Let arr86 be a binary variable equal to 1 if a man was arrested during 1986, and zero otherwise. You have a dataset of young men from California who were born in 1960 or 1961 and have at least one arrest prior to 1986. You also have data on the proportion of prior arrests that led to a conviction (pcnv), the average sentence served from prior convictions in months (avgsen), months spent in prison since age 18 prior to 1986 (tottime), months spent in prison in 1986 (ptime86), and the number of quarters (0 to 4) that the man was legally employed in 1986 (qemp86). You estimate the following linear probability model (standard errors are in parentheses): arr86 = 0.441 - .162pcnv + .0061avgsen - .0023tottime (.017) (.021) (.0065) (.005) - .022ptime86 - .043qemp86 (.005) (.005) n= 2725 R2=.0474 Intercept the coefficient on avgsen, commenting on both magnitude and significance. Based on these results, do longer sentences deter future crime?
Let arr86 be a binary variable equal to 1 if a man was arrested during 1986, and zero
otherwise. You have a dataset of young men from California who were born in 1960 or
1961 and have at least one arrest prior to 1986. You also have data on the proportion of
prior arrests that led to a conviction (pcnv), the average sentence served from prior
convictions in months (avgsen), months spent in prison since age 18 prior to 1986
(tottime), months spent in prison in 1986 (ptime86), and the number of quarters (0 to 4)
that the man was legally employed in 1986 (qemp86). You estimate the following linear
probability model (standard errors are in parentheses):
arr86 = 0.441 - .162pcnv + .0061avgsen - .0023tottime
(.017) (.021) (.0065) (.005)
- .022ptime86 - .043qemp86
(.005) (.005)
n= 2725
R2=.0474
Intercept the coefficient on avgsen, commenting on both magnitude and significance. Based on these results, do longer sentences deter future crime?

Trending now
This is a popular solution!
Step by step
Solved in 2 steps

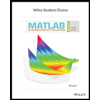
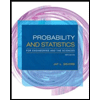
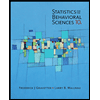
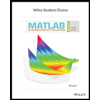
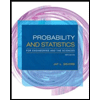
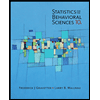
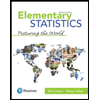
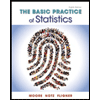
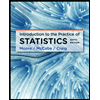