log likelihood. Concretely, assume a classification problem with c classes • Samples are (x(1¹), y(1)),..., (x(m), y(m)), wherex(1) = R¹, y(i) € {1,...,c}, j = 1,...,m • Parameters are 0 = {w₁b₁}=1... • Probablistic model is where Pr (3G) = i | xG),0) = softmax; (x(j)) softmax, (x) = ew/x+b; additional dimension of constant 1. Let x = N This unifies V Land V, L into V- L. b₂ W C k=1 Derive the log-likelihood L, and its gradient w.r.t. the parameters, VL and V₁L, for i = 1,..., c. ex+b Note: We can group w, and b, into a single vector by augmenting the data vectors with an W = - [.]. then a₂(x) = w/x+b¡ = wȚx.
log likelihood. Concretely, assume a classification problem with c classes • Samples are (x(1¹), y(1)),..., (x(m), y(m)), wherex(1) = R¹, y(i) € {1,...,c}, j = 1,...,m • Parameters are 0 = {w₁b₁}=1... • Probablistic model is where Pr (3G) = i | xG),0) = softmax; (x(j)) softmax, (x) = ew/x+b; additional dimension of constant 1. Let x = N This unifies V Land V, L into V- L. b₂ W C k=1 Derive the log-likelihood L, and its gradient w.r.t. the parameters, VL and V₁L, for i = 1,..., c. ex+b Note: We can group w, and b, into a single vector by augmenting the data vectors with an W = - [.]. then a₂(x) = w/x+b¡ = wȚx.
Advanced Engineering Mathematics
10th Edition
ISBN:9780470458365
Author:Erwin Kreyszig
Publisher:Erwin Kreyszig
Chapter2: Second-order Linear Odes
Section: Chapter Questions
Problem 1RQ
Related questions
Question
![2
Softmax classifier gradient. For softmax classifier, derive the gradient of the
log likelihood.
Concretely, assume a classification problem with c classes
• Samples are (x(1), y(1)),..., (x(m), y(m)), where x(1) Rn, y) = {1,...,c}, j = 1,...,m
• Parameters are 0 = {w₁b₁}=1,...c
. Probablistic model is
where
Pr
r(yG) = i | xG), 0) = softmax; (x(j))
softmax, (x) =
Σk=1
Derive the log-likelihood £, and its gradient w.r.t. the parameters, VwL and V₁L, for
i = 1,..., c.
W
Note: We can group w, and b, into a single vector by augmenting the data vectors with an
additional dimension of constant 1. Let x
wx.
=
1₁
[M]. then a, (x) = w/x+b₁ = '
ew/x+b;
ew/x+bk
This unifies VL and V, L into VL.
W₂
W₁](/v2/_next/image?url=https%3A%2F%2Fcontent.bartleby.com%2Fqna-images%2Fquestion%2Fb7b26484-772b-4284-af5a-fa2f3fa080bf%2F3ef6ef3a-8ef8-47da-8339-b9be7bf9a633%2Fvwu8037_processed.png&w=3840&q=75)
Transcribed Image Text:2
Softmax classifier gradient. For softmax classifier, derive the gradient of the
log likelihood.
Concretely, assume a classification problem with c classes
• Samples are (x(1), y(1)),..., (x(m), y(m)), where x(1) Rn, y) = {1,...,c}, j = 1,...,m
• Parameters are 0 = {w₁b₁}=1,...c
. Probablistic model is
where
Pr
r(yG) = i | xG), 0) = softmax; (x(j))
softmax, (x) =
Σk=1
Derive the log-likelihood £, and its gradient w.r.t. the parameters, VwL and V₁L, for
i = 1,..., c.
W
Note: We can group w, and b, into a single vector by augmenting the data vectors with an
additional dimension of constant 1. Let x
wx.
=
1₁
[M]. then a, (x) = w/x+b₁ = '
ew/x+b;
ew/x+bk
This unifies VL and V, L into VL.
W₂
W₁
Expert Solution

This question has been solved!
Explore an expertly crafted, step-by-step solution for a thorough understanding of key concepts.
Step by step
Solved in 2 steps with 1 images

Similar questions
Recommended textbooks for you
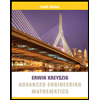
Advanced Engineering Mathematics
Advanced Math
ISBN:
9780470458365
Author:
Erwin Kreyszig
Publisher:
Wiley, John & Sons, Incorporated
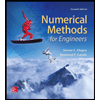
Numerical Methods for Engineers
Advanced Math
ISBN:
9780073397924
Author:
Steven C. Chapra Dr., Raymond P. Canale
Publisher:
McGraw-Hill Education
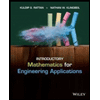
Introductory Mathematics for Engineering Applicat…
Advanced Math
ISBN:
9781118141809
Author:
Nathan Klingbeil
Publisher:
WILEY
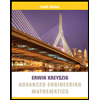
Advanced Engineering Mathematics
Advanced Math
ISBN:
9780470458365
Author:
Erwin Kreyszig
Publisher:
Wiley, John & Sons, Incorporated
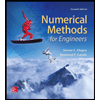
Numerical Methods for Engineers
Advanced Math
ISBN:
9780073397924
Author:
Steven C. Chapra Dr., Raymond P. Canale
Publisher:
McGraw-Hill Education
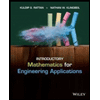
Introductory Mathematics for Engineering Applicat…
Advanced Math
ISBN:
9781118141809
Author:
Nathan Klingbeil
Publisher:
WILEY
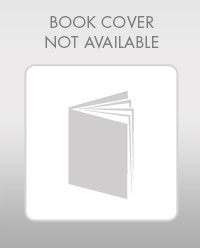
Mathematics For Machine Technology
Advanced Math
ISBN:
9781337798310
Author:
Peterson, John.
Publisher:
Cengage Learning,
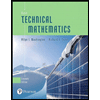
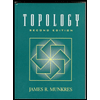