Summarize example 1.2 of the example applications of experimental design by determining the statement of the problem, objectives, choice of the
Summarize example 1.2 of the example applications of experimental design by determining the statement of the problem, objectives, choice of the
MATLAB: An Introduction with Applications
6th Edition
ISBN:9781119256830
Author:Amos Gilat
Publisher:Amos Gilat
Chapter1: Starting With Matlab
Section: Chapter Questions
Problem 1P
Related questions
Question
Summarize example 1.2 of the example applications of experimental design by determining the statement of the problem, objectives, choice of the response, variable, factors, design process.

Transcribed Image Text:EXAMPLE 1.2
Optimizing a Process
at 145°F and 2.1 hours of reaction time, producing yields
of around 80 percent. Figure 1.9 shows a view of the
time-temperature region from above. In this graph, the
lines of constant yield are connected to form response
contours, and we have shown the contour lines for yields
of 60, 70, 80, 90, and 95 percent. These contours are pro-
In a characterization experiment, we are usually interested
in determining which process variables affect the response.
A logical next step is to optimize, that is, to determine the
region in the important factors that leads to the best possi-
ble response. For example if the response is yield, we
would look for a region of maximum yield, whereas if the
response is variability in a critical product dimension, we jections on the time-temperature region of cross sections
would seek a region of minimum variability.
Suppose that we are interested in improving the yield
of a chemical process. We know from the results of a char-
acterization experiment that the two most important
process variables that influence the yield are operating
temperature and reaction time. The process currently runs
of the yield surface corresponding to the aforementioned
percent yields. This surface is sometimes called a
response surface. The true response surface in Figure 1.9
is unknown to the process personnel, so experimental
methods will be required to optimize the yield with
respect to time and temperature.
FIGURE 1.9 Contour plot of yield as a
function of reaction time and reaction temperature,
illustrating experimentation to optimize a process
Second optimization experiment
200
Path leading
to region of
higher yield
To locate the optimum, it is necessary to perform an
experiment that varies both time and temperature together,
that is, a factorial experiment. The results of an initial facto-
rial experiment with both time and temperature run at two
levels is shown in Figure 1.9. The responses observed at the
four corners of the square indicate that we should move in
the general direction of increased temperature and decreased
reaction time to increase yield. A few additional runs would
be performed in this direction, and this additional experimen-
tation would lead us to the region of maximum yield.
Once we have found the region of the optimum, a second
experiment would typically be performed. The objective of
this second experiment is to develop an empirical model of
the process and to obtain a more precise estimate of the opti-
mum operating conditions for time and temperature. This
approach to process optimization is called response surface
methodology, and it is explored in detail in Chapter 11. The
second design illustrated in Figure 1.9 is a central compos-
ite design, one of the most important experimental designs
used in process optimization studies.
190
180-
95%
170
160
90% Bo%
150
78
Initial
optimization
experiment
80
140 -
Current
operating
conditions
70
75
60%
0.5
1.0
1.5
2.0
2.5
Time (hours)
Temperature ("F)
Expert Solution

This question has been solved!
Explore an expertly crafted, step-by-step solution for a thorough understanding of key concepts.
Step by step
Solved in 2 steps

Recommended textbooks for you
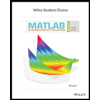
MATLAB: An Introduction with Applications
Statistics
ISBN:
9781119256830
Author:
Amos Gilat
Publisher:
John Wiley & Sons Inc
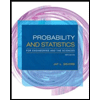
Probability and Statistics for Engineering and th…
Statistics
ISBN:
9781305251809
Author:
Jay L. Devore
Publisher:
Cengage Learning
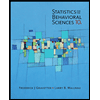
Statistics for The Behavioral Sciences (MindTap C…
Statistics
ISBN:
9781305504912
Author:
Frederick J Gravetter, Larry B. Wallnau
Publisher:
Cengage Learning
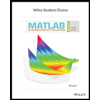
MATLAB: An Introduction with Applications
Statistics
ISBN:
9781119256830
Author:
Amos Gilat
Publisher:
John Wiley & Sons Inc
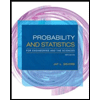
Probability and Statistics for Engineering and th…
Statistics
ISBN:
9781305251809
Author:
Jay L. Devore
Publisher:
Cengage Learning
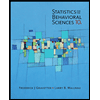
Statistics for The Behavioral Sciences (MindTap C…
Statistics
ISBN:
9781305504912
Author:
Frederick J Gravetter, Larry B. Wallnau
Publisher:
Cengage Learning
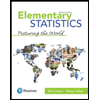
Elementary Statistics: Picturing the World (7th E…
Statistics
ISBN:
9780134683416
Author:
Ron Larson, Betsy Farber
Publisher:
PEARSON
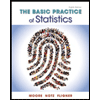
The Basic Practice of Statistics
Statistics
ISBN:
9781319042578
Author:
David S. Moore, William I. Notz, Michael A. Fligner
Publisher:
W. H. Freeman
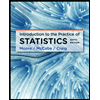
Introduction to the Practice of Statistics
Statistics
ISBN:
9781319013387
Author:
David S. Moore, George P. McCabe, Bruce A. Craig
Publisher:
W. H. Freeman