The first DataFrame must be called features, which is your feature matrix. The features DataFrame must contain the following feature columns in this exact order: days_cube, days_sq, days, temp, pop_dense_sq, pop_dense, pop. These features correspond to the features present in the equation above. ● days_cube and days_sq are the cubed and squared values of days, respectively. ● days is the number of days since the outbreak began in the respective capital city, which always begins with zero and adds 1 day for each row of recorded data for each capital city. There are 27 capital cities in Brazil. Each capital city has 151 days of recorded data. ● pop_dense_sq is the squared value of pop_dense ● pop is the city population ● Population density is defined as the population of a city divided by the area in kilometers squared of the city. The second DataFrame contains target values and must be called response, which are the accumulated case counts column for the capital cities. Your task is to construct these two Pandas DataFrames using the data provided.
The first DataFrame must be called features, which is your feature matrix. The features
DataFrame must contain the following feature columns in this exact order: days_cube,
days_sq, days, temp, pop_dense_sq, pop_dense, pop. These features correspond to
the features present in the equation above.
● days_cube and days_sq are the cubed and squared values of days, respectively.
● days is the number of days since the outbreak began in the respective capital city, which
always begins with zero and adds 1 day for each row of recorded data for each capital
city. There are 27 capital cities in Brazil. Each capital city has 151 days of recorded
data.
● pop_dense_sq is the squared value of pop_dense
● pop is the city population
● Population density is defined as the population of a city divided by the area in kilometers
squared of the city.
The second DataFrame contains target values and must be called response, which are the
accumulated case counts column for the capital cities. Your task is to construct these two Pandas
DataFrames using the data provided.


Trending now
This is a popular solution!
Step by step
Solved in 3 steps with 1 images

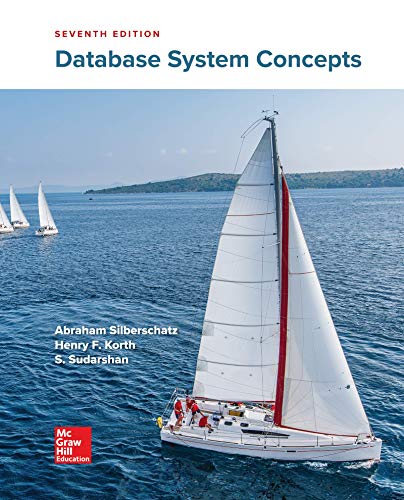
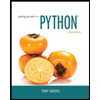
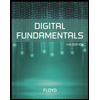
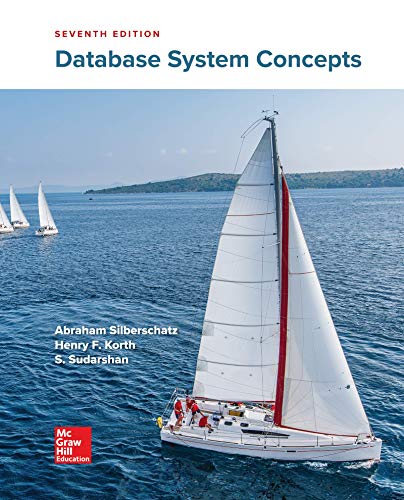
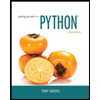
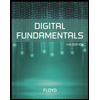
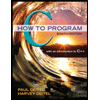
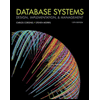
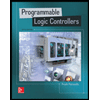