SUMMARY OUTPUT Regression Statistics Multiple 0.6918015 0.4785893 R Square Adjusted R Square 0.4311883 0.4960752 Standard Error 13 Observations ANOVA Significance F F MS SS df 10.096612 0.008800001 2.4846808 2.4846808 0.2460906 1 Regression 2.7069961 11 Residual 5.1916769 12 Total Upper 95.0% Lower 95.0% Upper 95% Standard Lower 95% P-value Error t Stat Coefficients -0.26559679 2.3211384 0.0019339 -0.265597 2.3211384 0.1081002 0.5876312 1.7490065 1.0277708 Intercept 0.0003511 0.0019339 0.0088 0.000351129 0.0003596 3.1775166 0.0011425 X Variable 1
Correlation
Correlation defines a relationship between two independent variables. It tells the degree to which variables move in relation to each other. When two sets of data are related to each other, there is a correlation between them.
Linear Correlation
A correlation is used to determine the relationships between numerical and categorical variables. In other words, it is an indicator of how things are connected to one another. The correlation analysis is the study of how variables are related.
Regression Analysis
Regression analysis is a statistical method in which it estimates the relationship between a dependent variable and one or more independent variable. In simple terms dependent variable is called as outcome variable and independent variable is called as predictors. Regression analysis is one of the methods to find the trends in data. The independent variable used in Regression analysis is named Predictor variable. It offers data of an associated dependent variable regarding a particular outcome.
Below is the output from regression. Use the p-value and decide if the equation is good to use for forecasting.


Trending now
This is a popular solution!
Step by step
Solved in 4 steps

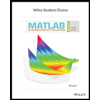
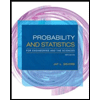
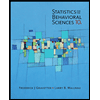
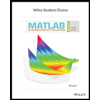
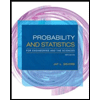
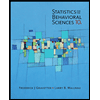
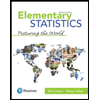
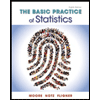
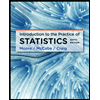