Activity 1: Year 2 Forecast Forecast next period Average Actual Demand Year 2 MAPE% MAPE Month Year 1 Year 2 Seasonal Index JAN JAN 80 0.954 FEB FEB 86 0.848 MAR = MAR 80 0.901 APR APR 111 1.033 MAY ÷ MAY 116 1.298 JUN JUN 122 1.219 JUL = JUL 101 1.139 AUG AUG 122 1.113 SEPT SEPT 86 1.033 OCT OCT 76 0.848 NOV 86 0.848 NOV = DEC DEC 76 0.768 Activity 2: Forecast Technique Analysis Forecasting technique that best fits the data: Seasonal Forecast ^ Scenario You have been given a task to create a demand forecast for the second year of sales of a premium outdoor grill. Accurate forecasts are important for many reasons, including for the company to ensure they have the materials they need to create the products required in a certain period of time. Your objective is to minimize the forecast error, which will be measured using the Mean Absolute Percentage Error (MAPE) with a goal of being below 25%. You have historical monthly sales data for the past year and access to software that provides forecasts based on five different forecasting techniques (Naïve, 3-Month Moving Average, Exponential Smoothing for .2, Exponential Smooth for .5, and Seasonal) to help determine the best forecast for that particular month. Based on the given data, you will identify trends and patterns to create a more accurate forecast. Approach Consider the previous month's forecast to identify which technique is most effective. Use that to forecast the next month. Remember to select the forecasting technique that produces the forecast error nearest to zero. For example: a. Naïve Forecast is 230 and the Forecast Error is -15. b. 3-Month Moving Forecast is 290 and the Forecast Error is -75. c. Exponential Smoothing Forecast for .2 is 308 and the Forecast Error is -93. d. Exponential Smoothing Forecast for .5 is 279 and the Forecast Error is -64. e. Seasonal Forecast is 297 and the Forecast Error is -82. The forecast for the next month would be 230 as the Naïve Forecast had the Forecast Error closest to zero with a -15. This forecasting technique was the best performing technique for that month. You do not need to do any external analysis-the forecast error for each strategy is already calculated for you in the tables below. Naïve Month Period Actual Demand Naïve Forecast Error 3- Month Moving Forecast 3- Month Moving Forecast Error Exponential Exponential Exponential Exponential Smoothing Smoothing Smoothing Smoothing Forecast for .2 Forecast Forecast for 5 Forecast Seasonal Seasonal Forecast Error .2 Error .5 Error ▾ Year 1 JAN 1 80 70 10 74 6 73 7 84 -4 FEB 2 86 80 6 75 11 77 9 101 -15 MAR 3 80 86 -6 79 1 77 3 82 -2 89 -9 APR 4 111 80 31 82 29 78 MAY 5 116 111 5 92 24 85 JUN 6 122 116 6 102 20 JUL 7 101 122 -21 116 -15 AUG ∞ 8 122 101 21 113 9 SEPT 9 86 122 -36 115 -29 103 OCT 10 76 86 -10 103 -27 NOV 11 86 76 10 95 -9 DEC 12 76 86 -10 83 -7 Ç ྨ 。 。 སྒ ཋ ཋ ྴ| 33 81 30 107 4 31 96 20 89 27 91 31 106 16 100 22 97 4 114 -13 89 12 98 24 108 14 110 12 -17 115 -29 83 3 100 -24 101 -25 90 -14 95 -9 89 -3 101 -15 93 -17 88 -12 99 -23 Year 2 JAN 13 76 FEB 14 MAR 15 APR 16 MAY 17 JUN 18 JUL 19 AUG 20 SEPT 21 OCT 22 NOV 23 DEC 24
Activity 1: Year 2 Forecast Forecast next period Average Actual Demand Year 2 MAPE% MAPE Month Year 1 Year 2 Seasonal Index JAN JAN 80 0.954 FEB FEB 86 0.848 MAR = MAR 80 0.901 APR APR 111 1.033 MAY ÷ MAY 116 1.298 JUN JUN 122 1.219 JUL = JUL 101 1.139 AUG AUG 122 1.113 SEPT SEPT 86 1.033 OCT OCT 76 0.848 NOV 86 0.848 NOV = DEC DEC 76 0.768 Activity 2: Forecast Technique Analysis Forecasting technique that best fits the data: Seasonal Forecast ^ Scenario You have been given a task to create a demand forecast for the second year of sales of a premium outdoor grill. Accurate forecasts are important for many reasons, including for the company to ensure they have the materials they need to create the products required in a certain period of time. Your objective is to minimize the forecast error, which will be measured using the Mean Absolute Percentage Error (MAPE) with a goal of being below 25%. You have historical monthly sales data for the past year and access to software that provides forecasts based on five different forecasting techniques (Naïve, 3-Month Moving Average, Exponential Smoothing for .2, Exponential Smooth for .5, and Seasonal) to help determine the best forecast for that particular month. Based on the given data, you will identify trends and patterns to create a more accurate forecast. Approach Consider the previous month's forecast to identify which technique is most effective. Use that to forecast the next month. Remember to select the forecasting technique that produces the forecast error nearest to zero. For example: a. Naïve Forecast is 230 and the Forecast Error is -15. b. 3-Month Moving Forecast is 290 and the Forecast Error is -75. c. Exponential Smoothing Forecast for .2 is 308 and the Forecast Error is -93. d. Exponential Smoothing Forecast for .5 is 279 and the Forecast Error is -64. e. Seasonal Forecast is 297 and the Forecast Error is -82. The forecast for the next month would be 230 as the Naïve Forecast had the Forecast Error closest to zero with a -15. This forecasting technique was the best performing technique for that month. You do not need to do any external analysis-the forecast error for each strategy is already calculated for you in the tables below. Naïve Month Period Actual Demand Naïve Forecast Error 3- Month Moving Forecast 3- Month Moving Forecast Error Exponential Exponential Exponential Exponential Smoothing Smoothing Smoothing Smoothing Forecast for .2 Forecast Forecast for 5 Forecast Seasonal Seasonal Forecast Error .2 Error .5 Error ▾ Year 1 JAN 1 80 70 10 74 6 73 7 84 -4 FEB 2 86 80 6 75 11 77 9 101 -15 MAR 3 80 86 -6 79 1 77 3 82 -2 89 -9 APR 4 111 80 31 82 29 78 MAY 5 116 111 5 92 24 85 JUN 6 122 116 6 102 20 JUL 7 101 122 -21 116 -15 AUG ∞ 8 122 101 21 113 9 SEPT 9 86 122 -36 115 -29 103 OCT 10 76 86 -10 103 -27 NOV 11 86 76 10 95 -9 DEC 12 76 86 -10 83 -7 Ç ྨ 。 。 སྒ ཋ ཋ ྴ| 33 81 30 107 4 31 96 20 89 27 91 31 106 16 100 22 97 4 114 -13 89 12 98 24 108 14 110 12 -17 115 -29 83 3 100 -24 101 -25 90 -14 95 -9 89 -3 101 -15 93 -17 88 -12 99 -23 Year 2 JAN 13 76 FEB 14 MAR 15 APR 16 MAY 17 JUN 18 JUL 19 AUG 20 SEPT 21 OCT 22 NOV 23 DEC 24
Chapter6: Target Markets: Segmentation And Evaluation
Section: Chapter Questions
Problem 17DRQ: Under what conditions might a firm use multiple forecasting methods?
Related questions
Question

Transcribed Image Text:Activity 1: Year 2 Forecast
Forecast next
period
Average
Actual Demand
Year 2
MAPE%
MAPE
Month
Year 1
Year 2
Seasonal Index
JAN
JAN
80
0.954
FEB
FEB
86
0.848
MAR
=
MAR
80
0.901
APR
APR
111
1.033
MAY
÷
MAY
116
1.298
JUN
JUN
122
1.219
JUL
=
JUL
101
1.139
AUG
AUG
122
1.113
SEPT
SEPT
86
1.033
OCT
OCT
76
0.848
NOV
86
0.848
NOV
=
DEC
DEC
76
0.768
Activity 2: Forecast Technique Analysis
Forecasting technique that best fits the data: Seasonal Forecast
^

Transcribed Image Text:Scenario
You have been given a task to create a demand forecast for the second year of sales of a premium outdoor grill.
Accurate forecasts are important for many reasons, including for the company to ensure they have the materials
they need to create the products required in a certain period of time. Your objective is to minimize the forecast
error, which will be measured using the Mean Absolute Percentage Error (MAPE) with a goal of being below 25%.
You have historical monthly sales data for the past year and access to software that provides forecasts based on
five different forecasting techniques (Naïve, 3-Month Moving Average, Exponential Smoothing for .2, Exponential
Smooth for .5, and Seasonal) to help determine the best forecast for that particular month. Based on the given
data, you will identify trends and patterns to create a more accurate forecast.
Approach
Consider the previous month's forecast to identify which technique is most effective. Use that to forecast the next
month.
Remember to select the forecasting technique that produces the forecast error nearest to zero. For example:
a. Naïve Forecast is 230 and the Forecast Error is -15.
b. 3-Month Moving Forecast is 290 and the Forecast Error is -75.
c. Exponential Smoothing Forecast for .2 is 308 and the Forecast Error is -93.
d. Exponential Smoothing Forecast for .5 is 279 and the Forecast Error is -64.
e. Seasonal Forecast is 297 and the Forecast Error is -82.
The forecast for the next month would be 230 as the Naïve Forecast had the Forecast Error closest to zero with a
-15. This forecasting technique was the best performing technique for that month. You do not need to do any
external analysis-the forecast error for each strategy is already calculated for you in the tables below.
Naïve
Month Period
Actual
Demand
Naïve Forecast
Error
3-
Month
Moving
Forecast
3-
Month
Moving
Forecast
Error
Exponential Exponential Exponential Exponential
Smoothing Smoothing Smoothing Smoothing
Forecast for .2 Forecast Forecast for 5 Forecast
Seasonal
Seasonal Forecast
Error
.2
Error
.5
Error
▾ Year 1
JAN
1
80
70
10
74
6
73
7
84
-4
FEB
2
86
80
6
75
11
77
9
101
-15
MAR
3
80
86
-6
79
1
77
3
82
-2
89
-9
APR
4
111
80
31
82
29
78
MAY
5
116
111
5
92
24
85
JUN
6
122
116
6
102
20
JUL
7
101 122
-21
116
-15
AUG
∞
8
122
101
21
113
9
SEPT
9
86
122
-36
115
-29
103
OCT 10
76
86
-10
103
-27
NOV 11
86
76
10
95
-9
DEC 12
76
86
-10
83
-7
Ç ྨ 。 。 སྒ ཋ ཋ ྴ|
33
81
30
107
4
31
96
20
89
27
91
31
106
16
100
22
97
4
114
-13
89
12
98
24
108
14
110
12
-17
115
-29
83
3
100
-24
101
-25
90
-14
95
-9
89
-3
101
-15
93
-17
88
-12
99
-23
Year 2
JAN
13
76
FEB
14
MAR 15
APR 16
MAY 17
JUN 18
JUL 19
AUG 20
SEPT 21
OCT 22
NOV 23
DEC
24
Expert Solution

This question has been solved!
Explore an expertly crafted, step-by-step solution for a thorough understanding of key concepts.
Step by step
Solved in 2 steps with 3 images

Recommended textbooks for you
Marketing
Marketing
ISBN:
9780357033791
Author:
Pride, William M
Publisher:
South Western Educational Publishing
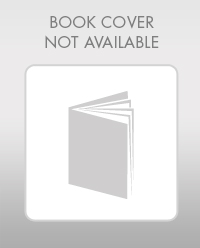
Contemporary Marketing
Marketing
ISBN:
9780357033777
Author:
Louis E. Boone, David L. Kurtz
Publisher:
Cengage Learning
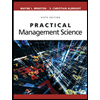
Practical Management Science
Operations Management
ISBN:
9781337406659
Author:
WINSTON, Wayne L.
Publisher:
Cengage,
Marketing
Marketing
ISBN:
9780357033791
Author:
Pride, William M
Publisher:
South Western Educational Publishing
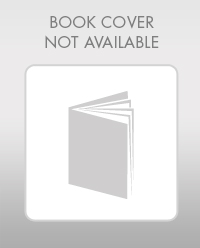
Contemporary Marketing
Marketing
ISBN:
9780357033777
Author:
Louis E. Boone, David L. Kurtz
Publisher:
Cengage Learning
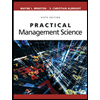
Practical Management Science
Operations Management
ISBN:
9781337406659
Author:
WINSTON, Wayne L.
Publisher:
Cengage,