What type of significance test would you run given the data above? What conditions must be satisfied for the test you have chosen in order to get valid results? Are the conditions satisfied? Run your test for significance using the data link above. Attach the data output including the hypotheses that you have chosen. Using the p-value (or critical values & test statistic), draw a proper conclusion and write said conclusion in context. Based on your conclusion, which type of error could have been made: a Type I error or a Type II error. Justify your answer. If you were a researcher, what type of data would you be interested in collecting? What would your null and alternative hypotheses be?
Contingency Table
A contingency table can be defined as the visual representation of the relationship between two or more categorical variables that can be evaluated and registered. It is a categorical version of the scatterplot, which is used to investigate the linear relationship between two variables. A contingency table is indeed a type of frequency distribution table that displays two variables at the same time.
Binomial Distribution
Binomial is an algebraic expression of the sum or the difference of two terms. Before knowing about binomial distribution, we must know about the binomial theorem.
Do You Have a Fever?
Sometimes when you are sick, your forehead feels really warm. You might have fever. How can you find out whether you do? By taking your temperature , of course. But what temperature should the thermometer show if you're healthy? Is this temperature the same for everyone?
Several years ago, researchers conducted a study to determine whether the "accepted" value for normal body temperature, 98.6oF, is accurate. They used an oral thermometer to measure the temperatures of a random sample of healthy men and women aged 18 to 40. As is often the case, the researchers did not provide their original data.
Allen Shoemaker, from Calvin College, produced a data set with the same properties as the original temperature readings. His data set consists of one oral temperature reading for each of the 130 randomly chosen, healthy 18- to 40-year-olds. A dotplot of Shoemaker's temperature data is shown below. A vertical line at 98.6oF was added for reference.
Statcrunch Data Link (Links to an external site.)
Exploratory data analysis revealed several interesting facts about this data set:
- The mean temperature was ˉx=98.25oF
- The standard deviation of the temperature reading was
Is "normal" body temperature really 98.6oF? Use to test the claim that the average body temperature for healthy 18- to 40-year-olds is actually less than 98.6oF.
-
- What type of significance test would you run given the data above?
- What conditions must be satisfied for the test you have chosen in order to get valid results? Are the conditions satisfied?
- Run your test for significance using the data link above. Attach the data output including the hypotheses that you have chosen.
- Using the p-value (or critical values & test statistic), draw a proper conclusion and write said conclusion in context.
- Based on your conclusion, which type of error could have been made: a Type I error or a Type II error. Justify your answer.
- If you were a researcher, what type of data would you be interested in collecting? What would your null and alternative hypotheses be?
-
-
- For example: Just down from my house is a stop sign, in which most people roll through without fully stopping. My claim would be "most people fail to stop at the stop sign" with H0: p = 0.50 and H1: p > 0.50. I would watch at random times of the day and keep a tally of those who do make a full stop vs. those who roll through or fail to stop at all. My proportion would be the number of people who don't stop divided by the total observations I recorded
- Try not to simply use my example but change the words around, but instead think of your own unique hypotheses. Feel free to use an average instead if you'd like.
- For example: Just down from my house is a stop sign, in which most people roll through without fully stopping. My claim would be "most people fail to stop at the stop sign" with H0: p = 0.50 and H1: p > 0.50. I would watch at random times of the day and keep a tally of those who do make a full stop vs. those who roll through or fail to stop at all. My proportion would be the number of people who don't stop divided by the total observations I recorded
-

Step by step
Solved in 2 steps

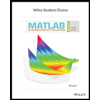
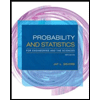
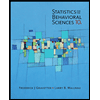
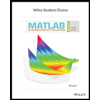
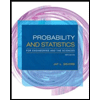
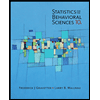
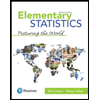
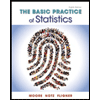
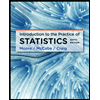