Suppose a researcher is trying to understand what makes living in a particular city desirable. She uses a list from a popular magazine of the current top 50 desirable cities to live in. Each city was given a desirability score by the magazine's viewers. The researcher is curious about how well she can predict those scores using only two independent variables: the number of miles to the closest airport and the median home price. She obtains the values for number of miles to the closest airport and median home price for each of the 50 cities and calculates the following values: Zero-Order Correlations: # of Miles to the Closest Airport and Median Home Price City Desirability Rating # of Miles to the Closest Airport Median Home Price City Desirability Rating 1.0001.000 −0.447−0.447 0.6040.604 # of Miles to the Closest Airport 1.0001.000 −0.066−0.066 Median Home Price 1.000 The researcher computes the partial correlation of 0.6440.644 between the city desirability rating and the median home price controlling for the number of miles to the closest airport. Because this value is not much different from the zero-order correlation between the city desirability rating and the ((A. # of miles to closest airport, B. median home price, C. city desirability rating)) , the researcher can consider the relationship between these two variables to be ((A. intervening, B. spurious, C. direct, D. interacting)), at least with respect to consideration of the number of miles to the closest airport. Using the provided correlations and the given partial correlation, the coefficient of multiple determination (R2R2) for the multiple regression equation predicting the city desirability rating from the other two variables is ((A. 0.730, B. 0.644, C. 0.532, D. 0.142)). The researcher can interpret this value to mean that ((A. 16%, B. 36.5%, C. 53.2%, D. 81%)) of the variance in the ((A. city diserability, B. median home price, C. # of miles to the closest airport)) is explained by ((A. median home price, B. # of miles to the closest airport, C. both # of miles to closest airport and the median home price, D. both the city desirability rating and the median home price, E. both the city desirability ragting and the # of miles to closest aiport)). . The independent variable number of miles to the closest airport predicts ((A. 21%, B. -44.7%, C. 20%, D. 60.4%)) of the variance in the dependent variable by itself. This suggests that also including median home price in the regression equation (( A. improves, B. does not improve)) how well the equation can predict the dependent variable. The multiple correlation coefficient (R) for the multiple regression equation predicting the city desirability rating from the other two variables is ((A. 0.532, B. 0.644, C. 0.142, D. 0.729))
Suppose a researcher is trying to understand what makes living in a particular city desirable. She uses a list from a popular magazine of the current top 50 desirable cities to live in. Each city was given a desirability score by the magazine's viewers. The researcher is curious about how well she can predict those scores using only two independent variables: the number of miles to the closest airport and the
Zero-Order
|
City Desirability Rating |
# of Miles to the Closest Airport |
Median Home Price |
---|---|---|---|
City Desirability Rating | 1.0001.000 | −0.447−0.447 | 0.6040.604 |
# of Miles to the Closest Airport | 1.0001.000 | −0.066−0.066 | |
Median Home Price |
1.000 |
The researcher computes the partial correlation of 0.6440.644 between the city desirability rating and the median home price controlling for the number of miles to the closest airport. Because this value is not much different from the
Using the provided correlations and the given partial correlation, the coefficient of multiple determination (R2R2) for the multiple regression equation predicting the city desirability rating from the other two variables is ((A. 0.730, B. 0.644, C. 0.532, D. 0.142)).
The researcher can interpret this value to mean that ((A. 16%, B. 36.5%, C. 53.2%, D. 81%)) of the variance in the ((A. city diserability, B. median home price, C. # of miles to the closest airport)) is explained by ((A. median home price, B. # of miles to the closest airport, C. both # of miles to closest airport and the median home price, D. both the city desirability rating and the median home price, E. both the city desirability ragting and the # of miles to closest aiport)). .
The independent variable number of miles to the closest airport predicts ((A. 21%, B. -44.7%, C. 20%, D. 60.4%)) of the variance in the dependent variable by itself. This suggests that also including median home price in the regression equation (( A. improves, B. does not improve)) how well the equation can predict the dependent variable.
The multiple

Trending now
This is a popular solution!
Step by step
Solved in 7 steps

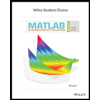
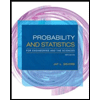
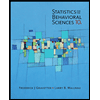
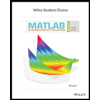
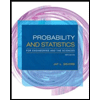
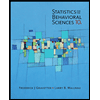
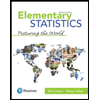
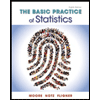
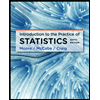