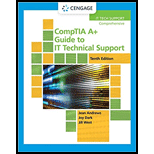
Bundle: Comptia A+ Guide To It Technical Support, 10th + Mindtap, 1 Term Printed Access Card
10th Edition
ISBN: 9780357012789
Author: Jean Andrews, Joy Dark, Jill West
Publisher: Cengage Learning
expand_more
expand_more
format_list_bulleted
Concept explainers
Expert Solution & Answer
Chapter 2, Problem 12TC
Explanation of Solution
Given:
An Intel Core i7-7700 processor and a Gigabyte GA-H110M-S2 motherboard.
To find: The steps to be taken before installing Intel Core i7-7700 processor on a Gigabyte GA-H110M-S2 motherboard...
Expert Solution & Answer

Trending nowThis is a popular solution!

Students have asked these similar questions
You are called by your supervisor to go and check a potential data bridge problem. What are the stepsyou will follow in order to check the database and fix any problems with it? Have in mind that youSHOULD normalize it as well. Describe in full, consider the following taking the database offline is not allowed since people are connected to it and how personal data might be bridged and not secured.Provide three references with you answer.
You are called by your supervisor to go and check a potential data bridge problem. What are the stepsyou will follow in order to check the database and fix any problems with it? Have in mind that youSHOULD normalize it as well. Describe in full, consider the following:• Taking the database offline is not allowed since people are connected to it.• Personal data might be bridged and not secured.
Provide three refernces with you answer
You are called by your supervisor to go and check a potential data bridge problem. What are the stepsyou will follow in order to check the database and fix any problems with it? Have in mind that youSHOULD normalize it as well. Describe in full, consider the following:• Taking the database offline is not allowed since people are connected to it.• Personal data might be bridged and not secured.
Provide three refernces with you answer from websites
Chapter 2 Solutions
Bundle: Comptia A+ Guide To It Technical Support, 10th + Mindtap, 1 Term Printed Access Card
Knowledge Booster
Learn more about
Need a deep-dive on the concept behind this application? Look no further. Learn more about this topic, computer-science and related others by exploring similar questions and additional content below.Similar questions
- Modern life has been impacted immensely by computers. Computers have penetrated every aspect of oursociety, either for better or for worse. From supermarket scanners calculating our shopping transactionswhile keeping store inventory; robots that handle highly specialized tasks or even simple human tasks,computers do much more than just computing. But where did all this technology come from and whereis it heading? Does the future look promising or should we worry about computers taking over theworld? Or are they just a necessary evil? Provide three references with your answer.arrow_forwardObjective: 1. Implement a custom Vector class in C++ that manages dynamic memory efficiently. 2. Demonstrate an understanding of the Big Five by managing deep copies, move semantics, and resource cleanup. 3. Explore the performance trade-offs between heap and stack allocation. Task Description: Part 1: Custom Vector Implementation 1. Create a Vector class that manages a dynamically allocated array. 。 Member Variables: ° T✶ data; // Dynamically allocated array for storage. std::size_t size; // Number of elements currently in the vector. std::size_t capacity; // Maximum number of elements before reallocation is required. 2. Implement the following core member functions: Default Constructor: Initialize an empty vector with no allocated storage. 。 Destructor: Free any dynamically allocated memory. 。 Copy Constructor: Perform a deep copy of the data array. 。 Copy Assignment Operator: Free existing resources and perform a deep copy. Move Constructor: Transfer ownership of the data array…arrow_forward2.68♦♦ Write code for a function with the following prototype: * Mask with least signficant n bits set to 1 * Examples: n = 6 -> 0x3F, n = 17-> 0x1FFFF * Assume 1 <= n <= w int lower_one_mask (int n); Your function should follow the bit-level integer coding rules Be careful of the case n = W.arrow_forward
- Hi-Volt Components You are the IT manager at Hi-Voltage Components, a medium-sized firm that makes specialized circuit boards. Hi-Voltage's largest customer, Green Industries, recently installed a computerized purchasing sys- tem. If Hi-Voltage connects to the purchasing system, Green Industries will be able to submit purchase orders electronically. Although Hi-Voltage has a computerized accounting system, that system is not capable of handling EDI. Tasks 1. What options does Hi-Voltage have for developing a system to connect with Green Industries' pur- chasing system? 2. What terms or concepts describe the proposed computer-to-computer relationship between Hi-Voltage and Green Industries? why not? 3. Would Hi-Voltage's proposed new system be a transaction processing system? Why or 4. Before Hi-Voltage makes a final decision, should the company consider an ERP system? Why or why not?arrow_forwardConsider the following expression in C: a/b > 0 && b/a > 0.What will be the result of evaluating this expression when a is zero? What will be the result when b is zero? Would it make sense to try to design a language in which this expression is guaranteed to evaluate to false when either a or b (but not both) is zero? Explain your answerarrow_forwardConsider the following expression in C: a/b > 0 && b/a > 0. What will be the result of evaluating this expression when a is zero? What will be the result when b is zero? Would it make sense to try to design a language in which this expression is guaranteed to evaluate to false when either a or b (but not both) is zero? Explain your answer.arrow_forward
- What are the major threats of using the internet? How do you use it? How do children use it? How canwe secure it? Provide four references with your answer. Two of the refernces can be from an article and the other two from websites.arrow_forwardAssume that a string of name & surname is saved in S. The alphabetical characters in S can be in lowercase and/or uppercase letters. Name and surname are assumed to be separated by a space character and the string ends with a full stop "." character. Write an assembly language program that will copy the name to NAME in lowercase and the surname to SNAME in uppercase letters. Assume that name and/or surname cannot exceed 20 characters. The program should be general and work with every possible string with name & surname. However, you can consider the data segment definition given below in your program. .DATA S DB 'Mahmoud Obaid." NAME DB 20 DUP(?) SNAME DB 20 DUP(?) Hint: Uppercase characters are ordered between 'A' (41H) and 'Z' (5AH) and lowercase characters are ordered between 'a' (61H) and 'z' (7AH) in the in the ASCII Code table. For lowercase letters, bit 5 (d5) of the ASCII code is 1 where for uppercase letters it is 0. For example, Letter 'h' Binary ASCII 01101000 68H 'H'…arrow_forwardWhat did you find most interesting or surprising about the scientist Lavoiser?arrow_forward
- 1. Complete the routing table for R2 as per the table shown below when implementing RIP routing Protocol? (14 marks) 195.2.4.0 130.10.0.0 195.2.4.1 m1 130.10.0.2 mo R2 R3 130.10.0.1 195.2.5.1 195.2.5.0 195.2.5.2 195.2.6.1 195.2.6.0 m2 130.11.0.0 130.11.0.2 205.5.5.0 205.5.5.1 R4 130.11.0.1 205.5.6.1 205.5.6.0arrow_forwardAnalyze the charts and introduce each charts by describing each. Identify the patterns in the given data. And determine how are the data points are related. Refer to the raw data (table):arrow_forward3A) Generate a hash table for the following values: 11, 9, 6, 28, 19, 46, 34, 14. Assume the table size is 9 and the primary hash function is h(k) = k % 9. i) Hash table using quadratic probing ii) Hash table with a secondary hash function of h2(k) = 7- (k%7) 3B) Demonstrate with a suitable example, any three possible ways to remove the keys and yet maintaining the properties of a B-Tree. 3C) Differentiate between Greedy and Dynamic Programming.arrow_forward
arrow_back_ios
SEE MORE QUESTIONS
arrow_forward_ios
Recommended textbooks for you
- A+ Guide To It Technical SupportComputer ScienceISBN:9780357108291Author:ANDREWS, Jean.Publisher:Cengage,A+ Guide to Hardware (Standalone Book) (MindTap C...Computer ScienceISBN:9781305266452Author:Jean AndrewsPublisher:Cengage LearningComptia A+ Core 1 Exam: Guide To Computing Infras...Computer ScienceISBN:9780357108376Author:Jean Andrews, Joy Dark, Jill WestPublisher:Cengage Learning
- Np Ms Office 365/Excel 2016 I NtermedComputer ScienceISBN:9781337508841Author:CareyPublisher:CengageCompTIA Linux+ Guide to Linux Certification (Mind...Computer ScienceISBN:9781305107168Author:Jason EckertPublisher:Cengage LearningSystems ArchitectureComputer ScienceISBN:9781305080195Author:Stephen D. BurdPublisher:Cengage Learning
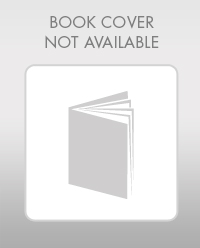
A+ Guide To It Technical Support
Computer Science
ISBN:9780357108291
Author:ANDREWS, Jean.
Publisher:Cengage,
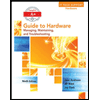
A+ Guide to Hardware (Standalone Book) (MindTap C...
Computer Science
ISBN:9781305266452
Author:Jean Andrews
Publisher:Cengage Learning
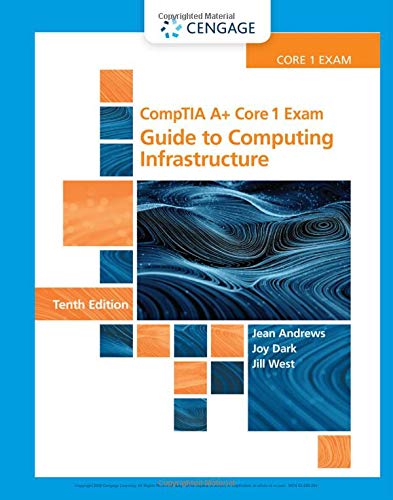
Comptia A+ Core 1 Exam: Guide To Computing Infras...
Computer Science
ISBN:9780357108376
Author:Jean Andrews, Joy Dark, Jill West
Publisher:Cengage Learning
Np Ms Office 365/Excel 2016 I Ntermed
Computer Science
ISBN:9781337508841
Author:Carey
Publisher:Cengage
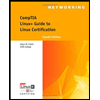
CompTIA Linux+ Guide to Linux Certification (Mind...
Computer Science
ISBN:9781305107168
Author:Jason Eckert
Publisher:Cengage Learning
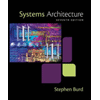
Systems Architecture
Computer Science
ISBN:9781305080195
Author:Stephen D. Burd
Publisher:Cengage Learning