TODO 1 Let's load the forestfires.csv by using Pandas read_csv() function. The read_csv() function works by taking in a path to a csv file (e.g., /home/user/Downloads/forestfires.csv could be a Linux/Mac file path; if you are using Windows, add r before your path). For simplicity, we are just going to pass the name of the csv as this assumes the forestfires.csv is in the same path as this notebook (i.e., your local path or current directory). Recall, we printed out the current directory and path of this notebook above. Make sure the forestfires.csv is in that directory. Load Forest Fires dataset by passing the name of the csv file "forestfires.csv" to the Pandas function read_csv() (docs). Store the output into the forestfire_df variable. Note: we wrote a custom exception to alert you if you didn't move your csv file to the correct location. So if you are getting an error take a second to read what it says. Using the forestfire_df DataFrame we just defined, call the columns class variable to store all the NAMES of the features in the dataset. Store the output into the variable feature_names. Note: recall that from last weeks lab, if you put a variable at the bottom of the cell Jupyter Notebook will automatically output what is contained in said variable to the notebook's output (i.e., the Out[] line). # This line checks to make sure the forestfire.csv is in the # same directory as this notebook. if not os.path.exists("forestfires.csv"): raise Exception(f"The forestfires.csv is not detected in your local path! " \ f"You need to move the 'forestfires.csv' file to the same " \ f"location/directory as this notebook which is {os.getcwd()}") # TODO 1.1 display(forestfire_df) todo_check([ (np.all(forestfire_df.iloc[0].values == np.array([7, 5, 'mar', 'fri', 86.2, 26.2, 94.3, 5.1, 8.2, 51, 6.7, 0.0, 0.0], dtype=object)), 'The 1st row does not match! Make sure you loaded the right dataset!') ]) # TODO 1.2 feature_names = print(f'The feature names are:\n{feature_names.values}') todo_check([ (np.all(feature_names.values == np.array(['X', 'Y', 'month', 'day', 'FFMC', 'DMC', 'DC', 'ISI', 'temp', 'RH','wind', 'rain', 'area'],dtype='object')), "Wrong column names detected! Make sure you used .columns!") ])
TODO 1
Let's load the forestfires.csv by using Pandas read_csv() function. The read_csv() function works by taking in a path to a csv file (e.g., /home/user/Downloads/forestfires.csv could be a Linux/Mac file path; if you are using Windows, add r before your path). For simplicity, we are just going to pass the name of the csv as this assumes the forestfires.csv is in the same path as this notebook (i.e., your local path or current directory). Recall, we printed out the current directory and path of this notebook above. Make sure the forestfires.csv is in that directory.
-
Load Forest Fires dataset by passing the name of the csv file "forestfires.csv" to the Pandas function read_csv() (docs). Store the output into the forestfire_df variable.
- Note: we wrote a custom exception to alert you if you didn't move your csv file to the correct location. So if you are getting an error take a second to read what it says.
-
Using the forestfire_df DataFrame we just defined, call the columns class variable to store all the NAMES of the features in the dataset. Store the output into the variable feature_names.
- Note: recall that from last weeks lab, if you put a variable at the bottom of the cell Jupyter Notebook will automatically output what is contained in said variable to the notebook's output (i.e., the Out[] line).
# This line checks to make sure the forestfire.csv is in the
# same directory as this notebook.
if not os.path.exists("forestfires.csv"):
raise Exception(f"The forestfires.csv is not detected in your local path! " \
f"You need to move the 'forestfires.csv' file to the same " \
f"location/directory as this notebook which is {os.getcwd()}")
# TODO 1.1
display(forestfire_df)
todo_check([
(np.all(forestfire_df.iloc[0].values == np.array([7, 5, 'mar', 'fri', 86.2, 26.2, 94.3, 5.1, 8.2, 51, 6.7, 0.0, 0.0],
dtype=object)), 'The 1st row does not match! Make sure you loaded the right dataset!')
])
# TODO 1.2
feature_names =
print(f'The feature names are:\n{feature_names.values}')
todo_check([
(np.all(feature_names.values == np.array(['X', 'Y', 'month', 'day', 'FFMC', 'DMC', 'DC', 'ISI', 'temp', 'RH','wind', 'rain', 'area'],dtype='object')), "Wrong column names detected! Make sure you used .columns!")
])

Introduction
Pandas:
Pandas is just a Library for python to manipulating and analyzing the data. It provides tools for dealing with large datasets in addition to data structures for effectively storing huge databases. Series & DataFrame are indeed the two main computerized systems in Pandas.
A Series is a universally valid one-dimensional labelled array. It resembles a column in a worksheet or a table in a database.
Any data type can be placed in a DataFrame, that is a two-dimensional labelled data structure. It is comparable to a SQL table or a spreadsheet. A DataFrame can be thought of as a grouping of Series, where each Series represents a columns in the DataFrame.
DataFrames:
A DataFrame is a two-dimensional, size-mutable, and heterogeneous data structure in the Pandas library for Python. It is used to represent and manipulate tabular data, with labeled axes (rows and columns). The data in a DataFrame can be of any type, including integers, floating point numbers, strings, or other objects. The labeled axes enable easy access and manipulation of the data in the DataFrame, making it a popular choice for data analysis and manipulation in Python.
Trending now
This is a popular solution!
Step by step
Solved in 2 steps

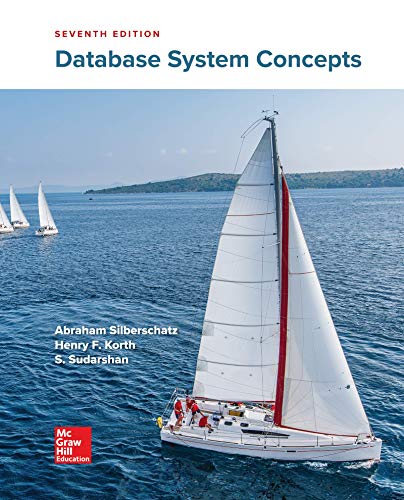
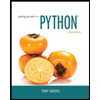
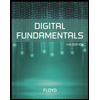
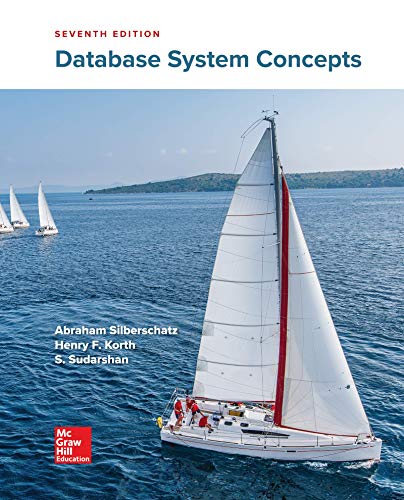
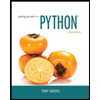
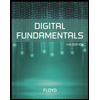
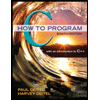
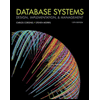
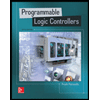