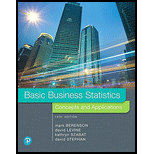
EBK BASIC BUSINESS STATISTICS
14th Edition
ISBN: 9780134685168
Author: STEPHAN
Publisher: YUZU
expand_more
expand_more
format_list_bulleted
Question
Chapter 16, Problem 39PS
a.
To determine
Perform a residual analysis.
b.
To determine
Compute
c.
To determine
Compute the MAD.
d.
To determine
Discuss whether the linear trend forecast is appropriate or not.
Expert Solution & Answer

Want to see the full answer?
Check out a sample textbook solution
Students have asked these similar questions
Please could you explain why 0.5 was added to each upper limpit of the intervals.Thanks
28. (a) Under what conditions do we say that two random variables X and Y are
independent?
(b) Demonstrate that if X and Y are independent, then it follows that E(XY) =
E(X)E(Y);
(e) Show by a counter example that the converse of (ii) is not necessarily true.
1. Let X and Y be random variables and suppose that A = F. Prove that
Z XI(A)+YI(A) is a random variable.
Chapter 16 Solutions
EBK BASIC BUSINESS STATISTICS
Ch. 16 - If you are using exponential smoothing for...Ch. 16 - Consider a nine-year moving average used to smooth...Ch. 16 - You are using exponential smoothing on an annual...Ch. 16 - Prob. 4PSCh. 16 - Prob. 5PSCh. 16 - How have stocks performed in the past? The...Ch. 16 - Prob. 7PSCh. 16 - Prob. 8PSCh. 16 - Prob. 9PSCh. 16 - Prob. 10PS
Ch. 16 - The linear trend forecasting equation for an...Ch. 16 - There has been much publicity about bounces paid...Ch. 16 - Prob. 13PSCh. 16 - Prob. 14PSCh. 16 - Prob. 15PSCh. 16 - The data shown in the following table and stored...Ch. 16 - Prob. 17PSCh. 16 - Prob. 18PSCh. 16 - Prob. 19PSCh. 16 - Prob. 20PSCh. 16 - Prob. 21PSCh. 16 - Prob. 22PSCh. 16 - You are given an annual time series with 40...Ch. 16 - Prob. 24PSCh. 16 - Prob. 25PSCh. 16 - Prob. 26PSCh. 16 - Prob. 27PSCh. 16 - Prob. 28PSCh. 16 - Prob. 29PSCh. 16 - Using the average baseball salary from 200 through...Ch. 16 - Using the yearly amount of solar power generated...Ch. 16 - The following residuals are from a linear trend...Ch. 16 - Prob. 33PSCh. 16 - Prob. 34PSCh. 16 - Prob. 35PSCh. 16 - Prob. 36PSCh. 16 - Prob. 37PSCh. 16 - Prob. 38PSCh. 16 - Prob. 39PSCh. 16 - Prob. 40PSCh. 16 - In forecasting daily time-series data, how many...Ch. 16 - In forecasting a quarterly time series over the...Ch. 16 - Prob. 43PSCh. 16 - Prob. 44PSCh. 16 - Are gasoline prices higher during the height of...Ch. 16 - Prob. 46PSCh. 16 - Prob. 47PSCh. 16 - The file Silver-Q contains the price in London for...Ch. 16 - Prob. 49PSCh. 16 - What is a time series?Ch. 16 - What are the different components of a time-series...Ch. 16 - What is the difference between moving average and...Ch. 16 - Prob. 53PSCh. 16 - How does the least-squares linear trend...Ch. 16 - How does autoregressive modelling differ from the...Ch. 16 - What are the different approaches to choosing an...Ch. 16 - What is the major difference between using SYX and...Ch. 16 - How does forecasting for monthly or quarterly data...Ch. 16 - Prob. 60PSCh. 16 - The monthly commercial and residential prices for...Ch. 16 - The data stored in McDonalds represent the gross...Ch. 16 - Teachers’ Retirement System of the City of New...Ch. 16 - Prob. 64PS
Knowledge Booster
Learn more about
Need a deep-dive on the concept behind this application? Look no further. Learn more about this topic, statistics and related others by exploring similar questions and additional content below.Similar questions
- (c) Utilize Fubini's Theorem to demonstrate that E(X)= = (1- F(x))dx.arrow_forward(c) Describe the positive and negative parts of a random variable. How is the integral defined for a general random variable using these components?arrow_forward26. (a) Provide an example where X, X but E(X,) does not converge to E(X).arrow_forward
- (b) Demonstrate that if X and Y are independent, then it follows that E(XY) E(X)E(Y);arrow_forward(d) Under what conditions do we say that a random variable X is integrable, specifically when (i) X is a non-negative random variable and (ii) when X is a general random variable?arrow_forward29. State the Borel-Cantelli Lemmas without proof. What is the primary distinction between Lemma 1 and Lemma 2?arrow_forward
arrow_back_ios
SEE MORE QUESTIONS
arrow_forward_ios
Recommended textbooks for you
- Functions and Change: A Modeling Approach to Coll...AlgebraISBN:9781337111348Author:Bruce Crauder, Benny Evans, Alan NoellPublisher:Cengage LearningCollege AlgebraAlgebraISBN:9781305115545Author:James Stewart, Lothar Redlin, Saleem WatsonPublisher:Cengage Learning
- Algebra and Trigonometry (MindTap Course List)AlgebraISBN:9781305071742Author:James Stewart, Lothar Redlin, Saleem WatsonPublisher:Cengage LearningLinear Algebra: A Modern IntroductionAlgebraISBN:9781285463247Author:David PoolePublisher:Cengage LearningAlgebra & Trigonometry with Analytic GeometryAlgebraISBN:9781133382119Author:SwokowskiPublisher:Cengage
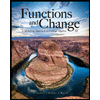
Functions and Change: A Modeling Approach to Coll...
Algebra
ISBN:9781337111348
Author:Bruce Crauder, Benny Evans, Alan Noell
Publisher:Cengage Learning
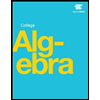
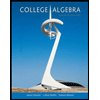
College Algebra
Algebra
ISBN:9781305115545
Author:James Stewart, Lothar Redlin, Saleem Watson
Publisher:Cengage Learning
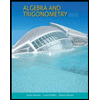
Algebra and Trigonometry (MindTap Course List)
Algebra
ISBN:9781305071742
Author:James Stewart, Lothar Redlin, Saleem Watson
Publisher:Cengage Learning
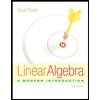
Linear Algebra: A Modern Introduction
Algebra
ISBN:9781285463247
Author:David Poole
Publisher:Cengage Learning
Algebra & Trigonometry with Analytic Geometry
Algebra
ISBN:9781133382119
Author:Swokowski
Publisher:Cengage
Time Series Analysis Theory & Uni-variate Forecasting Techniques; Author: Analytics University;https://www.youtube.com/watch?v=_X5q9FYLGxM;License: Standard YouTube License, CC-BY
Operations management 101: Time-series, forecasting introduction; Author: Brandoz Foltz;https://www.youtube.com/watch?v=EaqZP36ool8;License: Standard YouTube License, CC-BY