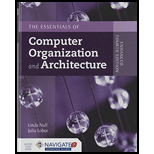
Essentials of Computer Organization and Architecture
4th Edition
ISBN: 9781284074482
Author: Linda Null, Julia Lobur
Publisher: Jones & Bartlett Learning
expand_more
expand_more
format_list_bulleted
Concept explainers
Expert Solution & Answer
Chapter 7, Problem 44E
Explanation of Solution
Magnetic Disk:
- The magnetic disk is a storage device that uses a process called magnetization in order to perform read and write operation.
- The disk is covered with magnetic coating and it saves information in the form of tracks and sectors.
- Example: Floppy disk
Pros: Disks are faster than tapes. It is reliable that is, it does not need human to change tapes or remote backup.
Cons: Disk backup is more expensive.
Magnetic Tape:
- Magnetic tapes are made up of thin, magnetizable coating.
- Magnetic tapes support various track densities and it undergoes serpentine recording method or helical scan recording method.
- A tape with higher density is more economical and allows to take quick backup...
Expert Solution & Answer

Want to see the full answer?
Check out a sample textbook solution
Students have asked these similar questions
In completing this report, you may be required to rely heavily on principles relevant, for example, the Work System, Managerial and Functional Levels, Information and International Systems, and Security. apply Problem Solving Techniques (Think Outside The Box) when completing. should reflect relevance, clarity, and organisation based on research. Your research must be demonstrated by Details from the scenario to support your analysis, Theories from your readings, Three or more scholarly references are required from books, UWIlinc, etc, in-text or narrated citations of at least four (4) references.
“Implementation of an Integrated Inventory Management System at Green Fields Manufacturing” Green Fields Manufacturing is a mid-sized company specialising in eco-friendly home and garden products. In recent years, growing demand has exposed the limitations of their fragmented processes and outdated systems. Different departments manage production schedules, raw material requirements, and…
1. Create a Book record that implements the Comparable interface, comparing the
Book objects by year
- title: String
>
- author: String
- year: int
Book
+ compareTo(other Book: Book): int
+ toString(): String
Submit your source code on Canvas (Copy
your code to text box or upload.java file)
>
Comparable
2. Create a main method in Book record.
1) In the main method, create an array of 2 objects of Book with your choice of
title, author, and year.
2) Sort the array by year
3) Print the object. Override the toString in Book to match the example output:
@Javadoc Declaration Console X Properties
Book [Java Application] /Users/kuan/.p2/pool/plugins/org.eclipse.justj.openjdk.hotspo
[Book: year=1901, Book: year=2010]
Q5-The efficiency of a 200 KVA, single phase transformer is 98% when operating at full load 0.8
lagging p.f. the iron losses in the transformer is 2000 watt. Calculate the i) Full load copper losses ii)
half load copper losses and efficiency at half load. Ans: 1265.306 watt, 97.186%
Chapter 7 Solutions
Essentials of Computer Organization and Architecture
Ch. 7.7A - Prob. 1ECh. 7.7A - Prob. 2ECh. 7.7A - Prob. 3ECh. 7.7A - Prob. 5ECh. 7.7A - Prob. 6ECh. 7.7A - Prob. 8ECh. 7.7A - Prob. 9ECh. 7.7A - Prob. 10ECh. 7 - Prob. 1RETCCh. 7 - Prob. 2RETC
Ch. 7 - Prob. 3RETCCh. 7 - Prob. 4RETCCh. 7 - Prob. 5RETCCh. 7 - Prob. 6RETCCh. 7 - Prob. 7RETCCh. 7 - Prob. 8RETCCh. 7 - Prob. 9RETCCh. 7 - Prob. 10RETCCh. 7 - Prob. 11RETCCh. 7 - Prob. 12RETCCh. 7 - Prob. 13RETCCh. 7 - Prob. 14RETCCh. 7 - Prob. 15RETCCh. 7 - Prob. 16RETCCh. 7 - Prob. 17RETCCh. 7 - Prob. 18RETCCh. 7 - Prob. 19RETCCh. 7 - Prob. 20RETCCh. 7 - Prob. 21RETCCh. 7 - Prob. 22RETCCh. 7 - Prob. 23RETCCh. 7 - Prob. 24RETCCh. 7 - Prob. 25RETCCh. 7 - Prob. 26RETCCh. 7 - Prob. 27RETCCh. 7 - Prob. 28RETCCh. 7 - Prob. 29RETCCh. 7 - Prob. 30RETCCh. 7 - Prob. 31RETCCh. 7 - Prob. 32RETCCh. 7 - Prob. 33RETCCh. 7 - Prob. 34RETCCh. 7 - Prob. 35RETCCh. 7 - Prob. 36RETCCh. 7 - Prob. 37RETCCh. 7 - Prob. 38RETCCh. 7 - Prob. 39RETCCh. 7 - Prob. 40RETCCh. 7 - Prob. 41RETCCh. 7 - Prob. 42RETCCh. 7 - Prob. 43RETCCh. 7 - Prob. 44RETCCh. 7 - Prob. 45RETCCh. 7 - Prob. 46RETCCh. 7 - Prob. 47RETCCh. 7 - Prob. 48RETCCh. 7 - Prob. 49RETCCh. 7 - Prob. 1ECh. 7 - Prob. 2ECh. 7 - Prob. 3ECh. 7 - Prob. 4ECh. 7 - Prob. 5ECh. 7 - Prob. 6ECh. 7 - Prob. 7ECh. 7 - Prob. 8ECh. 7 - Prob. 9ECh. 7 - Prob. 10ECh. 7 - Prob. 11ECh. 7 - Prob. 12ECh. 7 - Prob. 13ECh. 7 - Prob. 14ECh. 7 - Prob. 15ECh. 7 - Prob. 16ECh. 7 - Prob. 17ECh. 7 - Prob. 18ECh. 7 - Prob. 19ECh. 7 - Prob. 20ECh. 7 - Prob. 21ECh. 7 - Prob. 22ECh. 7 - Prob. 23ECh. 7 - Prob. 24ECh. 7 - Prob. 25ECh. 7 - Prob. 26ECh. 7 - Prob. 27ECh. 7 - Prob. 28ECh. 7 - Prob. 29ECh. 7 - Prob. 30ECh. 7 - Prob. 31ECh. 7 - Prob. 32ECh. 7 - Prob. 33ECh. 7 - Prob. 34ECh. 7 - Prob. 35ECh. 7 - Prob. 36ECh. 7 - Prob. 37ECh. 7 - Prob. 38ECh. 7 - Prob. 39ECh. 7 - Prob. 40ECh. 7 - Prob. 41ECh. 7 - Prob. 42ECh. 7 - Prob. 43ECh. 7 - Prob. 44ECh. 7 - Prob. 45ECh. 7 - Prob. 46ECh. 7 - Prob. 47ECh. 7 - Prob. 48ECh. 7 - Prob. 49E
Knowledge Booster
Learn more about
Need a deep-dive on the concept behind this application? Look no further. Learn more about this topic, computer-science and related others by exploring similar questions and additional content below.Similar questions
- 2. Consider the following pseudocode for partition: function partition (A,L,R) pivotkey = A [R] t = L for i L to R-1 inclusive: if A[i] A[i] t = t + 1 end if end for A [t] A[R] return t end function Suppose we call partition (A,0,5) on A=[10,1,9,2,8,5]. Show the state of the list at the indicated instances. Initial A After i=0 ends After 1 ends After i 2 ends After i = 3 ends After i = 4 ends After final swap 10 19 285 [12 pts]arrow_forward.NET Interactive Solving Sudoku using Grover's Algorithm We will now solve a simple problem using Grover's algorithm, for which we do not necessarily know the solution beforehand. Our problem is a 2x2 binary sudoku, which in our case has two simple rules: •No column may contain the same value twice •No row may contain the same value twice If we assign each square in our sudoku to a variable like so: 1 V V₁ V3 V2 we want our circuit to output a solution to this sudoku. Note that, while this approach of using Grover's algorithm to solve this problem is not practical (you can probably find the solution in your head!), the purpose of this example is to demonstrate the conversion of classical decision problems into oracles for Grover's algorithm. Turning the Problem into a Circuit We want to create an oracle that will help us solve this problem, and we will start by creating a circuit that identifies a correct solution, we simply need to create a classical function on a quantum circuit that…arrow_forwardusing r languagearrow_forward
- 8. Cash RegisterThis exercise assumes you have created the RetailItem class for Programming Exercise 5. Create a CashRegister class that can be used with the RetailItem class. The CashRegister class should be able to internally keep a list of RetailItem objects. The class should have the following methods: A method named purchase_item that accepts a RetailItem object as an argument. Each time the purchase_item method is called, the RetailItem object that is passed as an argument should be added to the list. A method named get_total that returns the total price of all the RetailItem objects stored in the CashRegister object’s internal list. A method named show_items that displays data about the RetailItem objects stored in the CashRegister object’s internal list. A method named clear that should clear the CashRegister object’s internal list. Demonstrate the CashRegister class in a program that allows the user to select several items for purchase. When the user is ready to check out, the…arrow_forward5. RetailItem ClassWrite a class named RetailItem that holds data about an item in a retail store. The class should store the following data in attributes: item description, units in inventory, and price. Once you have written the class, write a program that creates three RetailItem objects and stores the following data in them: Description Units in Inventory PriceItem #1 Jacket 12 59.95Item #2 Designer Jeans 40 34.95Item #3 Shirt 20 24.95arrow_forwardFind the Error: class Information: def __init__(self, name, address, age, phone_number): self.__name = name self.__address = address self.__age = age self.__phone_number = phone_number def main(): my_info = Information('John Doe','111 My Street', \ '555-555-1281')arrow_forward
- Find the Error: class Pet def __init__(self, name, animal_type, age) self.__name = name; self.__animal_type = animal_type self.__age = age def set_name(self, name) self.__name = name def set_animal_type(self, animal_type) self.__animal_type = animal_typearrow_forwardTask 2: Comparable Interface and Record (10 Points) 1. You are tasked with creating a Java record of Dog (UML is shown below). The dog record should include the dog's name, breed, age, and weight. You are required to implement the Comparable interface for the Dog record so that you can sort the records based on the dogs' ages. Create a Java record named Dog.java. name: String breed: String age: int weight: double + toString(): String > Dog + compareTo(otherDog: Dog): int > Comparable 2. In the Dog record, establish a main method and proceed to generate an array named dogList containing three Dog objects, each with the following attributes: Dog1: name: "Buddy", breed: "Labrador Retriever", age: 5, weight: 25.5 Dog2: name: "Max", breed: "Golden Retriever", age: 3, weight: 30 Dog3: name: "Charlie", breed: "German Shepherd", age: 2, weight: 22 3. Print the dogs in dogList before sorting the dogList by age. (Please check the example output for the format). • 4. Sort the dogList using…arrow_forwardThe OSI (Open Systems Interconnection) model is a conceptual framework that standardises the functions of a telecommunication or computing system into seven distinct layers, facilitating communication and interoperability between diverse network protocols and technologies. Discuss the OSI model's physical layer specifications when designing the physical network infrastructure for a new office.arrow_forward
- In a network, information about how to reach other IP networks or hosts is stored in a device's routing table. Each entry in the routing table provides a specific path to a destination, enabling the router to forward data efficiently across the network. The routing table contains key parameters determining the available routes and how traffic is directed toward its destination. Briefly explain the main parameters that define a routing entry.arrow_forwardYou are troubleshooting a network issue where an employee's computer cannot connect to the corporate network. The computer is connected to the network via an Ethernet cable that runs to a switch. Suspecting a possible layer 1 or layer 2 problem, you decide to check the LED status indicators on both the computer's NIC and the corresponding port on the switch. Describe five LED link states and discuss what each indicates to you as a network technician.arrow_forwardYou are a network expert tasked with upgrading the network infrastructure for a growing company expanding its operations across multiple floors. The new network setup needs to support increased traffic and future scalability and provide flexibility for network management. The company is looking to implement Ethernet switches to connect various devices, including workstations, printers, and IP cameras. As part of your task, you must select the appropriate types of Ethernet switches to meet the company's needs. Evaluate the general Ethernet switch categories you would consider for this project, including their features and how they differ.arrow_forward
arrow_back_ios
SEE MORE QUESTIONS
arrow_forward_ios
Recommended textbooks for you
- Database System ConceptsComputer ScienceISBN:9780078022159Author:Abraham Silberschatz Professor, Henry F. Korth, S. SudarshanPublisher:McGraw-Hill EducationStarting Out with Python (4th Edition)Computer ScienceISBN:9780134444321Author:Tony GaddisPublisher:PEARSONDigital Fundamentals (11th Edition)Computer ScienceISBN:9780132737968Author:Thomas L. FloydPublisher:PEARSON
- C How to Program (8th Edition)Computer ScienceISBN:9780133976892Author:Paul J. Deitel, Harvey DeitelPublisher:PEARSONDatabase Systems: Design, Implementation, & Manag...Computer ScienceISBN:9781337627900Author:Carlos Coronel, Steven MorrisPublisher:Cengage LearningProgrammable Logic ControllersComputer ScienceISBN:9780073373843Author:Frank D. PetruzellaPublisher:McGraw-Hill Education
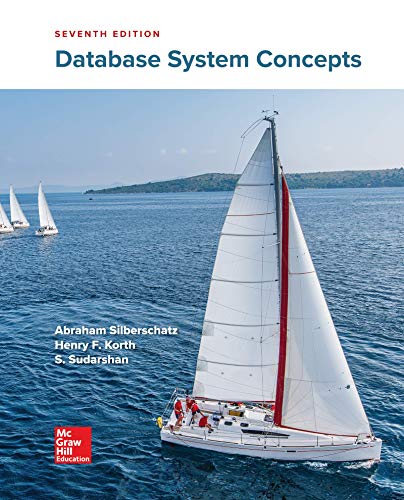
Database System Concepts
Computer Science
ISBN:9780078022159
Author:Abraham Silberschatz Professor, Henry F. Korth, S. Sudarshan
Publisher:McGraw-Hill Education
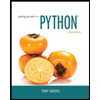
Starting Out with Python (4th Edition)
Computer Science
ISBN:9780134444321
Author:Tony Gaddis
Publisher:PEARSON
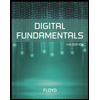
Digital Fundamentals (11th Edition)
Computer Science
ISBN:9780132737968
Author:Thomas L. Floyd
Publisher:PEARSON
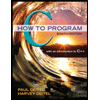
C How to Program (8th Edition)
Computer Science
ISBN:9780133976892
Author:Paul J. Deitel, Harvey Deitel
Publisher:PEARSON
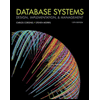
Database Systems: Design, Implementation, & Manag...
Computer Science
ISBN:9781337627900
Author:Carlos Coronel, Steven Morris
Publisher:Cengage Learning
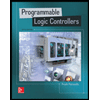
Programmable Logic Controllers
Computer Science
ISBN:9780073373843
Author:Frank D. Petruzella
Publisher:McGraw-Hill Education