Peter has data on animal weights (lb) and speed (km/h). 1. Write some noticeable facts in the data. Does linear model is fit it? (see Picture 1) 2. What do you learn from residuals? Now, does linear model fit the data? (see Picture 2) 3. Suppose linear regression model fit the data. Here is a model. Based on it, what is the relationship of weight and speed? Does it significant on statistic? ## lm(formula = speed ~ weight, data = Q4) ## ## Residuals: ## Min 1Q Median 3Q Max ## -3.3489 -0.4803 0.0133 0.3890 7.5201 ## ## Coefficients: ## Estimate Std. Error t value Pr(>|t|) ## (Intercept) 1.90798 0.09462 20.16 <2e-16 *** ## weight 0.11182 0.00324 34.51 <2e-16 *** ## --- ## Signif. codes: 0 '***' 0.001 '**' 0.01 '*' 0.05 '.' 0.1 ' ' 1 ## ## Residual standard error: 0.7742 on 299 degrees of freedom ## Multiple R-squared: 0.7993, Adjusted R-squared: 0.7987 ## F-statistic: 1191 on 1 and 299 DF, p-value: < 2.2e-16
Correlation
Correlation defines a relationship between two independent variables. It tells the degree to which variables move in relation to each other. When two sets of data are related to each other, there is a correlation between them.
Linear Correlation
A correlation is used to determine the relationships between numerical and categorical variables. In other words, it is an indicator of how things are connected to one another. The correlation analysis is the study of how variables are related.
Regression Analysis
Regression analysis is a statistical method in which it estimates the relationship between a dependent variable and one or more independent variable. In simple terms dependent variable is called as outcome variable and independent variable is called as predictors. Regression analysis is one of the methods to find the trends in data. The independent variable used in Regression analysis is named Predictor variable. It offers data of an associated dependent variable regarding a particular outcome.
Peter has data on animal weights (lb) and speed (km/h).
1. Write some noticeable facts in the data. Does linear model is fit it?
(see Picture 1)
2. What do you learn from residuals? Now, does linear model fit the data?
(see Picture 2)
3. Suppose linear regression model fit the data. Here is a model. Based on it, what is the relationship of weight and speed? Does it significant on statistic?
## lm(formula = speed ~ weight, data = Q4)
##
## Residuals:
## Min 1Q
## -3.3489 -0.4803 0.0133 0.3890 7.5201
##
## Coefficients:
## Estimate Std. Error t value Pr(>|t|)
## (Intercept) 1.90798 0.09462 20.16 <2e-16 ***
## weight 0.11182 0.00324 34.51 <2e-16 ***
## ---
## Signif. codes: 0 '***' 0.001 '**' 0.01 '*' 0.05 '.' 0.1 ' ' 1
##
## Residual standard error: 0.7742 on 299 degrees of freedom
## Multiple R-squared: 0.7993, Adjusted R-squared: 0.7987
## F-statistic: 1191 on 1 and 299 DF, p-value: < 2.2e-16



Step by step
Solved in 3 steps

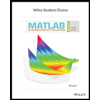
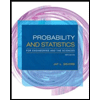
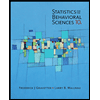
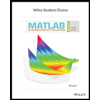
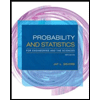
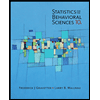
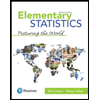
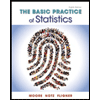
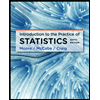