Problem 9: Capacity of Recurrent Neural Networks (RNNs) for Sequence Modeling Statement: Prove that recurrent neural networks (RNNs) with a certain architecture and activation functions can represent any computable sequence function. Specifically, show that RNNs are Turing complete under appropriate conditions. Key Points for the Proof: • Define the computational capabilities of RNNs and relate them to models of computation like Turing machines. Construct an RNN architecture that can simulate the operations of a Turing machine. • Address the role of activation functions and network depth in achieving computational universality. Provide a formal argument demonstrating the equivalence of RNNs to Turing-complete systems.
Problem 9: Capacity of Recurrent Neural Networks (RNNs) for Sequence Modeling Statement: Prove that recurrent neural networks (RNNs) with a certain architecture and activation functions can represent any computable sequence function. Specifically, show that RNNs are Turing complete under appropriate conditions. Key Points for the Proof: • Define the computational capabilities of RNNs and relate them to models of computation like Turing machines. Construct an RNN architecture that can simulate the operations of a Turing machine. • Address the role of activation functions and network depth in achieving computational universality. Provide a formal argument demonstrating the equivalence of RNNs to Turing-complete systems.
Linear Algebra: A Modern Introduction
4th Edition
ISBN:9781285463247
Author:David Poole
Publisher:David Poole
Chapter5: Orthogonality
Section5.3: The Gram-schmidt Process And The Qr Factorization
Problem 7BEXP
Related questions
Question

Transcribed Image Text:Problem 9: Capacity of Recurrent Neural Networks (RNNs) for Sequence
Modeling
Statement: Prove that recurrent neural networks (RNNs) with a certain architecture and activation
functions can represent any computable sequence function. Specifically, show that RNNs are Turing
complete under appropriate conditions.
Key Points for the Proof:
•
Define the computational capabilities of RNNs and relate them to models of computation like
Turing machines.
Construct an RNN architecture that can simulate the operations of a Turing machine.
• Address the role of activation functions and network depth in achieving computational
universality.
Provide a formal argument demonstrating the equivalence of RNNs to Turing-complete
systems.
Expert Solution

This question has been solved!
Explore an expertly crafted, step-by-step solution for a thorough understanding of key concepts.
Step by step
Solved in 2 steps

Recommended textbooks for you
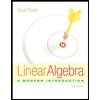
Linear Algebra: A Modern Introduction
Algebra
ISBN:
9781285463247
Author:
David Poole
Publisher:
Cengage Learning
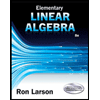
Elementary Linear Algebra (MindTap Course List)
Algebra
ISBN:
9781305658004
Author:
Ron Larson
Publisher:
Cengage Learning
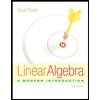
Linear Algebra: A Modern Introduction
Algebra
ISBN:
9781285463247
Author:
David Poole
Publisher:
Cengage Learning
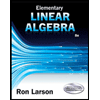
Elementary Linear Algebra (MindTap Course List)
Algebra
ISBN:
9781305658004
Author:
Ron Larson
Publisher:
Cengage Learning