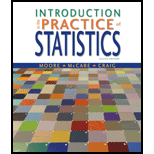
Concept explainers
(a)
To find: The predicted count values.
(a)

Answer to Problem 71E
Solution: The predicted count values are:
Explanation of Solution
Calculation: The provided least-squares regression equation is:
The time values are provided in the data as 1, 3, 5 and 7.
Substituting the values of time in the above linear regression equation, the following results are obtained:
For
For
For
For
Hence, the predicted count values obtained are
(b)
Section 1
To find: The difference between the observed and the predicted counts.
(b)
Section 1

Answer to Problem 71E
Solution: The differences obtained are
Explanation of Solution
Calculation: The respective observed counts are provided in the exercise as:
for the times
The respective predicted counts for the above times are obtained from part (a) as:
The differences
For
For
For
For
Hence, the differences obtained are
Section 2
The number of positive differences between the observed and the predicted counts.
Section 2

Answer to Problem 71E
Solution: The two positive differences are
Explanation of Solution
Clearly, the positive difference values are
The number of negative differences between the observed and the predicted counts.

Answer to Problem 71E
Solution: The two negative differences are
Explanation of Solution
Clearly, the negative difference values are
(c)
Section 1
To find: Squares of the differences obtained in part(b).
(c)
Section 1

Answer to Problem 71E
Solution: The squares of the differences are:
Explanation of Solution
Calculation: The differences obtained in the part (b) above are:
The squares of the differences obtained are calculated as follows:
The square of the difference
The square of the difference
The square of the difference
The square of the difference
Hence, the squares of the differences obtained are
Section 2
To find: The sum of the squares of the differences obtained in Section 1 above.
Section 2

Answer to Problem 71E
Solution: The sum of the differences obtained is
Explanation of Solution
Calculation: The squares of the differences are obtained in Section 1 above as:
The sum of the differences
Hence, the sum of the differences obtained is
(d)
Section 1
To find: The predicted count values.
(d)
Section 1

Answer to Problem 71E
Solution: The predicted count values obtained are:
Explanation of Solution
Calculation: The provided least-squares regression equation is:
The time values are provided in the data as 1, 3, 5 and 7.
Substituting the values of time in the above linear regression equation, the following results are obtained:
For
For
For
For
Hence, the predicted count values obtained are:
For the times 1, 3, 5 and 7 respectively.
Section 2:
To find: The difference between the observed and the predicted counts.
Section 2:

Answer to Problem 71E
Solution: The differences obtained are
Explanation of Solution
The differences
For
For
For
For
Hence, the differences obtained are
Section 3:
The number of positive differences between the observed and the predicted counts.
Section 3:

Answer to Problem 71E
Solution: The four positive differences are
Explanation of Solution
Clearly, all the difference values are positive. Hence, all the 4 differences
The number of negative differences between the observed and the predicted counts.

Answer to Problem 71E
Solution: There are no negative differences.
Explanation of Solution
Clearly, none of the values are negative. Hence, 0 out of 4 differences are negative.
Section 4:
To find: Squares of the differences obtained in section 2 of part (d).
Section 4:

Answer to Problem 71E
Solution: The squares of the differences are:
Explanation of Solution
Calculation: The differences obtained in the section 2 of part (d) above are:
The squares of the differences obtained are calculated as follows:
The square of the difference
The square of the difference
The square of the difference
The square of the difference
Hence, the squares of the differences obtained are
Section 5:
To find: The sum of the squares of the differences obtained in Section 3 above.
Section 5:

Answer to Problem 71E
Solution: The sum of the differences is
Explanation of Solution
The sum of the differences
Hence, the sum of the differences is
(e)
To explain: The least-squares inference based on the calculations performed.
(e)

Answer to Problem 71E
Solution: The following least-square regression is a better measure of the relationship between the count and the time:
Explanation of Solution
The differences (residuals) are both positive and negative values, whereas, for the regression line,
all the differences (residuals) are high and positive values. Thus, the residual plots for the first regression line will lie both below and above the x-axis. Also, its predicted regression line will lie much near to the observed regression line. Whereas, the residual plots for the second regression line will lie only above the x-axis. Also, with such high and positive differences, the predicted regression line will lie far to the observed regression line. Hence, the first line better depicts the relationship between the count and the time because it gives a better approximate value of the response variable.
Want to see more full solutions like this?
Chapter 2 Solutions
Introduction to the Practice of Statistics: w/CrunchIt/EESEE Access Card
- An electronics company manufactures batches of n circuit boards. Before a batch is approved for shipment, m boards are randomly selected from the batch and tested. The batch is rejected if more than d boards in the sample are found to be faulty. a) A batch actually contains six faulty circuit boards. Find the probability that the batch is rejected when n = 20, m = 5, and d = 1. b) A batch actually contains nine faulty circuit boards. Find the probability that the batch is rejected when n = 30, m = 10, and d = 1.arrow_forwardTwenty-eight applicants interested in working for the Food Stamp program took an examination designed to measure their aptitude for social work. A stem-and-leaf plot of the 28 scores appears below, where the first column is the count per branch, the second column is the stem value, and the remaining digits are the leaves. a) List all the values. Count 1 Stems Leaves 4 6 1 4 6 567 9 3688 026799 9 8 145667788 7 9 1234788 b) Calculate the first quartile (Q1) and the third Quartile (Q3). c) Calculate the interquartile range. d) Construct a boxplot for this data.arrow_forwardPam, Rob and Sam get a cake that is one-third chocolate, one-third vanilla, and one-third strawberry as shown below. They wish to fairly divide the cake using the lone chooser method. Pam likes strawberry twice as much as chocolate or vanilla. Rob only likes chocolate. Sam, the chooser, likes vanilla and strawberry twice as much as chocolate. In the first division, Pam cuts the strawberry piece off and lets Rob choose his favorite piece. Based on that, Rob chooses the chocolate and vanilla parts. Note: All cuts made to the cake shown below are vertical.Which is a second division that Rob would make of his share of the cake?arrow_forward
- Three players (one divider and two choosers) are going to divide a cake fairly using the lone divider method. The divider cuts the cake into three slices (s1, s2, and s3). If the choosers' declarations are Chooser 1: {s1 , s2} and Chooser 2: {s2 , s3}. Using the lone-divider method, how many different fair divisions of this cake are possible?arrow_forwardTheorem 2.6 (The Minkowski inequality) Let p≥1. Suppose that X and Y are random variables, such that E|X|P <∞ and E|Y P <00. Then X+YpX+Yparrow_forwardTheorem 1.2 (1) Suppose that P(|X|≤b) = 1 for some b > 0, that EX = 0, and set Var X = 0². Then, for 0 0, P(X > x) ≤e-x+1²² P(|X|>x) ≤2e-1x+1²² (ii) Let X1, X2...., Xn be independent random variables with mean 0, suppose that P(X ≤b) = 1 for all k, and set oσ = Var X. Then, for x > 0. and 0x) ≤2 exp Σ k=1 (iii) If, in addition, X1, X2, X, are identically distributed, then P(S|x) ≤2 expl-tx+nt²o).arrow_forward
- Theorem 5.1 (Jensen's inequality) state without proof the Jensen's Ineg. Let X be a random variable, g a convex function, and suppose that X and g(X) are integrable. Then g(EX) < Eg(X).arrow_forwardCan social media mistakes hurt your chances of finding a job? According to a survey of 1,000 hiring managers across many different industries, 76% claim that they use social media sites to research prospective candidates for any job. Calculate the probabilities of the following events. (Round your answers to three decimal places.) answer parts a-c. a) Out of 30 job listings, at least 19 will conduct social media screening. b) Out of 30 job listings, fewer than 17 will conduct social media screening. c) Out of 30 job listings, exactly between 19 and 22 (including 19 and 22) will conduct social media screening. show all steps for probabilities please. answer parts a-c.arrow_forwardQuestion: we know that for rt. (x+ys s ا. 13. rs. and my so using this, show that it vye and EIXI, EIYO This : E (IX + Y) ≤2" (EIX (" + Ely!")arrow_forward
- Theorem 2.4 (The Hölder inequality) Let p+q=1. If E|X|P < ∞ and E|Y| < ∞, then . |EXY ≤ E|XY|||X|| ||||qarrow_forwardTheorem 7.6 (Etemadi's inequality) Let X1, X2, X, be independent random variables. Then, for all x > 0, P(max |S|>3x) ≤3 max P(S| > x). Isk≤narrow_forwardTheorem 7.2 Suppose that E X = 0 for all k, that Var X = 0} x) ≤ 2P(S>x 1≤k≤n S√2), -S√2). P(max Sk>x) ≤ 2P(|S|>x- 1arrow_forwardarrow_back_iosSEE MORE QUESTIONSarrow_forward_ios
- MATLAB: An Introduction with ApplicationsStatisticsISBN:9781119256830Author:Amos GilatPublisher:John Wiley & Sons IncProbability and Statistics for Engineering and th...StatisticsISBN:9781305251809Author:Jay L. DevorePublisher:Cengage LearningStatistics for The Behavioral Sciences (MindTap C...StatisticsISBN:9781305504912Author:Frederick J Gravetter, Larry B. WallnauPublisher:Cengage Learning
- Elementary Statistics: Picturing the World (7th E...StatisticsISBN:9780134683416Author:Ron Larson, Betsy FarberPublisher:PEARSONThe Basic Practice of StatisticsStatisticsISBN:9781319042578Author:David S. Moore, William I. Notz, Michael A. FlignerPublisher:W. H. FreemanIntroduction to the Practice of StatisticsStatisticsISBN:9781319013387Author:David S. Moore, George P. McCabe, Bruce A. CraigPublisher:W. H. Freeman
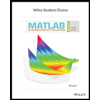
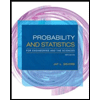
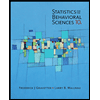
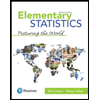
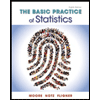
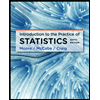