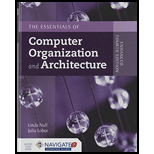
Explanation of Solution
Verification of the ratio being consistent when compared with the other system:
- System performance is considered as one of main factor of a processor.
- It is used to determine the speed a problem can be solved.
- It is also used to determine the factors such as number of problems that can be allocated at particular amount of time and also the number of problems that can be handled by the processor.
- The relative performance between the two systems is measured and expected by the run time of the
program of the individual.
Given:
The information about the system A and System c are shown in the below table:
Program |
Execution time System A(sec) |
Execution time System B(sec) |
Execution time System C(sec) |
V | 50 | 100 | 500 |
W | 200 | 400 | 600 |
X | 250 | 500 | 500 |
Y | 400 | 800 | 800 |
Z | 5000 | 4100 | 3500 |
Consider there are n programs and each programs are considered to have their own runtime on each systems.
The geometric mean of the one system’s runtime is obtained by normalizing it with the another system.
The process of normalization is carried out by taking the products of the ratio of the run time and by taking the nth root of the product.
The below tables illustrates the how the system B and system C is being normalized with that of the system A.
Program |
Execution time System A(sec) | Normalized to A |
Execution time System B(sec) | Normalized to B |
Execution time System C(sec) | Normalized to C |
V | 50 | 1 | 100 | 0.5 | 500 | 0.1 |
W | 200 | 1 | 400 | 0.5 | 600 | 0.33 |
X | 250 | 1 | 500 | 0.5 | 500 | 0.5 |
Y | 400 | 1 | 800 | 0.6 | 800 | 0.5 |
Z | 5000 | 1 | 4100 | 1.22 | 3500 | 1.43 |
Calculating geometic Mean:
The formula to calculate the geometric mean:
System A:
calculate the geometric mean for the system A:
System B:
calculate the geometric mean for the system B:

Want to see the full answer?
Check out a sample textbook solution
Chapter 11 Solutions
Essentials of Computer Organization and Architecture
- using r languagearrow_forwardWhat significant justification is there for the -> operator in C and C++?arrow_forwardMultidimensional arrays can be stored in row major order, as in C++, or in column major order, as in Fortran. Develop the access functions for both of these arrangements for three-dimensional arrays.arrow_forward
- What are the arguments for and against Java’s implicit heap storage recovery, when compared with the explicit heap storage recovery required in C++? Consider real-time systems.arrow_forward8. Name and Email AddressesWrite a program that keeps names and email addresses in a dictionary as key-value pairs. The program should display a menu that lets the user look up a person’s email address, add a new name and email address, change an existing email address, and delete an existing name and email address. The program should pickle the dictionary and save it to a file when the user exits the program. Each time the program starts, it should retrieve the dictionary from the file and unpickle it. How would the user be able to use the program?arrow_forwardPlease solve and answer the question correctly please. Thank you!!arrow_forward
- Please solve and answer the question correctly please. Thank you!!arrow_forwardW AutoSave Off File Home Insert Draw Design Assignment_1[1] - Protected... ■ Saved V > Search Layout References Mailings Review View Help QuillBot RefWorks - RCM PROTECTED VIEW Be careful-files from the Internet can contain viruses. Unless you need to edit, it's safer to stay in Protected View. Enable Editing PR Comments Viewing Share × 2. The following state transition table is a simplified model of process management, with the labels representing transitions between states of READY, RUN, BLOCKED, and NONRESIDENT. READY RUN BLOCKED NONRESIDENT READY RUN - 1 - 5 BLOCKED 2 4 3 - 6 Give an example of an event that can cause each of the above transitions. Draw a diagram if that helps. (5) Page 2 of 4 20 of 278 words Text Predictions: On 64 f W Focus + 170% ENG US 17:13 2025/03/24arrow_forwardI need help understanding how could I got the IP Address for the Last Host on this Subnet & the Broadcast Address for this Subnetarrow_forward
- I need help understanding how could I got the IP Address for the Last Host on this Subnet & the Broadcast Address for this Subnetarrow_forwardPlease answer Java OOP homework scenario below: You have been hired by the National Insurance Company to create a program to track insurance policies for all its customers. National Insurance provides three types of insurance: automobile, home and life insurance. The program should be able to track up to 100 policies. Based on the provided information, create an efficient, object-oriented solution with good design principles that will allow a user to manage policies. To do this, the solution should have a menu with the following capabilities: Add Policy o This capability will allow a user to add a policy. The user should specify if the policy is for home, auto, or life. Once the type of policy has been selected, the user should be prompted for all required fields for the policy type. Remove Policy o This capability will allow a user to select any policy and remove it from the list. View policies o This capability will allow a user to see all the policies. All policy fields…arrow_forwardWhat are the two errors in my pseudocode?Module getAverage(Integer value1, Integer value2, Integer value3) Declare Integer average average = value1 + value2 + value3 / 3 Display average End Modulearrow_forward
- Database System ConceptsComputer ScienceISBN:9780078022159Author:Abraham Silberschatz Professor, Henry F. Korth, S. SudarshanPublisher:McGraw-Hill EducationStarting Out with Python (4th Edition)Computer ScienceISBN:9780134444321Author:Tony GaddisPublisher:PEARSONDigital Fundamentals (11th Edition)Computer ScienceISBN:9780132737968Author:Thomas L. FloydPublisher:PEARSON
- C How to Program (8th Edition)Computer ScienceISBN:9780133976892Author:Paul J. Deitel, Harvey DeitelPublisher:PEARSONDatabase Systems: Design, Implementation, & Manag...Computer ScienceISBN:9781337627900Author:Carlos Coronel, Steven MorrisPublisher:Cengage LearningProgrammable Logic ControllersComputer ScienceISBN:9780073373843Author:Frank D. PetruzellaPublisher:McGraw-Hill Education
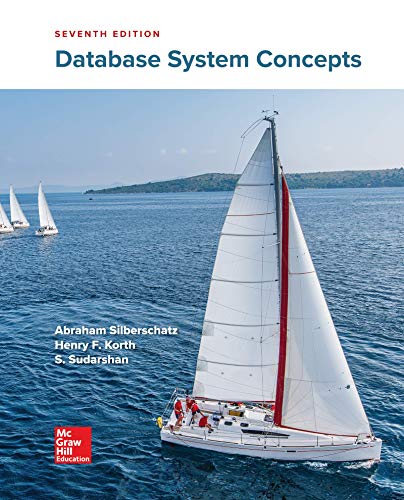
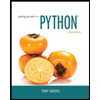
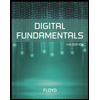
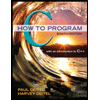
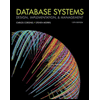
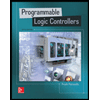