ODO 6 Slice the 'area' column using either iloc, loc or square brackets [ ]. Store the output into the variable area_values. Plot area_values using the Matplotlib plt.hist() function. For the plt.hist() function all we have to do is pass area_values and it will automatically compute the bins our data (bins help to discretize continuous data by breaking the continuous data into discrete groups). Optionally, we could specify the bins. See the docs for more information. Call and print the value_counts() method from area_values to see how many data samples have values near 0. Store the output into the variable area_value_count. # TODO 6.1 area_values = display(area_values) todo_check([ (area_values.shape == (517,), 'area_values shape did not match (517,)'), (np.all(area_values.values[-10:] == np.array([ 0. , 0. , 2.17, 0.43, 0. , 6.44, 54.29, 11.16, 0. ,0. ])), 'area_values did not contain the correct values!') ]) # TODO 6.2 plt.show() # TODO 6.3 area_value_count = display(area_value_count) todo_check([ (area_value_count.shape == (251,), 'area_value_count shape did not match (251,)'), (np.all(area_value_count.values[:5] == np.array([247, 3, 2, 2, 2])), 'area_value_count values did not match!') ])
TODO 6
-
Slice the 'area' column using either iloc, loc or square brackets [ ]. Store the output into the variable area_values.
-
Plot area_values using the Matplotlib plt.hist() function. For the plt.hist() function all we have to do is pass area_values and it will automatically compute the bins our data (bins help to discretize continuous data by breaking the continuous data into discrete groups). Optionally, we could specify the bins. See the docs for more information.
-
Call and print the value_counts() method from area_values to see how many data samples have values near 0. Store the output into the variable area_value_count.
# TODO 6.1
area_values =
display(area_values)
todo_check([
(area_values.shape == (517,), 'area_values shape did not match (517,)'),
(np.all(area_values.values[-10:] == np.array([ 0. , 0. , 2.17, 0.43, 0. , 6.44, 54.29, 11.16, 0. ,0. ])), 'area_values did not contain the correct values!')
])
# TODO 6.2
plt.show()
# TODO 6.3
area_value_count =
display(area_value_count)
todo_check([
(area_value_count.shape == (251,), 'area_value_count shape did not match (251,)'),
(np.all(area_value_count.values[:5] == np.array([247, 3, 2, 2, 2])), 'area_value_count values did not match!')
])

TODO 6 is a section in code that is responsible for creating a histogram of the "area" column of a DataFrame and counting the number of occurrences of each value in the "area" column.
The first step of TODO 6 is to slice the 'area' column from the DataFrame and store it into the variable "area_values".
The next step is to plot the histogram of the "area_values". This can be done using the Matplotlib plt.hist() function, where "area_values" is passed as the argument to the function.
The final step is to count the number of occurrences of each value in the "area_values" and store the result in the variable "area_value_count". This can be done using the value_counts() method from the "area_values" variable.
The output of the code should be:
- A histogram of the "area_values"
- A table showing the count of occurrences of each value in the "area_values".
Todo_check() is a function that checks if the expected outcome has been met, it will display an error message if the outcome does not match the expected result.
Trending now
This is a popular solution!
Step by step
Solved in 4 steps with 1 images

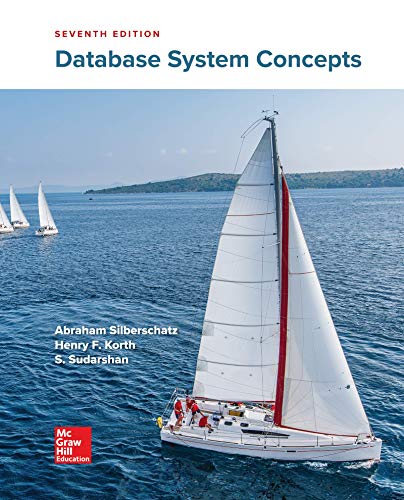
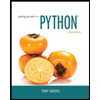
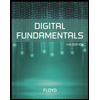
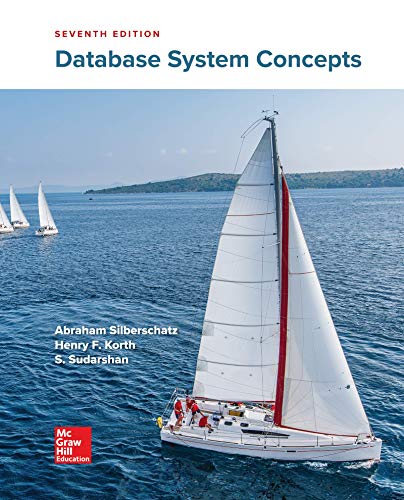
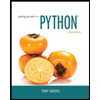
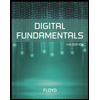
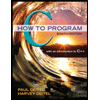
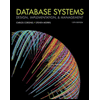
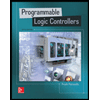