The station manager of a local television station is interested in predicting the amount of television (in hours) that a person in the viewing area will watch. The explanatory variables are age (in years), education (highest level obtained, in years) and family size (number of people in household). The multiple regression output is shown below: Summary measures Multiple R R-Square Adj R-Square 0.6644 StErr of Estimate 0.5598 ANOVA Table Source df SS MS F p-value Explained 3 13.9682 4.6561 0.0000 Unexplained 18 5.6413 0.3134 Regression coefficients Coefficient Std Err t-value p-value Constant 1.683 1.1696 1.4389 0.1674 Age -0.0498 0.0199 -2.5018 0.0222 Education 0.2135 0.0503 4.2426 0.0005 Family Size 0.0405 0.0784 0.5168 0.6116 Fill in the missing three numbers (in boxes shown above). Show calculations. Multiple R = R-Square = F =
Correlation
Correlation defines a relationship between two independent variables. It tells the degree to which variables move in relation to each other. When two sets of data are related to each other, there is a correlation between them.
Linear Correlation
A correlation is used to determine the relationships between numerical and categorical variables. In other words, it is an indicator of how things are connected to one another. The correlation analysis is the study of how variables are related.
Regression Analysis
Regression analysis is a statistical method in which it estimates the relationship between a dependent variable and one or more independent variable. In simple terms dependent variable is called as outcome variable and independent variable is called as predictors. Regression analysis is one of the methods to find the trends in data. The independent variable used in Regression analysis is named Predictor variable. It offers data of an associated dependent variable regarding a particular outcome.
- The station manager of a local television station is interested in predicting the amount of television (in hours) that a person in the viewing area will watch. The explanatory variables are age (in years), education (highest level obtained, in years) and family size (number of people in household). The multiple regression output is shown below:
Summary measures |
|
|
|
|
|||||||
Multiple R |
|
|
|
|
|
||||||
R-Square |
|
|
|
|
|
||||||
Adj R-Square |
0.6644 |
|
|
|
|
||||||
StErr of Estimate |
0.5598 |
|
|
|
|
||||||
|
|
|
|
|
|
||||||
ANOVA Table |
|
|
|
|
|
||||||
Source |
df |
SS |
MS |
F |
p-value |
||||||
Explained |
3 |
13.9682 |
4.6561 |
|
0.0000 |
||||||
Unexplained |
18 |
5.6413 |
0.3134 |
|
|
||||||
Regression coefficients |
|
|
|
|
|
||||||
|
Coefficient |
Std Err |
t-value |
p-value |
|
|
|||||
Constant |
1.683 |
1.1696 |
1.4389 |
0.1674 |
|
|
|||||
Age |
-0.0498 |
0.0199 |
-2.5018 |
0.0222 |
|
|
|||||
Education |
0.2135 |
0.0503 |
4.2426 |
0.0005 |
|
|
|||||
Family Size |
0.0405 |
0.0784 |
0.5168 |
0.6116 |
|
|
|||||
- Fill in the missing three numbers (in boxes shown above). Show calculations.
Multiple R =
R-Square =
F =

Trending now
This is a popular solution!
Step by step
Solved in 2 steps

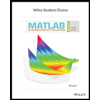
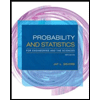
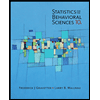
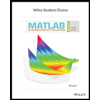
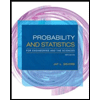
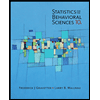
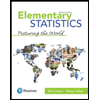
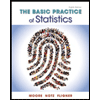
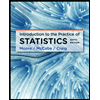