textbook solutions
- 24/7 expert homework help
data citizen.
-
Themes Across Different Groups:
-
Participants commonly shared interests in exploring the liminal aspects of urban life and the interplay between these elements and data systems.
-
Navigating the barrier and balance of datafication, they made the invisible visible and documented everyday excesses of datafication.
-
Some saw a political aspect to this approach, pushing against full datafication that might lead to a completely organized city with no room for spontaneity or unpredictability.
-
Role of Structure and Movement:
-
The structure of data walks, with distinct roles for participants and the requirement to create a map, narrows the focus of data collected and forces a performative narrative.
-
This interplay between structure and movement breaks down the distinction between data as "what is given" and
data as something that must always be actively constructed through observation, expertise, filtering, contestation, and narrative, often in conversation with others.
-
Challenging Big Data Paradigms:
-
Data walks, with their collaborative and performative structure, provide a method for generating diverse types of data.
-
They also challenge the ways in which top-down big data paradigms limit the possibilities for experiencing and researching data.
-
Paying attention and staying with the trouble -
Paying Attention and Staying with the Trouble:
-
The data walk process emphasizes the act of paying attention and attending to the liminal aspects of urban life, suggesting ways to undertake what Isabelle Stengers and Donna Haraway call 'paying attention' and 'staying with the trouble.'
-
Challenging Reductionist Thinking:
-
Stengers and Haraway argue that current ways of
Your preview ends here
Eager to read complete document? Join bartleby learn and gain access to the full version
- Access to all documents
- Unlimited textbook solutions
- 24/7 expert homework help
thinking about science and data block individuals from understanding their relationships with the worlds they inhabit.
-
These feminist philosophers challenge reductive thinking by pushing for alternative perspectives that consider the complex interactions between humans, nature, and technology.
-
Critique of Anthropocene and Capitalocene:
-
Haraway critiques the concept of the anthropocene, which attributes ecological transformation solely to human activity, and suggests that the term Capitalocene might be more descriptive.
-
She highlights the importance of acknowledging changes in scale, rate, synchronicity, and complexity that have shaped world systems.
-
Cthulucene as a New World-Thinking Mode:
-
Haraway introduces the idea of the Cthulucene, a world-
thinking mode that entangles various temporalities, spatialities, and intra-active entities, including human and non-human elements.
-
This perspective allows for more comprehensive and robust biological-cultural-political-technological recuperation and recomposition.
-
Reconsidering 'God-Eye' and Data Rendering:
-
Philosophically, the intense focus of data walks challenges the notion of a 'god-eye' perspective, suggesting that only certain types of actions can be rendered as data.
-
The stories of liminality and inscrutability in urban data speak to the problematic nature of mediation and the idea that "the being of humankind is to be outside itself,"
as proposed by Bernard Stiegler.
-
Engagement with Alterity and Ethics:
-
Kember and Zylinska call for an ethics that acknowledges productive engagement with alterity, emphasizing the importance of recognizing the Other and engaging with difference.
-
Redefining Data in Radical Media Studies:
-
As media transition into becoming data, radical media studies might benefit from reimagining and redefining
Your preview ends here
Eager to read complete document? Join bartleby learn and gain access to the full version
- Access to all documents
- Unlimited textbook solutions
- 24/7 expert homework help
data, acknowledging its complexities and the need to consider new perspectives and ethical frameworks.
-
The call to explore these threads and develop them further reflects the evolving nature of datafication in everyday communication and society.
Conclusion
-
Objective Data Perception:
-
Data is often perceived as objective, and its subjectivity is typically focused on the individual rather than the collective.
-
Challenges to Constructed or Phenomenological Views:
-
When data is viewed as constructed or phenomenological, there is a challenge in integrating people's everyday, emotional, and non-expert knowledge into this construct.
-
Evolution of Data Walks:
-
Data walks have evolved over time, integrating various philosophical and political positions related to walking traditions and their relevance to data.
-
Framework for Collective Knowledge Creation:
-
The data walk has developed into a framework for radical, collective, bottom-up knowledge creation and sharing, emphasizing performative practices.
-
Alignment with Diverse Interests:
-
The data walk approach has proven adaptable and relevant to a wide range of participants, fostering collaborations and friendships while accommodating various concerns and perspectives related to data, information, knowledge, and place.
-
Countering Ontological and Epistemological Frameworks:
-
Data walks offer an alternative to emerging ontological frameworks that present data as an inscrutable force larger than individuals.
-
They also challenge epistemological frameworks that claim data
as an absolute truth.
-
Repositioning Knowledge and Feeling:
-
Through their humanity, data walks reposition knowledge and emotions, highlighting the potential for data to be created through performative acts.
-
Promoting Joy, Curiosity, and Engagement:
-
The primary goal of data walks is not to entirely counter the datafied experience of everyday life but to provoke joy,
Your preview ends here
Eager to read complete document? Join bartleby learn and gain access to the full version
- Access to all documents
- Unlimited textbook solutions
- 24/7 expert homework help
curiosity, and engagement.
-
They offer new ways to tell stories and foster fresh perspectives on why data matters.
LECTURE NOTES
WEEK 9: DATA BROKERS AND YOU
-
November 8
SUMMARY
-
Data brokers buy, privatize, and resell information.
-
Form alliances to gatekeep information behind absurd paywalls (Cartels)
-
Antitrust laws are made to prevent these alliances, don’t affect Data Cartels as the public are largely unaware of their operations.
-
Consumers become the creators and buyers of their own data - data that should be open
to the public.
-
Misinformation becomes publicly available, while the truth is locked behind a paywall
-
2 Types of Data Brokering: Commercial: Sold to smaller organizations / individuals
(Individual items such as publications, ect.)
Institutional: Sold to large organizations (typically Big data collected from civilians)
Bigger Data Brokers typically do institutional brokering, setting high prices intended for large companies or governments to buy. This locks out individuals or smaller, scholarly organizations from having access.
-
Datasets created by government held under lawful standards for ethical data collection, but when datasets are bought from brokers the source of the data is not questioned. Thus governments can use unethically sourced data created by brokers.
Lamdan, Sarah. 2022. In Data Cartels: The Companies That Control and
Your preview ends here
Eager to read complete document? Join bartleby learn and gain access to the full version
- Access to all documents
- Unlimited textbook solutions
- 24/7 expert homework help
Monopolize Our Information, 1–26. Stanford University Press. https://doi.org/10.1515/9781503633728-003.
Chapter 1: The Data Cartels: An Overview
LINK
(Requires authentication through Ares) https://www.degruyter.com/document/doi/10.1515/9781503633728-003/html
NOTES
All about Data Dealers:
-
Informations selling companies (RELX/Thomson Reuters) privatize any information they can obtain the rights to, and then resell the info.
-
Paywall exclusive information, can set their prices for the information due to their exclusive access to the information
-
Use their high prices on info to buy smaller companies that produce the information, further privatizing data.
-
Data brokers conduct data analytics on some private data sets, sell the analytics.
-
Often take data created by consumers (Through devices and services offered to us), and resell that data. Consumers are both the supply and the demand.
-
This closing of data occurs in many fields (Academic publishings, journalism, ect).
What makes these companies Data Cartels?
-
“Data Cartels”: Maintaining high prices by restricting competition (in this case by creating a monopoly on information).
-
There are (anti-trust) laws to prevent collusion of large companies creating cartels.
These laws often ignore Data Cartels, as they operate in the background
and rarely make news.
Lamdan, Sarah. 2022. In Data Cartels: The Companies That Control and Monopolize Our Information, 1–26. Stanford University Press. https://doi.org/10.1515/9781503633728-003
.
Your preview ends here
Eager to read complete document? Join bartleby learn and gain access to the full version
- Access to all documents
- Unlimited textbook solutions
- 24/7 expert homework help
Chapter 2: Data Brokering
LINK
(Requires authentication through Ares) https://www.degruyter.com/document/doi/10.1515/9781503633728-003/html
NOTES
-
2 Types of Data Brokering: Commercial: Sold to smaller organizations / individuals
(Individual items such as publications, ect.)
Institutional: Sold to large organizations (typically Big data collected from civilians)
Bigger Data Brokers typically do institutional brokering, setting high prices intended for large companies or governments to buy. This locks out individuals or smaller, scholarly organizations from having access.
-
Datasets created by government held under lawful standards for ethical data collection, but when datasets are bought from brokers the source of
the data is not questioned. Thus governments can use unethically sourced data created by brokers.
LECTURE NOTES
Data Brokers:
●
Collect consumer data
○
Offline & Online
○
Purchasing or otherwise obtained
●
Typically data collected without the knowledge of the consumer
●
Data are combined and analyzed by brokers
●
Collect and/or sell raw or analyzed data about people for various purposes
○
Sold to those who might use that data to influence their decisions.
Ex: Social Credit, Insurance, advertizing ect.
●
Records of old data maintained alongside updated current data.
US Law definition:
Your preview ends here
Eager to read complete document? Join bartleby learn and gain access to the full version
- Access to all documents
- Unlimited textbook solutions
- 24/7 expert homework help
“Business that knowingly collects and sells to third parties the personal information of a consumer with whom the business does not have a direct relationship.”
Within “Data Cartels”:
2 Types of Data brokers:
●
Commercial Data Brokering - companies crunch data and sell services
●
Institutional Data Brokering - companies sell data to the government and other institutions. (REXL & Thomson Reuters, mentioned in the readings, are ‘Institutional’ brokers.
First party
- Amazon, Google (Obtain and use large quantities of user data)
Second Party
- Smaller companies, typically collect & use data for their own uses.
Third Party
- Very big, private & public records. Since they’re records, the data is
not necessarily used by 3rd party.
“Cartels”
●
“Alliances of Rivals”: actors which collude in order to dominate and control the market.
●
Anti-Trust regulations were created to prevent this control over the market, but have been lax.
●
*In reading: REXL & Thomson Reuters are continuously buying and merging small publishing / data analysis companies in order to control the availability of data on the market.
This is so they may control the price at which they sell these data.
Metaphors/Concepts in the reading:
●
Translators of Moral Choice
Your preview ends here
Eager to read complete document? Join bartleby learn and gain access to the full version
- Access to all documents
- Unlimited textbook solutions
- 24/7 expert homework help
(Data decides whether someone is considered “risky”, ex. insurance)
●
Reduce our rights to algorithms (Ensuring the methods in which this data is collected are hidden away, so that one can’t easily influence the outcome)
●
Datafication of injustice
(Those who buy the data may use it for any nefarious purposes, without punishment to the seller)
●
Shopping malls for information:
(Self explanatory)
●
Mosaic of Immigrant Lives (Immigrants have large amt of data available, might be used by ICE, ect.)
●
360 degree view
(Illusory objective nature of data)
●
Hoarding information and wall it off
(Taking open-source scholarly data, buying the rights for cheap, and walling it off until it is sold for much more later, resulting in closed-source expensive data)
●
Paywalled / Walled Gardens (See above)
●
Benevolent shopping dictators
(COVID databases were made open by RELX & Thomson Reuters during peak covid, but that data is at the liberty of the data brokers to provide, rather than being open source for everyone.)
●
Octopus-like monopolies
(Wide network branching into every part of the market)
●
Sow digital harms
●
Operate in the shadows (Their data is used in many important systems (insurance/credit score), but the brokers themselves stay out of the public eye)
●
“The truth is paywalled and the lies are free” - Motivation for attempting to open this data to the public.
What do data brokers know?
Your preview ends here
Eager to read complete document? Join bartleby learn and gain access to the full version
- Access to all documents
- Unlimited textbook solutions
- 24/7 expert homework help
Data brokers are able to collect many kinds of data on a person tied to their digital devices.
Ex. Locations, movement patterns, age, how active (if using physical monitoring
apps), ect.
Metaphor: Panopticon
The panopticon: Pan meaning all, and optic meaning view
The panopticon is a prison in which a watch tower maintains a 360 degree view of a circular construction of the cells around the tower.
Thus, the tower has the potential to be watching every cell at once.
Metaphor for data brokers: One can never be certain that they’re not being
watched (or that their data is not being collected)
Panoptic Sorting:
Predictive analytics based on the data collection of an individual.
Ex. Insurance, how likely is someone to crash based on their demographic?
This can take into account age, sex, race, ect. so long as the algorithm is hidden.
Data processing:
-
Surveillance
“The systematic investigation or montiroing of the actions or communications of one or more persons.”
-
Personal dataveillance
“Identifiable persons who by their actions have attracted the attention of the panoptic system”
-
Mass surveillance
“... is the surveillance of groups of people (usually large groups) and the reason for
Your preview ends here
Eager to read complete document? Join bartleby learn and gain access to the full version
- Access to all documents
- Unlimited textbook solutions
- 24/7 expert homework help
investigation or monitoring is to identify individuals who belong to …”
-
Dataveillance:
“... is the systematic use of the personal … (cut off)
Processes
●
Indentification ●
Classification
●
Prediction
●
Targeting
WEEK 10: WATCHING YOU
SUMMARY
-
Survellance is not always bad, must examine the context/purpose of the surveillance as well as where the data collected is going.
-
Many different types & methods of surveillance, gone over in the lecture notes.
-
Who is collecting the data / organizing the surveillance:
Is this source trustworthy?
-
What is the purpose of the surveillance?
Data collection
Security
-
What is being done with the data collected?
Selling of spacial data and otherwise
Collected for security purposes
Analysis for profit optimization of corporations
Law enforcement
-
Misidentification: What happens when a person is misidentified by these surveillance systems? What are the consequences?
-
Surveillance infrastructure:
Widespread existence of data infrastructure exists in many places, how do we ensure that this infrastructure is being used ethnically?
-
Rights and freedoms:
How might surveillance infringe on personal freedoms?
Your preview ends here
Eager to read complete document? Join bartleby learn and gain access to the full version
- Access to all documents
- Unlimited textbook solutions
- 24/7 expert homework help
Monahan, T. and Murakami Wood, D., 2018, Surveillance Studies a Reader,
Oxford University Press.
CHAPTER 1
LINK
Ch. 1, J. B. Rule, Private Lives and Public Surveillance: Social Control in the Computer Age
NOTES
Monahan, T. and Murakami Wood, D., 2018, Surveillance Studies a Reader,
Oxford University Press.
CHAPTER 4
LINK
Ch. 4, D. Lyons, Surveillance Studies: An Overview
SUMMARY
REFLECTION
Monahan, T. and Murakami Wood, D., 2018, Surveillance Studies a Reader,
Oxford University Press.
CHAPTER 9
LINK
Ch. 9, K. D. Haggerty and R. V. Ericson, The Surveillant Assemblage
SUMMARY
REFLECTION
Your preview ends here
Eager to read complete document? Join bartleby learn and gain access to the full version
- Access to all documents
- Unlimited textbook solutions
- 24/7 expert homework help
Monahan, T. and Murakami Wood, D., 2018, Surveillance Studies a Reader,
Oxford University Press.
CHAPTER 22
LINK
Ch. 22, D. Nelkin and L. Andrews, DNA Identification and Surveillance Creep
KEY
SECTIONS
REFLECTION
Monahan, T. and Murakami Wood, D., 2018, Surveillance Studies a Reader,
Oxford University Press.
CHAPTER 46
LINK
Ch. 46, R. Clarke, Information Technology and Dataveillance
KEY
SECTIONS
REFLECTION
Monahan, T. and Murakami Wood, D., 2018, Surveillance Studies a Reader,
Oxford University Press.
CHAPTER 72
LINK
Ch. 72, S. Browne, Dark Matters: On the surveillance of Blackness
KEY
SECTIONS
REFLECTION
LECTURE NOTES
Surveillance is not necessarily nefarious,
Your preview ends here
Eager to read complete document? Join bartleby learn and gain access to the full version
- Access to all documents
- Unlimited textbook solutions
- 24/7 expert homework help
Consider surveillance with the intention of catching “bad actors”
(Criminals, assassins, ect).
|As well as health surveillance, monitoring health statistics to predictively catch medical emergencies before they occur (Heart-rate monitoring, catching cancer early, ect.)
Surveillant Assemblage
Who is collecting the data (surveilling), and for what purpose?
●
Data flows / Data doubles
●
“Multiplicity of heterogeneous objects, whose unity comes solely from the fact that
these items function together, that they work together as a functional entity”
●
“Surveillance is driven by the desire to bring systems together, to comine practices and technologies and integrate them into a larger whole”
●
The body is abstracted into data, then reassembled into different settings through a series of data flows - data double
●
Data about the body is broken into components for the purpose of observation
●
Centres of calculation (labs, forensic centres, ect.), data doubles circulate within these
●
Data trail: What is left behind by passive or active activities
●
Data detritus: (Data decay? how it changes over time. Ex. Martial status, age, ect)
Dataveillance:
Mass Surveillance:
●
Mass surveillance infrastructure is fixed, with marginal cost
(It’s already in place, and only has maintenance costs)
Lends itself to automation, automatically surveilling areas (recording, ect)
without the assurance that the data will ever be used / looked at.
NDA ID & Surveillance Creep:
●
Surveillance creep: How data, originally collected for a specific purpose, might have it’s purpose change over time.
Data that was collected for a good purpose might be used later for nefarious
Your preview ends here
Eager to read complete document? Join bartleby learn and gain access to the full version
- Access to all documents
- Unlimited textbook solutions
- 24/7 expert homework help
purposes.
Data Surveillance:
Eavesdropping gets you the conversation:
Surveillance gets you everything else (video? all other aspects)
Data is content, metadata is context.
Surveillance gets you the context
Dataveillance is cheaper:
●
Surveillance is expensive
●
Corperate surveillance is growing and cheaper - data brokers
●
Enablers:
○
Computational Power
○
Mass distributed (digital) storage
○
Data analysis techniques (optimization)
○
Cheaper devices
●
Cheaper = wider distributed
Misidentification:
-
The implications of face-identification systems being used and misidentifying a person are varied and disturbing. (Identifying criminals, facial tracking in video games, ect. ect.)
-
Facial identification systems are far from perfect, especially for non-white people, as the machine learning systems were originally developed using a largely white majority.
-
The implications of “gaydar”-like systems that detect groups of people can be incredibly dangerous in countries with anti-lgbt laws.
Surveillance Studies:
-
“Focused, systematic and routine attention to personal details for purposes of
Your preview ends here
Eager to read complete document? Join bartleby learn and gain access to the full version
- Access to all documents
- Unlimited textbook solutions
- 24/7 expert homework help
influence, management, protection or direction”
(Above: Watching over people for <positive intentions>)
-
Studying how to implement positive surveillance systems within society and otherwise.
-
Asymmetric distribution of power
(Those in the room get to decide how/why people are surveilled, potentially deciding who gets surveilled more often (potentially arbitrarily or nefariously)
Hidden Surveillance:
-
Hidden and normalized infrastructure is powerful, as it is easier for the public to ignore and/or passively accept as typical.
Sousveillance
●
“Watching from below: Watching the watchers”
●
Ex. Recording police using mobile phones
●
“Question the asymmetrical nature of surveillance” & Keeping “the watchers” accountable.
●
Reflectionism increases equality between the surveyor and the surveille
●
(Ex: If a story is told using recordings from one of two parties, it may be edited in favor of that party / discarded if it is not in favor.
By recording both parties, we can create an equal power distribution (or get closer to it).
Neo-Panopticon (dataveillance):
●
Privacy is a psychological, social, and political requirement.
●
Unknowing subjects / objects of surveillance
●
Inducing abeyance (state of disuse)
Coveillance:
●
Watching each other
●
Should be done within the public interest, keeping each other accountable.
Your preview ends here
Eager to read complete document? Join bartleby learn and gain access to the full version
- Access to all documents
- Unlimited textbook solutions
- 24/7 expert homework help
Counterveillance:
●
Intentionally deceiving / avoiding surveillance systems as a new form of balance (Wearing masks, sweaters, glasses, hiding faces, ect.)
●
This type of surveillance avoidance actually results in being watched more, as one becomes an outlier in the system.
●
Typically only effective on a large scale so that one is not an outlier for doing so (Hong Kong protests)
●
Otherwise, appearing typical is a form of ‘avoiding surveillance’ - blending in to avoid unique treatment.
WEEK 11: AL/ML ALGORITHMS, AUTOMATION, ROBOTS AND YOU
SUMMARY
Crawford, Kate and Joler, Vladan. 2018. Anatomy of an AI System: The Amazon Echo As An Anatomical Map of Human Labor, Data and Planetary
Resources. AI Now Institute and Share Lab. http://www.anatomyof.ai.
LINK
NOTES
LECTURE NOTES
Planetary Scale & Materialism of Smart Devices
-
Smart devices rely on unsustainable resource extraction practices in their
Your preview ends here
Eager to read complete document? Join bartleby learn and gain access to the full version
- Access to all documents
- Unlimited textbook solutions
- 24/7 expert homework help
manufacturing. One example of this is the use of Lithium, used in the batteries of smart devices, which
is often sourced from unethical mining practices in underdeveloped countries.
These devices have a limited lifespan, and must be replaced.
This becomes a greater problem due to profit-maximizing business practices that aim to have consumers replace an entire product rather than having it repaired.
(Right to repair laws being developed to combat these practices)
-
Labor:
Unethical working environments within underdeveloped countries is often mandatory to maintain the supply chain required to source ‘semi-global’ accessibility to these devices.
-
Energy consumption:
Consists of the energy required to create the product, as well as to operate it.
AI & Machine Learning:
-
Machine learning: Large data sets are used to inform the correct action for the machine to take in any given situation. These data sets are constantly evolving as a response to consumer data collection. Thus AI devices are required to constantly listen and examine any input given to them.
-
Bias:
When the data set is largely formed using data from a non-diverse group, it only learns
to respond to that group. If the data set grows to a large volume within a non-diverse group, it will be difficult to reverse these effects, as newer groups will be unaccepted by the norms that the system is accustomed to.
-
Machine Objectivity:
When relying on the accuracy of a machine learning device to make decisions, many believe that the system is unable to make a mistake. When machines are assumed to be correct, often safeguards are not put in place to check for inaccuracies. Thus, when
these machines are given tasks that have potential consequences to citizens, biases within the dataset can lead to inaccuracies that manifest real consequences.
-
Observation:
When accepting that smart devices are constantly listening to input, maintaining privacy relies on the practices of the provider of that smart device. While there is an expectation that privacy laws should keep our data safe, many use these devices
Your preview ends here
Eager to read complete document? Join bartleby learn and gain access to the full version
- Access to all documents
- Unlimited textbook solutions
- 24/7 expert homework help
without assurance that their conversations and data might remain private.
-
Algorithm Design:
“Black box”: Inputs and outputs to the system are seen, but how the system actually works to processes the inputs is unseen.
Consumers see the algorithms in their black-box form; They see how a smart device will respond to the commands given to them, but how those responses are formed are unknown. Without open algorithms, we do not know the extent to which the algorithms may be designed to influence or manipulate the user.
For example, when asking a smart device (Google Home / Amazon Alexa) about fast food nearby, we may get a list of potential options. We are unsure as to whether these options are obtained by proximity to the user, if there may be a preference for franchises owned by the producer, or any other potential biases to the information given.
Furthermore, we do not know how the system might process this request as part of learning; are we considered vulnerable to food-delivery advertisements as a result of this question?
WEEK 12: DATA ACTIVISM, DATA JUSTICE & YOU
-
November 29
SUMMARY
D’Ignazio, C. and Klein, L. F. 2020, Data Feminism, The MIT Press.
Introduction: Why Data Science Needs Feminism
Conclusion: Now Let’s Multiply
Our Values and Our Metrics for Holding Ourselves Accountable
LINK
KEY
SECTIONS
Introduction: Why Data Science Needs Feminism
-
Christine Mann Darden at NASA (1967):
-
Newly minted master's degree in applied math.
-
Became a data analyst at NASA's Langley Research Center.
Your preview ends here
Eager to read complete document? Join bartleby learn and gain access to the full version
- Access to all documents
- Unlimited textbook solutions
- 24/7 expert homework help
-
Context of high tensions in the U.S., including protests against the Vietnam War and the 1967 Detroit Riot.
-
Space Race and Darden's Contribution:
-
Space race backdrop; the U.S. losing to the Soviet Union.
-
Darden's data analysis crucial for the successful return of Apollo 11, securing a U.S. victory.
-
Darden, a Black woman, faced sexism and racism at NASA, challenging the nation's ideals.
-
Women Computers at NASA:
-
Overview of Langley's Advanced Computing Division and the use of human computers.
-
Discrimination faced by women computers, often treated as unskilled temporary workers.
-
Recent recognition of their contributions, thanks to works like "Hidden Figures."
-
Feminism and Data Science
-
Linking feminism to the belief in the political, social, and economic equality of the sexes.
-
Advocacy work integral to feminism and its application to data science.
-
Darden’s Advocacy and Career Path
-
Darden's observations at Langley: men with math credentials in engineering, women in computing pools.
-
Darden's courageous approach to her division chief to address gender disparities.
-
Relevance of Darden's experience to the book's focus on feminism and data science.
-
Intersectionality and Critique of Early Feminism
-
Discussion of Betty Friedan's "The Feminine Mystique" and its limitations.
-
Introduction of the concept of intersectionality, considering
race, class, and other dimensions.
-
Recognition of the diverse experiences of women and the need for integrated analysis.
-
Drawing from Black Scholars and Activists
-
Reference to bell hooks and the critique of Friedan's neglect of non-white and poor women.
-
Emphasis on intersectionality as crucial for addressing present challenges.
-
Acknowledgment of the authors' positionality as straight, white, cisgender women.
-
Central aim of the book
-
Description of the book's aim: an intersectional feminism addressing present inequities using data.
-
Acknowledgment of the complexity of the task and the need for ongoing efforts.
Your preview ends here
Eager to read complete document? Join bartleby learn and gain access to the full version
- Access to all documents
- Unlimited textbook solutions
- 24/7 expert homework help
-
Encouragement for readers to take steps toward justice, drawing inspiration from Darden's story.
-
Christine Darden's self-advocacy as a model for challenging power and working toward justice.
-
The potential for using data to remake the world in a more
equitable way.
What is Data Feminism?
-
Christine Darden’s Career Advancement
-
Darden's groundbreaking research and accomplishments at NASA.
-
Despite her achievements, she faced unequal recognition compared to male counterparts.
-
Consultation with Gloria Champine and the revelation of gender-based promotion disparities.
-
Feminism and Darden’s Success
-
Acknowledgment of feminist movements bringing women's issues to the forefront.
-
Darden's rise attributed to both individual efforts and collective feminist activism.
-
Recognition of the systemic nature of gender inequality through data visualization.
-
Diversity within Feminism
-
Definition of feminism encompassing diverse projects challenging sexism and oppression.
-
Reference to the term "feminisms" acknowledging the varied nature of feminist activism.
-
Inclusion of regular individuals, public intellectuals, organizing groups, scholars, and activists.
-
Intersectionality and its importance
-
Introduction to Kimberlé Crenshaw's concept of intersectionality in the late 1980s.
-
Explanation of the DeGraffenreid v. General Motors case illustrating the need for intersectional analysis.
-
Emphasis on the intersection of various dimensions of identity and forces of privilege and oppression.
-
Power Differentials and Data Feminism
-
Recognition of unequal power distribution globally.
-
Data feminism's aim to address existing inequalities in data science.
-
Commitment to co-liberation and the idea that oppressive systems harm everyone.
-
Authors’ Background and Motivation
-
Introductions of the authors, Catherine and Lauren.
-
Catherine's background as a hacker mama, experiences in tech, and motivation to reframe the tech world with a feminist lens.
Your preview ends here
Eager to read complete document? Join bartleby learn and gain access to the full version
- Access to all documents
- Unlimited textbook solutions
- 24/7 expert homework help
-
Lauren's description as a professional nerd, her work in early American literature, and the aim to connect historical issues of racism and sexism to contemporary data science challenges.
-
Data and its Role in Power Dynamics
-
Historical origin of the term "data" in the mid-seventeenth century.
-
Rhetorical purpose of identifying information as data for creating a solid basis for claims.
-
Questioning whose information becomes data and the need for trust in data feminism.
-
Christine Darden’s Story and the Power of Data
-
Darden's use of data to make arguments and effect change.
-
The collaboration between Darden and Champine highlighting flaws in data-driven projects.
-
The recognition that data feminism underscores the importance of people behind the numbers.
-
People Behind the Data
-
Emphasis on the collaborative nature of data work.
-
The inherent reduction of life experience when converting it into data.
-
Data feminism as both a goal and a process, guiding thoughts and actions toward remaking the world.
Data Feminism in Action
-
Data as a Double-edged Sword
-
Data has been historically used by those in power as a tool to consolidate control.
-
The book aims to reveal how governments and corporations employed data to maintain an unequal status
quo.
-
Expansive Definition of Data Science:
-
Definition of data science through three examples, highlighting its diverse applications.
-
Examples include AI-driven art, systems addressing human reporting bias in speech, and gender analysis in Hollywood film dialogue.
-
Seven Core Principles of Data Feminism
-
Overview of the seven principles derived from intersectional feminist thought.
-
Principles include examining and challenging power, elevating emotion and embodiment, rethinking binaries and hierarchies, embracing pluralism, considering context,
and making labor visible.
-
1. Examine power. Data feminism begins by analyzing how power operates in theworld.
Your preview ends here
Eager to read complete document? Join bartleby learn and gain access to the full version
- Access to all documents
- Unlimited textbook solutions
- 24/7 expert homework help
-
2. Challenge power. Data feminism commits to challenging unequal power structuresand working toward justice.
-
3.Elevate emotion and embodiment. Data feminism
teaches us to value multiple forms of knowledge, including the knowledge that comes from people as
living, feeling bodies in the world.
-
4. Rethink binaries and hierarchies. Data feminism requires us to challenge the gender binary, along with other systems of counting and classification that perpetuate oppression.
-
5. Embrace pluralism. Data feminism insists that the most complete knowledge comes from synthesizing multiple perspectives, with priority given to local, Indigenous, and experiential ways of knowing.
-
6. Consider context. Data feminism asserts that data are not neutral or objective. They are the products of unequal social relations, and this context is essential for conducting accurate, ethical analysis.
-
7. Make labor visible. The work of data science, like
all work in the world, is the work of many hands. Data feminism makes this labor visible so that it can be recognized and valued
-
Introduction of feminist Concepts
-
Introduction of feminist concepts such as the matrix of domination, situated knowledge, and emotional labor.
-
Connection of these concepts to the principles of data feminism.
-
Focus on People at the Cutting Edge
-
Highlighting individuals at the forefront of data and justice,
including engineers, activists, artists, and scholars.
-
Presentation of diverse projects challenging power through content, form, and process.
-
Defining Feminism in Data Projects
-
Exploration of what makes a data project feminist in terms
of content, form, and process.
-
Emphasis on a commitment to action and a desire to remake the world.
-
Goals Against the Status Quo
-
The overarching go al is to stand against a world that benefits certain individuals at the expense of others.
-
Prioritization of voices from marginalized communities and
those with direct experience of inequality.
-
Addressing Inequalities at the intersection
Your preview ends here
Eager to read complete document? Join bartleby learn and gain access to the full version
- Access to all documents
- Unlimited textbook solutions
- 24/7 expert homework help
-
Acknowledgment of inequalities at the intersection of gender and race.
-
Recognition of racism as a source of entrenched issues of
inequality, particularly in the United States.
-
Inclusivity and Openings for Conversatiojn
-
Acknowledgment of choices made in selecting voices and work to include.
-
Inviting readers to view gaps as openings for conversation
and contribute to the ongoing dialogue.
-
Audience and Purpose of the Book
-
Intended for data scientists, feminists, professionals in data-driven fields, and communities engaging with data.
-
Aims to provide concrete steps for action and enhance understanding of charts and statistics in day-to-day life
-
Data feminism for Everyone
-
Affirmation that data feminism is inclusive of all genders.
-
Emphasis on its relevance beyond gender, focusing on power differentials and challenging and changing them using data.
CONCLUSION
Google Walkout for Real Change
-
November 1, 2018, a global walkout involving over 20,000 Google workers.
-
Triggered by revelations of a $90 million exit package for Andy Rubin, accused of sexual misconduct.
-
Seven core organizers crafted demands, including ending forced arbitration and promoting diversity.
Historical Precedents and Collective Power:
-
Historian Mar Hicks connects the Google walkout to a 1970s UK strike by computer workers.
-
Explores the power of collective organizing and disruption of essential services.
-
Highlights the bargaining power of core workers in tech companies.
Tech Workers' Collective Actions:
-
Discusses the potential impact of tech workers using their collective power.
-
Examples include Slack developers' potential strike and efforts by groups like Tech Workers Coalition.
-
Explores how digital solidarity can translate into physical spaces and human relationships.
Design Justice and Data for Black Lives
-
Design Justice Network focuses on redesigning design to empower marginalized communities.
-
Data for Black Lives, founded by Yeshimabeit Milner, aims for
Your preview ends here
Eager to read complete document? Join bartleby learn and gain access to the full version
- Access to all documents
- Unlimited textbook solutions
- 24/7 expert homework help
meaningful change using data and technology.
-
Both emphasize values, principles, and community engagement.
Diverse Forms of Collective Organizing
-
Explores various forms of collective organizing beyond white-
collar workers.
-
Examples include Tech Solidarity, Lerna JavaScript library's political stand, and worker-owned tech cooperatives.
-
Mention of codes of ethics, the Toronto Declaration, and values guiding open government initiatives.
Challenges and Unfulfilled Demands
-
Highlights challenges faced post-walkout, including legal actions,
retaliation, and insufficient responses.
-
Google's partial compliance with demands, emphasizing the need for an intersectional feminist approach.
-
Ongoing struggles for equity, transparency, and inclusive culture.
Matrix of domination
-
Google's legal actions as an example of the matrix of domination.
-
Points out the need to monitor powerful groups and collaborate with those most impacted.
-
Acknowledges the importance of featuring diverse voices and experiences throughout the book.
-
Definition
-
Framework developed by Patricia Hill Collins
-
Analyzes how various social structures (race, class, gender, sexuality) intersect and interact.
-
Forms a complex web of power relations.
-
Oppression and Privilege: -
Shapes individuals' experiences of both oppression
and privilege.
-
Comprehensive Analysis: -
Encourages a holistic understanding of social inequality.
-
Multidimensional Impact: -
Recognizes that each axis of oppression influences
lives in multiple dimensions.
-
Context in the Text: -
Used to explain how powerful institutions maintain control and resist challenges by deploying structural and disciplinary domains.
-
Monitoring Power: -
Emphasizes the need to monitor dominant groups and institutions.
-
Collaboration
-
stresses collaboration with groups most impacted by power differentials.
Your preview ends here
Eager to read complete document? Join bartleby learn and gain access to the full version
- Access to all documents
- Unlimited textbook solutions
- 24/7 expert homework help
-
Intersectional Feminism
-
Supports addressing issues from an intersectional feminist
Expansive Conception of Data Science
-
Emphasizes the need for a broad view of data science beyond quantitative methods.
-
Challenges the myth of solo data science work and advocates for
collaboration.
-
Discusses interventions required at each phase of data work for feminist data science to thrive.
Diverse Starting Points for Change
-
Highlights various starting points for challenging oppression, including academia, activism, and the arts.
-
Examples range from Indigenous statistics and design justice to AI critiques and political activism.
-
Emphasizes the importance of building links between diverse ecosystems.
Call to Multiply and Mobilize
-
Encourages the multiplication of efforts, ecosystems, and collaborations.
-
Urges resistance and reimagination before the full determination of norms in the data economy.
-
Invites collective action and courage to envision a data science beyond the matrix of domination.
OUR VALUES AND OUR METRICS FOR HOLDING OURSELVES ACCOUNTABLE
Multivocal Feminism:
-
Historical feminist movements lacked equal value for diverse voices.
-
Suffrage movement excluded Black women; lesbian feminists faced exclusion.
-
Activists, especially Black feminists, emphasize intersectionality
—considering social power related to gender, race, class, ability, sexuality, and more.
Equity as Outcome and Process:
-
Equity addresses an unjust past, valuing diverse knowledges.
-
Process involves listening deeper, challenging the status quo, and prioritizing marginalized voices.
-
Patricia Hill Collins emphasizes the need to account for historical
injustices.
Prioritizing Proximity:
-
Kimberly Seals Allers highlights the importance of community knowledge.
Your preview ends here
Eager to read complete document? Join bartleby learn and gain access to the full version
- Access to all documents
- Unlimited textbook solutions
- 24/7 expert homework help
-
Prioritize voices with direct experiences of injustice over distant perspectives.
-
Communities intimately understand problems, uncounted phenomena, and effective interventions.
Acknowledging the Humanity of Data:
-
Transformation of human experience into data often simplifies and reduces complexity.
-
Data has been wielded as an instrument of oppression, reinforcing inequality.
-
Effort to introduce context, acknowledge complexity, and recognize data's limits.
Reflexive, Transparent, and Accountable:
-
Acknowledge that knowledge is shaped by perspectives and limitations.
-
Strive to be reflexive, transparent, and accountable for the work.
-
Recognize the journey toward justice involves making mistakes and learning from them.
-
Open to direct and critical feedback, viewing it as a generous offer and a chance for transformation.
Evolution of Metrics:
-
Evolving table of explicit metrics to guide citation audits and examples in the book.
-
Recognition of foregrounding race and racism due to the U.S. context.
-
Commitment to transparency, reflexivity, and accountability in addressing issues of inequality and injustice.
A Reflection on Our Final Metrics
-
complexities of maintaining values, recognizing biases in scholarly practices, and the ongoing need for accountability.
Shifts in Metrics:
-
Notable changes between the first draft and the final version of metrics.
-
Achieved the goal of including a trans voice in every chapter.
-
More Indigenous perspectives in the final version than in the first draft
Unmet Aspirational Metrics:
-
Proportional representation of women, nonbinary people, and people of color decreased.
-
Proportionally fewer projects from the Global South.
-
More projects from the academic realm.
-
Less work from individuals directly impacted by the issues.
Explanations for Outcomes:
-
Process-related explanation: Did not keep precise tabs on citational metrics during revision.
-
Aspirational metrics avoided to prevent tokenization and gaming
Your preview ends here
Eager to read complete document? Join bartleby learn and gain access to the full version
- Access to all documents
- Unlimited textbook solutions
- 24/7 expert homework help
the system.
-
Gaps between draft metrics and aspirational metrics considered during revision.
-
Unaware of changing proportions of projects and citations, leading to an imbalance.
Root Cause of the Issue:
-
Root cause attributed to the authors and their positions as scholars in higher education.
-
Acknowledgment of the dominance of white individuals, especially white men, in the academic world.
Citations and Scholarly Bias:
-
Peer review process emphasized backing assertions with citations.
-
Additional citations added between draft and final version to enhance scholarship.
-
Unintentional reproduction of biases of academia in citational practices.
-
Reflection on the similarity of this mechanism to the privilege hazard discussed in the book.
Quantified Reminder of Bias:
-
Final metrics serve as a quantified reminder that "legitimate knowledge" carries race, gender, class, and geographic location characteristics.
-
Acknowledgment of falling back on learned habits in citational practices.
-
Despite challenging biases in writing, the authors recognize their adherence to scholarly credibility pressures.
Values and Accountability
-
Acknowledgment that values alone are not enough; they need consistent action and accountability.
-
Emphasis on the difficulty and time-consuming nature of maintaining accountability.
-
Uncomfortable final metrics serve as evidence of the gap between ideals and actions, guiding efforts to bridge those gaps and persist.
REFLECTION
LECTURE NOTES
Your preview ends here
Eager to read complete document? Join bartleby learn and gain access to the full version
- Access to all documents
- Unlimited textbook solutions
- 24/7 expert homework help
WEEK 13: CRITICAL DATA STUDIES AND YOU
-
December 6
SUMMARY
Kitchin, R. and Lauriault, T. P. 2018, Toward Critical Data Studies: Charting and Unpacking Data Assemblages and Their Work, Chapter 1 in Thinking Big Data in Geography: New Regimes, New Research, edited by J. Thatcher, J. Ekert and A. Shears.
LINK
NOTES
LECTURE NOTES
Your preview ends here
Eager to read complete document? Join bartleby learn and gain access to the full version
- Access to all documents
- Unlimited textbook solutions
- 24/7 expert homework help
Related Documents
Recommended textbooks for you
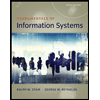
Fundamentals of Information Systems
Computer Science
ISBN:9781337097536
Author:Ralph Stair, George Reynolds
Publisher:Cengage Learning
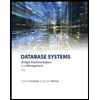
Database Systems: Design, Implementation, & Manag...
Computer Science
ISBN:9781305627482
Author:Carlos Coronel, Steven Morris
Publisher:Cengage Learning
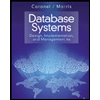
Database Systems: Design, Implementation, & Manag...
Computer Science
ISBN:9781285196145
Author:Steven, Steven Morris, Carlos Coronel, Carlos, Coronel, Carlos; Morris, Carlos Coronel and Steven Morris, Carlos Coronel; Steven Morris, Steven Morris; Carlos Coronel
Publisher:Cengage Learning
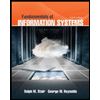
Fundamentals of Information Systems
Computer Science
ISBN:9781305082168
Author:Ralph Stair, George Reynolds
Publisher:Cengage Learning
Np Ms Office 365/Excel 2016 I Ntermed
Computer Science
ISBN:9781337508841
Author:Carey
Publisher:Cengage
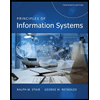
Principles of Information Systems (MindTap Course...
Computer Science
ISBN:9781305971776
Author:Ralph Stair, George Reynolds
Publisher:Cengage Learning
Recommended textbooks for you
- Fundamentals of Information SystemsComputer ScienceISBN:9781337097536Author:Ralph Stair, George ReynoldsPublisher:Cengage LearningDatabase Systems: Design, Implementation, & Manag...Computer ScienceISBN:9781305627482Author:Carlos Coronel, Steven MorrisPublisher:Cengage LearningDatabase Systems: Design, Implementation, & Manag...Computer ScienceISBN:9781285196145Author:Steven, Steven Morris, Carlos Coronel, Carlos, Coronel, Carlos; Morris, Carlos Coronel and Steven Morris, Carlos Coronel; Steven Morris, Steven Morris; Carlos CoronelPublisher:Cengage Learning
- Fundamentals of Information SystemsComputer ScienceISBN:9781305082168Author:Ralph Stair, George ReynoldsPublisher:Cengage LearningNp Ms Office 365/Excel 2016 I NtermedComputer ScienceISBN:9781337508841Author:CareyPublisher:CengagePrinciples of Information Systems (MindTap Course...Computer ScienceISBN:9781305971776Author:Ralph Stair, George ReynoldsPublisher:Cengage Learning
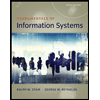
Fundamentals of Information Systems
Computer Science
ISBN:9781337097536
Author:Ralph Stair, George Reynolds
Publisher:Cengage Learning
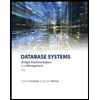
Database Systems: Design, Implementation, & Manag...
Computer Science
ISBN:9781305627482
Author:Carlos Coronel, Steven Morris
Publisher:Cengage Learning
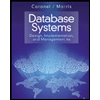
Database Systems: Design, Implementation, & Manag...
Computer Science
ISBN:9781285196145
Author:Steven, Steven Morris, Carlos Coronel, Carlos, Coronel, Carlos; Morris, Carlos Coronel and Steven Morris, Carlos Coronel; Steven Morris, Steven Morris; Carlos Coronel
Publisher:Cengage Learning
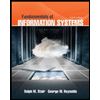
Fundamentals of Information Systems
Computer Science
ISBN:9781305082168
Author:Ralph Stair, George Reynolds
Publisher:Cengage Learning
Np Ms Office 365/Excel 2016 I Ntermed
Computer Science
ISBN:9781337508841
Author:Carey
Publisher:Cengage
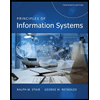
Principles of Information Systems (MindTap Course...
Computer Science
ISBN:9781305971776
Author:Ralph Stair, George Reynolds
Publisher:Cengage Learning